Accurate Medical Image Annotation for Healthcare AI Solutions
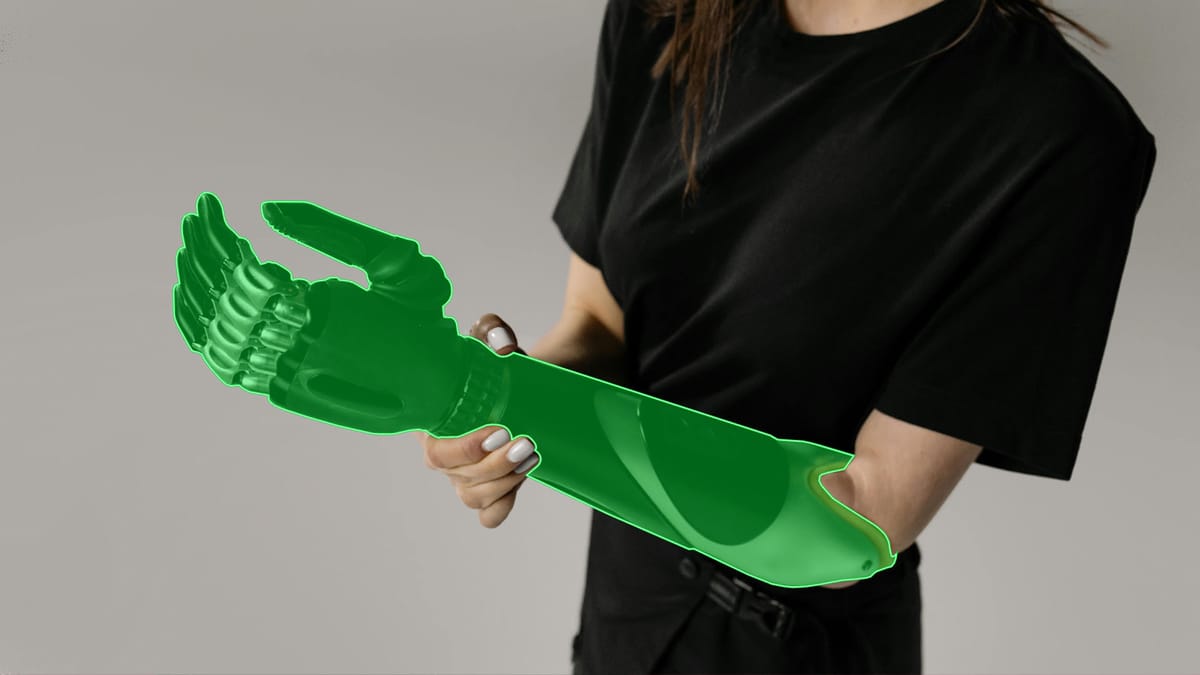
Whether in radiology, pathology, or other medical disciplines, precise medical image annotation is key to creating AI algorithms that accurately identify and classify health issues. Advanced annotation methods and expert verification guarantee the highest quality annotated data. This enables the creation of reliable medical imaging AI solutions.
Key Takeaways
- Accurate annotations are essential for training effective healthcare AI models.
- These services improve AI-driven diagnostics and patient care accuracy.
- Precision and scalability are key components of annotation services.
Importance of Medical Image Annotation in Healthcare
Accurate clinical image labeling empowers healthcare professionals to pinpoint medical conditions with enhanced precision. Annotations offer critical details that facilitate the correct interpretation of images, essential for diagnosing various health issues. This precision minimizes misdiagnoses, ensuring patients receive timely and appropriate treatment. The study underlines the critical role of high-quality annotations in training AI models for medical image analysis, highlighting their indispensable value.
Supporting Treatment Planning
Detailed diagnostic annotation software is vital for efficient treatment planning. It provides supplementary information through medical imaging annotation, enabling healthcare providers to formulate more effective treatment strategies. For example, annotations help in pinpointing the exact area affected on an image, facilitating precise interventions. This leads to enhanced patient care, backed by data-driven decisions.
The importance of medical imaging annotation is profound. It contributes to both immediate clinical needs and long-term healthcare advancements, driving innovations and groundbreaking discoveries in medicine.
Key Aspect | Relevance |
---|---|
Diagnosis Accuracy | Reduces misdiagnoses and helps in appropriate treatment selection |
Treatment Planning | Enables precise intervention based on detailed image analysis |
AI Model Training | Essential for developing accurate and efficient diagnostic tools |
Healthcare Advancements | Supports innovative research and improves healthcare practices |
Manual annotation is a foundational step in these endeavors, requiring precise spatial and temporal labeling by human annotators. Tools like ImageJ and 3D Slicer facilitate processing for AI research, underscoring the significance of medical image annotation. The precision of this process ensures that hospitals can effectively utilize the vast amounts of images, converting raw data into actionable medical insights.
Types of Medical Images
Medical images are vital in today's diagnostics, providing detailed insights for healthcare professionals. They include X-ray imaging, MRI scanning, and CT scan imaging. Each type offers unique advantages for diagnosing and monitoring different conditions.
X-rays
X-ray imaging is one of the oldest and most commonly used methods in radiology. It's highly effective for examining bones, joints, and certain tissues. X-rays reveal contrasts in different densities within the body. This is fundamentally beneficial for detecting fractures, bone malformations, and certain infections.
MRIs
MRI scanning, or Magnetic Resonance Imaging, uses powerful magnetic fields and radio waves to generate detailed images of soft tissues. It excels in visualizing organs, muscles, and connective tissues. MRI scans are essential for diagnosing conditions such as tumors, brain disorders, and ligament injuries.
CT Scans
CT scan imaging, or Computed Tomography, combines X-ray technology with computer processing to create cross-sectional images of the body. CT scans provide detailed information of both bones and soft tissues. They are invaluable for diagnosing complex conditions like cancers, cardiovascular diseases, and internal injuries.
Each of these medical image types plays a critical role in healthcare. They offer unique diagnostic capabilities that enhance patient outcomes and treatment planning. These diverse imaging techniques collectively advance our ability to understand and treat various medical conditions with precision.
Techniques for Medical Image Annotation
Medical image annotation labels medical data, mainly images, to train AI for disease detection. Accurate annotation is key to creating models that offer reliable diagnoses. This reduces errors in medical assessments. We'll explore manual and automated annotation techniques.
Manual Annotation Process
Manual annotation involves trained professionals labeling medical images. This method is critical for high-quality training data. It's essential for building accurate AI models in healthcare. Key techniques include:
- Image Classification Annotation: Categorizes images into normal and abnormal conditions, aiding decision-making.
- Object Detection Annotation: Identifies and labels specific objects or anomalies within images, aiding in locating abnormalities.
- Medical Image Segmentation Annotation: Delineates and labels organ or structure boundaries within an image for detailed understanding.
- Point Annotation: Focuses on identifying specific points within an image, essential for pinpointing anomalies at precise locations.
These techniques are vital for ensuring high-quality training data. They help develop algorithms that can detect diseases early by identifying subtle signs.
Automated Annotation Tools
Automated tools for annotation significantly improve the annotation process's efficiency and speed. These tools enable annotators to mark and label specific regions within medical images quickly. Notable features include:
- Advanced Image Processing: Tools like ImageJ and 3D Slicer aid in processing medical images for AI research.
- Anonymization Techniques: Crucial for protecting patient privacy during dataset preparation.
- Machine Learning Integration: Machine learning models accelerate image annotation, improving efficiency and accuracy.
Automated tools streamline the annotation process, ensuring consistency and scalability. They make handling large volumes of medical data possible. Combining manual precision with automated efficiency leads to robust anatomical annotation. This revolutionizes disease detection and early intervention.
Challenges in Medical Image Annotation
Medical image annotation is vital for healthcare AI, yet it encounters major hurdles. These issues stem from the variability in imaging data and the complexity of medical terms. The task requires precision, expertise, and consistency, making it both labor-intensive and complex.
Variability in Imaging Data
The variability in imaging data is a significant challenge. It arises from patient conditions, imaging equipment, techniques, and contrast levels. Achieving uniform annotations is hard due to this variability. Annotators need extensive exposure to diverse data to ensure consistent and accurate labels.
The challenges in image annotation are compounded by the vast amount of data. Cloud-based platforms and AI tools can improve efficiency. Yet, human expertise is essential for nuanced cases.
Complexity of Medical Terminology
The complexity of medical terminology adds to the difficulty of pathology image labeling. Annotators need deep medical knowledge for accurate labels. This specialized knowledge makes consistent labeling challenging, as medical terms evolve.
Terminology complexity also causes interobserver variability. Even experienced professionals may annotate the same image differently. Standardized and precise annotations are key for reliable AI models. For example, radiologist burnout can affect label quality.
Our research shows that image variability requires extensive training datasets. AI tools that blend human expertise with automation can reduce workload and improve accuracy.
Innovations like the AI-driven AIDE framework tackle these challenges. It optimizes datasets with noisy labels, boosting performance in various applications. Improving annotation efficiency is critical for healthcare AI to deliver better results.
Role of Artificial Intelligence in Annotation
Artificial intelligence (AI) is revolutionizing medical image annotation, boosting both efficiency and speed. It enables us to process vast amounts of medical imaging data at a pace unmatched by manual methods. The surge in AI and machine learning in healthcare, along with advancements in medical imaging, drives this expansion.
Improving Efficiency and Speed
AI significantly enhances annotation efficiency, essential for meeting the demand for telemedicine and personalized care. AI tools streamline the annotation process, cutting down the time needed for complex medical images. They quickly and accurately identify patterns and anomalies, aiding in clinical decision-making and improving patient care. For instance, deep learning models trained with precise images excel in detecting brain tumors and neuro-related conditions.
Reducing Human Error
AI's integration in annotation greatly reduces human error. It follows standardized guidelines and checks, leading to higher accuracy in medical images. This is critical for training AI models that aid in precise diagnoses across various medical fields. For example, AI models can significantly lower inaccuracies in liver disease diagnosis through quantitative assessments based on annotated images.
Combining AI with human validation ensures high-quality standards in large datasets. This hybrid approach reduces mistakes, making diagnostic and treatment planning tools more reliable. As detailed in this comprehensive review, AI and medical imaging are transforming medical diagnosis, patient care, and surgical precision.
Aspect | Impact of AI |
---|---|
Efficiency | Processes large datasets quickly |
Accuracy | Reduces human error, enhances reliability |
Diagnosis | Improves precision for cardiac, thoracic, and neurological issues |
Clinical Decision-making | Supports better treatment planning and outcomes |
Quality Assurance in Medical Image Annotation
In the field of medical imaging, quality assurance is essential. The accuracy and clarity of annotations are key to precise diagnoses and effective treatments. This begins with standardized annotation protocols and thorough review processes. These steps ensure high standards in medical image annotation.
Standardizing Annotation Protocols
Standardized annotation protocols are vital for consistency in annotated datasets. This method reduces variability, ensuring models are trained uniformly. The diversity and complexity of medical images highlight the need for such protocols. By following established guidelines, medical imaging AI quality improves, leading to reliable diagnostic results.
Implementing Review Processes
Implementing detailed review processes in annotation is essential for maintaining quality. Expert annotators perform multiple checks to ensure accuracy and consistency. Techniques like random sampling, peer reviews, and automated checks are used to validate annotations. This approach minimizes human error and supports precise data interpretation in medical diagnostics.
Quality Assurance Element | Description | Impact on Medical Imaging AI |
---|---|---|
Standardized Annotation Protocols | Ensures consistent and uniform annotation across datasets | Improves the accuracy of machine learning models |
Review Processes | Multiple layers of checks by expert annotators | Reduces human error and enhances data reliability |
Quality Control | Regular updates, continuous training, and feedback loops | Maintains high standards in medical image annotation |
By focusing on quality assurance through standardized protocols and robust review processes, we meet the high demands of AI diagnostics in medical imaging. This structured approach enhances medical imaging AI quality. It benefits patient outcomes and advances healthcare.
Applications of Annotated Medical Images
Annotated medical images are key to advancing healthcare fields. They improve clinical diagnosis accuracy and support AI model development. These labeled datasets are essential for research and AI diagnostics.
Research in Radiology
In radiology, these images offer detailed datasets for scientists. They help understand medical conditions and treatments better. Annotated images provide precise insights into disease progression and treatment responses.
Training AI Models for Diagnostics
Annotated medical images are vital for AI diagnostics training. They help AI models learn and interpret medical images accurately. This speeds up and improves diagnostic processes.
The global healthcare data annotation tools market was valued at USD 129.9 million in 2022. It's expected to grow at a CAGR of 27.5% from 2023 to 2030. This growth highlights the importance of high-quality datasets in AI diagnostics.
Quality in training data is critical. A smaller, high-quality dataset often outperforms a larger, lower-quality one. This focus on precision ensures AI models provide reliable and accurate results. It improves patient care and clinical decision-making.
Industry Standards and Regulations
Following industry standards in healthcare is essential for the reliability and ethical use of annotated medical images. By aligning with HIPAA compliance and DICOM standards, we ensure our medical image annotations meet legal and professional benchmarks. This secures patient data and supports seamless interoperability among various imaging systems.
HIPAA Compliance
HIPAA compliance is a critical aspect of any healthcare-related process, including medical image annotation. It ensures the privacy and security of patients' health information, which is of utmost importance. Respecting medical annotation regulations is not just about legal adherence but also about building trust with patients and healthcare providers. Implementing strict data protection protocols helps prevent unauthorized access and breaches, ensuring the confidentiality of sensitive medical data throughout the annotation process.
DICOM Imaging Standards
DICOM standards play a vital role in medical imaging by providing a universal format for storing and transmitting medical images. This standardization facilitates effective communication and interpretation of medical data across different healthcare systems and devices. By adhering to DICOM standards in our annotation processes, we ensure that our annotated images are easily accessible and compatible with various medical imaging tools, improving their utility in diagnosis and treatment planning.
Following industry standards in healthcare such as HIPAA and DICOM aids in maintaining high accuracy levels in healthcare data annotation. Accurate annotations are indispensable as they directly impact diagnoses, treatment plans, and patient outcomes. For more insights on the significance of accurate medical image annotation, you can visit this comprehensive guide.
Standard | Purpose | Benefits |
---|---|---|
HIPAA Compliance | Protect patient information | Ensures privacy, builds trust |
DICOM Standards | Interoperability of medical images | Improves communication, enhances diagnosis accuracy |
Trends in Medical Image Annotation
The future of medical image annotation is bright, thanks to rapid advancements in machine learning. These innovations promise to transform this field, making the process more efficient and accurate. Sophisticated algorithms and integration with Electronic Health Records (EHR) are key to this transformation.
Advances in Machine Learning
Machine learning has made tremendous progress, with deep learning at the forefront. Techniques like convolutional neural networks and generative adversarial networks are significantly improving annotation accuracy and efficiency. These technologies excel in identifying and categorizing complex image patterns, essential for precise medical diagnostics.
Transfer learning is also gaining traction, enabling the use of pre-trained models to reduce data requirements and training time. This enhances the overall efficiency of the annotation process. Bounding box annotation and semantic segmentation are evolving, allowing for more nuanced object recognition and classification down to the pixel level.
Integration with Electronic Health Records
EHR integration is a significant leap in image annotation trends. It combines annotated medical images with patient data, streamlining information flow. This leads to more insightful and personalized patient care. It also aids in developing AI-driven solutions that meet individual patient needs effectively.
Automation tools are increasingly used for real-time annotation, essential in rapidly evolving fields like autonomous driving and surveillance. The ability to immediately analyze images and integrate findings into electronic health records ensures healthcare professionals have the latest information for decision-making.
Looking ahead, the growing market for data annotation, projected to reach USD 5.3 billion by 2030, highlights the need for continuous innovation. From automated annotation tools to IoT device integration, these advancements promise to revolutionize medical image annotation and patient care.
Key Trends | Impact |
---|---|
Machine Learning Advances | Improved accuracy and efficiency in annotation |
EHR Integration | Streamlined information flow and personalized care |
Real-Time Annotation | Immediate decision-making for fast-paced applications |
Growth in Data Annotation Market | Increased demand for advanced annotation tools |
How to Choose a Medical Image Annotation Partner
Choosing the right medical image annotation partner is critical for AI-driven healthcare success. The healthcare data annotation tool market was valued at USD 129.9 million in 2022. It's expected to grow at a 27.5% CAGR from 2023 to 2030. Accurate and efficient annotations are essential for improving diagnostic precision and AI model training.
Evaluating Expertise and Experience
Assessing a partner's expertise in medical imaging modalities like X-rays, MRIs, and CT scans is key. Their experience with various projects and annotations gives insight into their ability to handle complex data. The support for standard formats like DICOM and TIFF is also vital for workflow integration. Radiology projects often require multiple experts, making collaborative tools essential.
Assessing Technology and Tools Used
Technology assessment in annotation tools is equally important. Look for partners using advanced tools like Labellerr, Encord, and 3D Slicer. These tools offer AI-assisted annotation for better efficiency and accuracy. Quality control is critical for reliable data, ensuring AI model training effectiveness. With varying tool costs, balancing budget with data security and privacy compliance is essential. Reliable support and a knowledgeable community can significantly improve tool effectiveness.
FAQ
What is the significance of accurate medical image annotation for AI-driven diagnostics?
Accurate medical image annotation is key for AI-driven diagnostics. It labels anatomical and pathological features in images precisely. This is essential for training AI models that assist in accurate diagnosis and treatment planning. It ultimately improves patient care outcomes.
Why is medical image annotation important in healthcare?
Medical image annotation is vital in healthcare. It enhances diagnosis accuracy and supports treatment planning. Precise labeling of clinical images aids in developing diagnostic software. This improves AI capabilities in medical imaging.
What types of medical images are commonly annotated?
Commonly annotated medical images include X-rays, MRIs, and CT scans. X-rays examine bones and joints. MRIs are best for soft tissues. CT scans provide detailed views of both bones and soft tissues, essential for radiological analysis.
What techniques are used for medical image annotation?
Techniques include manual annotation and automated tools. Manual annotation requires trained professionals to label images. Automated tools use software to process large datasets efficiently, ensuring quality through expert oversight.
What are the main challenges in medical image annotation?
Challenges include variability in imaging data and complex medical terminology. Different settings and patient conditions affect data. Extensive knowledge is needed for consistent annotations across diverse images.
How does Artificial Intelligence improve annotation efficiency and accuracy?
Artificial Intelligence boosts efficiency by processing large imaging data quickly. This reduces time compared to manual methods. AI also ensures accuracy by providing standardized guidelines, essential for reliable AI model training.
How do we ensure quality assurance in medical image annotation?
Quality assurance is ensured through standardized protocols and consistent annotations. Rigorous review processes with multiple checks by expert annotators verify accuracy. This maintains high standards for AI diagnostic solutions.
What are the applications of annotated medical images?
Annotated medical images are used in radiology research. They provide insights into conditions and treatment effectiveness. These datasets are also vital for training AI models, improving diagnostic accuracy and patient care.
What industry standards and regulations do we adhere to in medical image annotation?
We follow industry standards and regulations like HIPAA compliance and DICOM imaging standards. These ensure annotated medical images meet legal and ethical requirements. They secure patient data and maintain interoperability among systems.
What are the future trends in medical image annotation?
Future trends include advances in machine learning and integration with electronic health records (EHR). These trends streamline the annotation process. They allow for smooth information flow and deeper insights from medical images, improving AI-driven patient care.
How should one choose a medical image annotation partner?
When choosing a partner, evaluate their expertise and experience. Ensure they can handle medical data specifics. Assess their technology and tools for precise, high-quality annotations that aid in AI model training for advanced diagnostics.
Comments ()