A Brief Chat About Data Labeling in Machine Learning and AI [+ Future Expectations]
![A Brief Chat About Data Labeling in Machine Learning and AI [+ Future Expectations]](/content/images/size/w1200/2023/03/labeling.jpg)
Let’s put things very simply. Much of machine learning revolves around something humans do automatically. You're simultaneously perceiving and classifying when you arrive at a red light. It’s the same when you pick up an apple at the grocery store and scan it carefully for defects. Looking out of an airplane window, you label the sections of ground in your mind’s eye.
You might not have known this, but you’re a data labeling expert.
Data labeling is an essential tool that machines use to learn. If our programs can label data autonomously, humans benefit greatly.
The process starts with manual labeling, done by human annotators. Before we can allow autonomous programs to drive our cars, there’s work to do. Manual labeling lays the groundwork. We need human-labeled data from real driving scenarios to feed our autonomous driving programs.
The labels we give the software are essentially rules. When the reference data is strong, our machine-learning software operates better. This balance works the other way too. Corrupted data and errors erode quality. If your training software has flaws, can you rely on the software it teaches? Low-quality data can seriously affect the outcome and profitability of annotation projects.
Trust is vital between humans. The idea of letting AI make its own decisions can be unsettling to some. For the foreseeable future, society will have extremely high demands for AI’s accuracy.
We need this autonomous software to draw conclusions and extrapolate. Before that, we need to use trusted data labeling techniques. When we think about trusting autonomous programs, self-driving vehicles are the first industry that comes to mind. Aerial labeling of crops and agricultural AI will help us meet global food demands, but only if we can trust its predictions.
The Three Main Methods of Machine Learning
There are varying degrees of machine learning automation. There’s still a significant human element to two of these primary categories.
Supervised Machine Learning
Once a labeled dataset is fed to a machine learning program, some adjustments can be made. The program gets monitored by human annotators for ongoing accuracy and compared to benchmark data. Once everything is properly synced and labeled accurately, the full potential can be tapped into. These automated programs can make predictions and classify data.
Semi-Supervised Machine Learning
Semi-supervised learning is useful when companies need to label a large volume of data. With massive amounts of data, it’s not always possible to annotate everything with a supervised approach. For example, self-driving technology has to be built upon a huge dataset. For situations like that, it’s best to automate a portion of the annotation and labeling.
Unsupervised Machine Learning
That’s right, unsupervised learning. There’s no human intervention for this category of machine learning method. Instead, algorithms give the programs actionable parameters to approach unlabeled datasets. This can be a viable strategy for discovering hidden trends within customer data. Unsupervised machine learning programs can also find new sales strategies, plus other ways to optimize business operations.
The Relationship Between Data Labeling Tools and ML
These two have a very close relationship; one can’t exist without the other. Data labeling trains machine learning, teaching software how to classify what it sees. The annotated data acts as a set of rules for these automated programs.
After the AI model learns the training rules and performs with accuracy, we reap the benefits. We can use these machine learning programs to predict all sorts of things. The predictions that Netflix makes are one of the keys to its success. Your movie and TV show viewing habits are autonomously labeled, and your recommendation list is built.
Manual Labeling vs. Automated Labeling Tools
Some data annotation tools can be automated, but what's the catch? Depending on the data you use the labeling tool for, you might need to validate the results. The more niche and specific the data is, the tougher it might be to label autonomously. For these situations, manual data labeling tools make much more sense.
Projects with a vast amount of data, like self-driving software, require some degree of automation. The accuracy requirements of the data determine the degree of human involvement. For example, an automated keyword labeling tool needs less supervision than a self-driving data labeling tool.
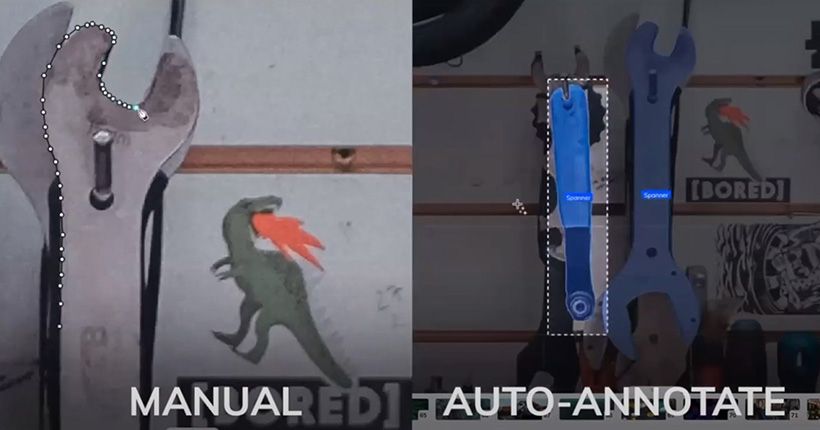
How Machine Learning Affects Our Future
Many people see machine learning programs as a net negative for the job market. We’ve already experienced this modernization in other industries, like auto manufacturing. Society was concerned that the internet would reduce jobs, but we’ve seen the opposite. It might be better to look at it as a restructuring, not a depletion. We already see a significant uptick in data-related jobs. Experts predict that AI will create a large number of jobs. Let’s look at some career paths and employment that machine learning can create.
Data Sourcing
Data is like digital gold in today’s world. Those who can collect it and earmark sources will be in high demand. Data sourcing professionals also guide and keep data labeling projects on track.
Data Labeling
Data labelers are also known as annotators. They are essential for creating the training data labeling for machine learning. We will only be able to automate our AI systems if annotators have trained them first. Data labelers are already in high demand, and that’s not expected to change. The market size of the data labeling tools industry is projected to grow by more than 27% between 2021 and 2028.
Data Analyst
Every boat needs a rudder. Airplanes need ailerons. Data needs analysts.
Even with incredibly productive machine learning programs, we sometimes need humans to give directions. For example, converting data into something actionable might need a human touch. An analyst can choose the next steps with an AI model's data.
AI Engineers
This is one of the fastest-growing job markets in the world. If AI was a thoroughbred stallion, AI engineers would be the jockeys. They’ll decide which systems can benefit from AI integration, then manage implementation.
The demand for AI specialists has grown by 74% yearly since 2016. There’s not much chance of this growth slowing anytime soon.
These are just a small selection of the employment opportunities that machine learning creates. Regarding automation and technology, the World Economic Fund made a prediction for 2025. 85 million jobs will be displaced, but 97 million new roles will be created. Even if their numbers are remotely accurate, we can expect millions of additional employment opportunities. There’s no doubt society will have to adapt, but new jobs will help the transition period.
Comments ()