A machine-learning framework is able to detect gender stereotypes in movie scripts by the actions of characters
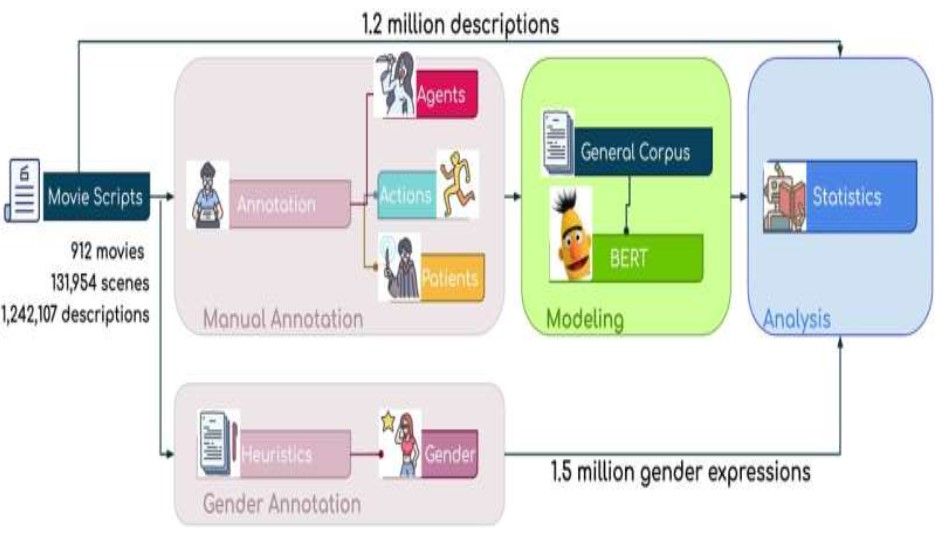
In the initial stage of the research, manual annotations were collected for 9,613 descriptions, as well as gender expression labels for over 1.5 million characters. As a result, a machine learning model was developed to identify actions, agents, and patients in movie scripts using natural language. Finally, statistical analysis was performed in order to identify portrayal differences across characters' portrayed attributes. The image was taken from Martinez et al., 2022, PLOS ONE, CC-BY 4.0 (creativecommons.org/licenses/by/4.0/).
Using scene descriptions in movie scripts, researchers have developed a novel machine-learning framework capable of automatically identifying the actions of different characters. Based on the application of the framework to hundreds of movie scripts, it can be found that the actions of the characters tend to reflect widespread gender stereotypes, some of which are consistent throughout history. On December 21, Victor Martinez and colleagues from the University of Southern California, U.S., published their findings in the open-access journal PLOS ONE.
Several traditional gender stereotypes are consistently portrayed in movies, TV shows, and other media, some of which may be harmful. The use of computational frameworks has been explored by some researchers as an efficient and accurate method of analyzing large amounts of character dialogue in scripts in order to gain a deeper understanding of this issue. However, some harmful stereotypes may not be expressed through the words of characters, but rather through their actions.
Using a machine-learning approach, Martinez and colleagues developed a computational model that is capable of automatically analyzing scene descriptions in movie scripts and identifying different character actions. This model was used to analyze over 1.2 million scene descriptions from 912 movie scripts produced between 1909 and 2013. They are identifying fifty thousand actions performed by twenty thousand characters.
The researchers then conducted statistical analyses to determine whether characters of different genders performed different actions. A number of differences were identified in these analyses that were attributed to gender stereotypes.
The researchers found that female characters tend to have less agency than male characters and are more likely to display affection than male characters. Characters are more likely to gawk or watch female characters than male characters, indicating a strong emphasis on appearances.
Even though the researchers' model does not fully capture the nuanced societal context connecting the script to each scene and narrative as a whole, these findings are consistent with previous research on gender stereotypes in popular media. As a result, people's real-life beliefs and behavior can be influenced as a result of media perpetuating harmful stereotypes.
It is possible to refine and apply the new machine-learning framework in order to include notions of intersectionality such as gender, age, and race in order to collect a deeper understanding of this issue.
According to the authors, "Researchers have proposed using machine-learning methods to identify stereotypes expressed by characters in media, but these methodologies do not take into account harmful stereotypes conveyed by character actions. This study aimed to develop a machine-learning framework that could identify character actions based on descriptions provided in movie scripts. We collected 1.2 million descriptions of scenes from 912 movie scripts on a large scale in order to study systematic gender differences in movie portrayals."
Src: Public Library of Science
Comments ()