A Code of Practice on Data Annotation for Autonomous Driving
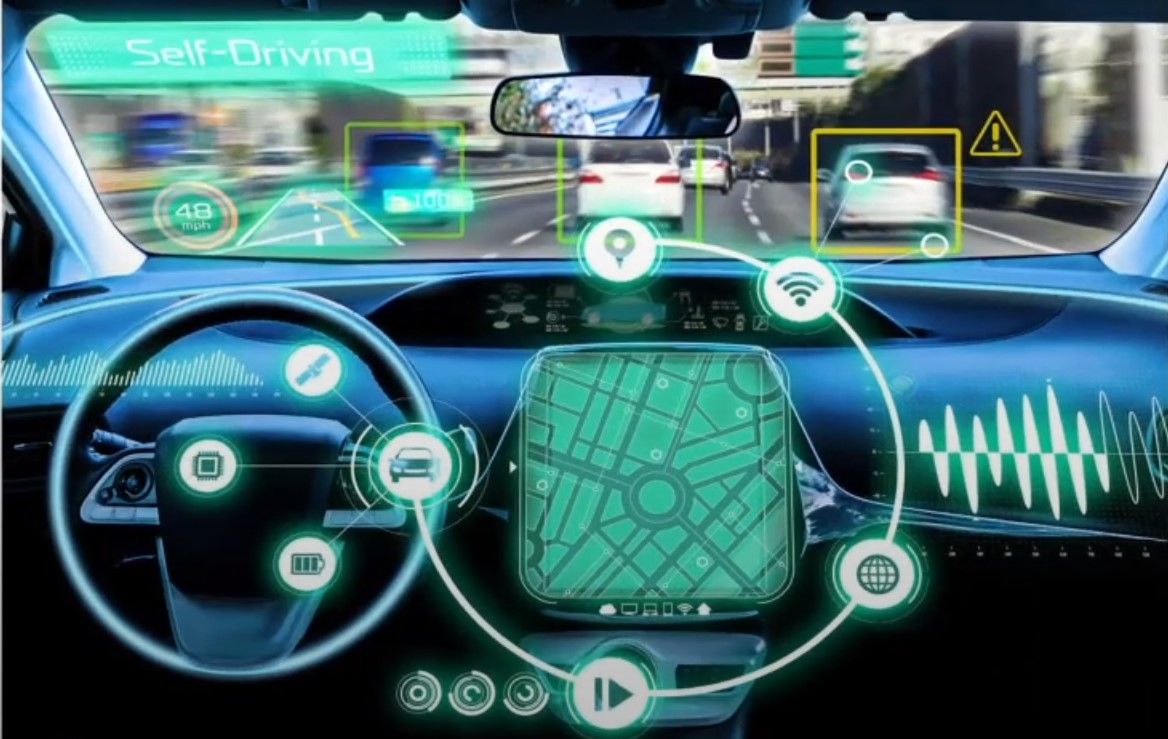
As the development of autonomous driving technology continues to progress, the need for high-quality data annotation has become increasingly important. Data annotation is the process of labeling and categorizing data sets to train machine learning algorithms. In the context of autonomous driving, this means labeling images, videos, and other sensor data to help self-driving cars recognize and respond to different driving scenarios.
In this article, we will explore the importance of data annotation in autonomous driving and discuss the various types of annotation techniques that are commonly used. We will also examine the challenges that come with data annotation and provide best practices for ensuring accuracy and consistency in the labeling process. Additionally, we will look at the tools and technologies that are available to aid in data annotation and provide case studies to demonstrate the real-world impact of this technology. Finally, we will discuss the future of data annotation in autonomous driving and the role it will play in the continued development of self-driving cars.
1. Introduction To Data Annotation For Autonomous Driving
Data annotation is an essential aspect of autonomous driving to ensure the safe navigation of self-driving vehicles. Autonomous cars use advanced driver-assistance systems (ADAS), which require neural networks trained through data input. Data management is critical in the development process, including data processing and analytics, to guarantee optimal downstream processing.
Manual data annotation can become a bottleneck; hence semi-automatic approaches can be more efficient in this regard. Large amounts of normalized and processed data are necessary for successful autonomous vehicle development, which is why open datasets such as the A2D2 dataset are useful for providing training information.
High-quality human-labeled AI training data for autonomous vehicles can impact safety levels and improve error-free navigation. Companies specialize in creating annotated datasets that provide comprehensive understanding about aspects that might affect safety or limit navigation accuracy.
Finally, deep learning and datasets have played an integral role in progress towards achieving better automation levels. However, it is necessary to develop more resources that provide a better overview of available datasets and their features. This would allow easier access to available information during development periods where testing requires vast amounts of relevant information.
2. Importance Of Data Annotation In Autonomous Driving
Autonomous driving relies on data annotation to ensure safe navigation of real-world environments. Labeled data is crucial for the ability of autonomous vehicles to detect and respond to objects on the road, including their nature and movement. This is essential in making advanced driver-assistance systems (ADAS) functions possible through neural network training and cross-validation on benchmarks. Without proper labeling, autonomous vehicles would be unable to differentiate between a pedestrian, a lamppost, or a signboard.
Moreover, data processing and analytics are used to produce the right set of labeled data that autonomous vehicles need for them to act correctly while navigating through uncertain scenarios. Annotation scope for autonomous driving encompasses several types of labels including 2D/3D bounding boxes, sensor fusion annotation, polygons, polylines, lane changes, semantic segmentation among others.
Environmental perception through semantic segmentation is critical in recognizing clear driving areas from surrounding obstacles in autonomous driving. However interestingly enough an Autonomous vehicle will require traversing at least 11 billion miles of engaged training that surpasses human ability before it can reach desired performance metrics using synthetic training alone.
3. Types Of Data Annotation Techniques
Object detection, data processing, and analytics are essential components in producing the right data for autonomous vehicle algorithms to act upon. There are various image annotation types available for use in autonomous vehicles, including polygons, bounding boxes, and semantic segmentation.
Bounding boxes and polygonal segmentation are popular annotation methods for object detection in autonomous driving. Bounding boxes draw rectangles around objects to identify their location in an image. Polygonal segmentation uses multiple vertices to map out the perimeters of objects with complex shapes. Incorporating these techniques into data annotation can improve object recognition accuracy.
Semantic segmentation is another vital technique that involves dividing images into segments based on similar characteristics such as color or texture. This method provides more contextual information about an object or scene compared to bounding boxes or polygons alone.
Choosing the right annotation tools and naming tasks is essential for automation in data annotation for autonomous vehicles. Automated tools can save time on manual labor, but they require proper configuration and training to ensure accurate results.
In summary, when annotating data for autonomous driving applications, it is necessary to consider multiple criteria such as accuracy requirements and tool availability. Implementing diverse and high-quality annotations which may include bounding boxes, polygonal segmentation or semantic segmentation will provide valuable insights needed by AI algorithms expand their understanding of what they see while operating autonomously on the roads ahead.
4. Challenges In Data Annotation For Autonomous Driving
Developing machine learning algorithms and deep learning networks for driverless systems require data collection and annotation, which are resource-intensive, challenging, and costly tasks. As the autonomous driving sector continues to grow, there is a need for end-to-end solutions that automate the data labeling process.
Accurately predicting the behavior of other road users is one of the hardest problems in autonomous driving, which makes the annotation of large amounts of structured labeled data critical to training computer vision systems in self-driving vehicles. Despite technological advancements in automation engines such as UAI, bias remains a persistent challenge in data used to train autonomous vehicle models. There is also an urgent need for additional validation and algorithm training.
Data annotation services are crucial for advancing autonomous driving technology. Automotive experience matters significantly in successfully completing large-scale annotation projects for self-driving cars. Opportunities present themselves through ADAS technology providing opportunities transformation of the automotive sector by shifting toward more intelligent solutions.
While automated solutions are essential regarding meeting AI's current demands, delivering accurate results benefits from having humans supplement AI training with human feedback as well natural knowledge about interpreting visual cues typically present on roads that define some safe rules. Incorporating good human judgment based on contexts such as traffic can eventually lead to braking bad biases in labeled datasets thus making self-driving vehicles safer ultimately increasing passenger confidence while preventing hazards along with greater efficiency both economically and environmentally.
5. Best Practices For Data Annotation In Autonomous Driving
Efficient data management with feature-rich and scalable tooling is crucial for successful autonomous driving software development. One of the most popular annotation methods for autonomous driving is bounding boxes and polygonal segmentation. However, automated scoring and 3D Gold Tasks can also improve scoring quality in data labeling for autonomous vehicles.
To train autonomous vehicles, it's important to have a best practice data split for training sets. A 70-80% split should be used for training sets while validation and test sets should be around 10-15%. Additionally, different types of data and their complexities need to be differentiated when labeling self-driving car datasets.
Annotation tool selection is critical in ensuring efficient and high-quality data labeling in autonomous driving. Object tracking algorithms based on machine learning can assist video annotation, making it an even more streamlined process.
Starting the annotation process with Data Scientists and SMEs can help "bootstrap" the dataset with their own answers. This can make sure the initial annotations are accurate, which will only allow automation to further improve accuracy over time. At its core then good practices start with choosing appropriate tools but goes much deeper than that by maintaining discipline during assembly annotations like proper staff training!
6. Case Studies On Data Annotation In Autonomous Driving
Today’s self-driving vehicles require significant edge cases that go beyond human capabilities, meaning timely and accurate labeling is crucial. Image annotation types such as polygons, bounding boxes, 3D cuboids, semantic segmentation, lines, and splines all provide the level of detail required for greater accuracy.
Despite the promises made by automated driving systems to provide safer and more efficient transportations solutions than ever before, recent reports indicate that fatalities involving automated vehicles are on the rise. This highlights the importance of enforcing a code of practice for data annotation in autonomous driving.
This is why Keylabs is one of the most trusted names in global technology services - providing high-quality video and image annotation tools specifically designed for autonomous transportation systems.
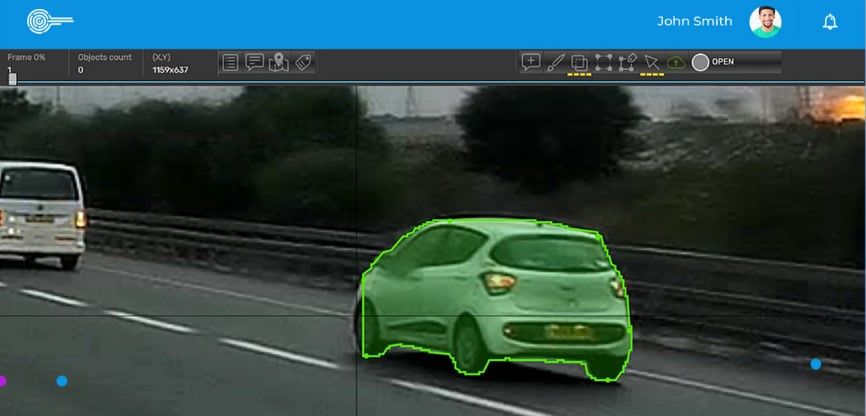
7. Future Of Data Annotation In Autonomous Driving
The landscape of data annotation in autonomous driving continues to evolve, with a shift towards automation. One way this is being realized is through the development of deep learning models that can automate annotation processes at scale. However, while automation offers significant benefits such as faster processing times and reduced costs, accuracy and precision are critical factors that require constant monitoring.
Currently, human oversight and intervention are still needed for quality control measures such as error checking and verification. Mislabelled data can negatively impact autonomous driving systems, leading to safety risks on the roads. Therefore, implementing accurate annotation standards and developing best practices for high-quality data collection remains a priority.
While automated data annotation will become increasingly important over time due to its scalability, it’s essential to recognize its limitations. For example, there may be situations where the system needs interception by human supervisors who have expertise in specific areas of road safety or engineering. Thus achieving reliable results requires striking a balance between automation and human intervention into the foreseeable future.
Although the future of data annotation in autonomous driving leads towards more automation from current operations involving traditional approaches like manual annotations or outsourcing work to experts; it's important we recognise that accurate annotations provide necessary context for training machine-learning based models upon which autonomy depends on so an adequate level of operator knowledge-to-data remains essential.
Comments ()