Annotating Underwater Imagery: Techniques for Marine Life and Environments
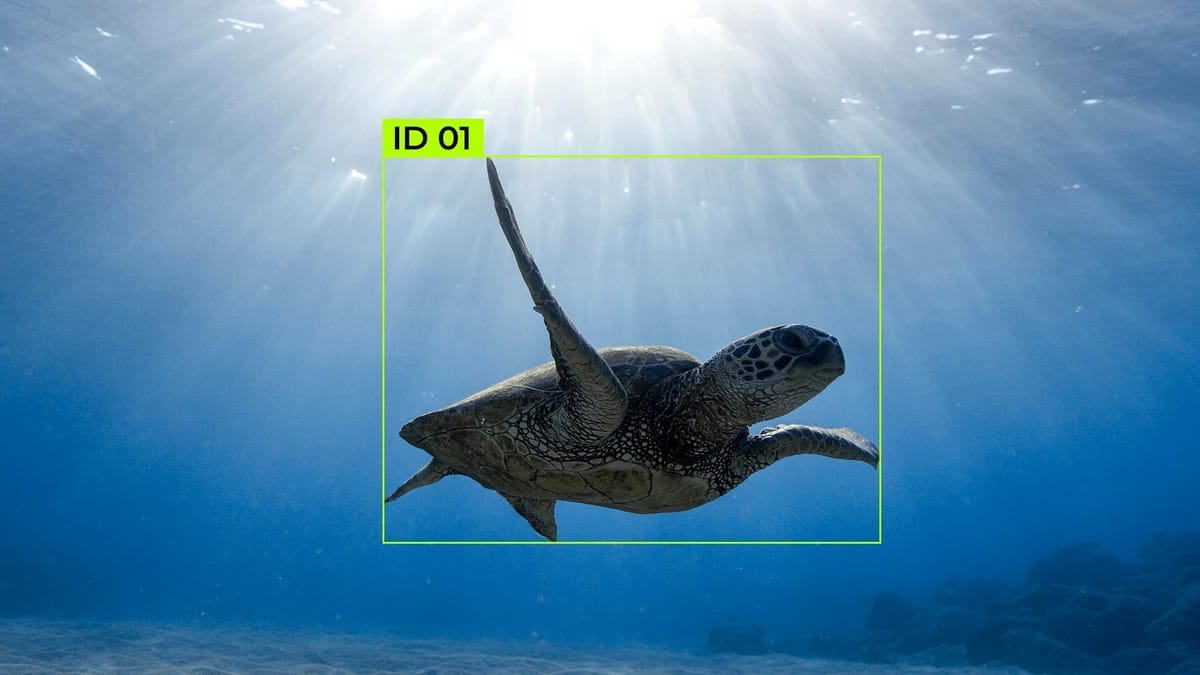
We are at the cusp of a technological revolution in underwater image labeling, essential for practical marine life annotation, environmental monitoring, and aquatic scene understanding. Dynamic underwater ecosystems present complex challenges that require innovative solutions through deep learning and crowdsourcing methodologies.
Key Takeaways
- Oceanographic monitoring advancements elevate observation scales, yet annotating underwater images remains challenging.
- Accurate marine life annotation is critical for environmental monitoring and understanding aquatic scenes.
- Deep learning algorithms and crowdsourcing platforms are improving marine imagery annotation efficiency.
- Advanced data labeling methods are essential in global research and conservation programs.
- The development of image annotation technologies helps to reveal the complex features of underwater ecosystems.
Importance of Underwater Image Labeling
Underwater image labeling assists with concentrating on marine biological systems, supports protection drives, and extends comprehension of sea life. Explanation includes gathering information and naming pictures of the remote ocean climate, permitting researchers to follow marine life and break down their territories.
Enhancing Marine Research and Conservation
This method significantly expands marine research capabilities, providing essential data for analyzing aquatic ecosystems. For example, in one study, about 950 underwater images were collected, of which 890 reference images were selected for the Underwater Image Enhancement Benchmark (UIEB) dataset. This dataset is used to create deep neural networks that improve the quality of underwater images, which in turn helps in accurately identifying species and studying their habitats.
Supporting Biodiversity Studies
Underwater image labeling picture explanation plays a key part in biodiversity research by recognizing and tracking marine species precisely. Notwithstanding, customary techniques frequently neglect to convey the ideal outcomes due to obscuring, decreased differentiation, and a variety of twisting commonplace of submerged symbolism. Advanced convolutional neural networks (CNNs), such as Water-Net, can improve image quality, enabling a more deep analysis of ocean ecosystems.
The UIEB dataset, created through manual segmentation, has become a valuable resource in research related to underwater image enhancement using deep learning. Thanks to authentic annotated images, researchers were able to generate high-quality samples for training neural networks, leading to significant breakthroughs in marine biodiversity research and conservation initiatives.
Continuous development of underwater image annotation methods remains a priority. As deep learning-based segmentation architectures become increasingly complex, they require large datasets with thousands of samples to work effectively. Using technologies such as CLAHE adaptive histogram alignment, Mahalanobis distance measurement, and K-means clustering has significantly improved the quality of underwater image segmentation, which positively impacts the preservation of marine ecosystems and biodiversity protection.
Manual vs. Automated Annotation
A manual explanation is still a technique generally involved in making excellent datasets, principally because it depends on the mastery of experts who can recognize subtleties in submerged pictures that robotized frameworks could ignore. This human touch guarantees that the datasets are exact and dependable. However, the downside is that this process can be incredibly time-consuming and demands a lot of workforces, which limits its practical use when dealing with large volumes of data.
The annotation landscape is changing with the rise of automated solutions powered by deep neural networks, like CNN, YOLOv3, and YOLOv7.
Use of Annotation Tools and Software
Annotation tools and underwater software applications are vital for improving annotation processes. Tools like Rep-UWnet and modules like CBAM and MSTB help overcome challenges like noise and image clarity, which are essential for practical underwater imaging.
Modern annotation tools offer features such as:
- Noise Reduction – Enhances image clarity by reducing noisy elements.
- Feature Prioritization – Uses CBAM to focus on the most informative parts of the image.
- Unified Frameworks – Combines various models and techniques to address underwater imaging challenges comprehensively.
By employing these advanced tools, we enhance the efficiency and accuracy of underwater image annotation. This empowers researchers and conservationists to analyze marine environments better and drives progress in marine biology and environmental studies.
Popular Tools for Underwater Image Labeling
In recent years, marine image analysis has become an important field of computer science. The development and use of effective underwater labeling software is key in this field. One of such tools is the Keylabs platform, which provides services to deliver high-quality labeled data.
Visual Complexity of Marine Environments
The intricacy of the marine climate, including its different water streams, sea designs, and dynamic water highlights, is a significant test. Even though practical and effective for object acknowledgment, optical cameras frequently experience the ill effects of picture quality issues because of these intricacies. This visual complexity can reduce image quality and make accurate labeling difficult even for experienced observers.
Detecting small objects in these environments and searching for them is complex. Deep learning methods, which have focused on underwater object detection, are necessary because they can learn feature representations from data.
Variable Lighting Conditions
The fluctuation of light in submerged photography adds to the difficulties. Submerged lighting conditions fluctuate because of water profundity, turbidity, and the presence of marine life. These circumstances can prompt changes in lighting that corrupt picture quality and compromise detail. Optical distortions and non-uniform illumination necessitate advanced image processing techniques such as de-hazing and color correction to enhance image clarity.
Marine research has developed various algorithmic approaches to compensate for these conditions. Yet, selecting the best algorithm is challenging, as reviews comparing enhancement techniques show varying results. Despite advancements in these technologies, the traditional approach of one observer manually annotating all image data in one session is becoming increasingly unfeasible due to the growing volume and higher resolution of marine imagery. Automated or semi-automated solutions are critical.
Developing robust, efficient annotation tools and methodologies remains critical as visual complexity and lighting variability continue to impact underwater image quality. Combining expert knowledge with advanced algorithms is essential to mitigate these image quality issues and improve the accuracy and Efficiency of underwater image annotations.
Standardizing Labeling Criteria
Ensuring consistency and accuracy in annotated datasets is a critical task. Standardization of labeling criteria plays a key role in achieving this goal. Keymakr offers advanced annotation tools that improve labeling accuracy, including automatic annotation and semantic segmentation. Standardized protocols are essential to avoid inconsistencies, especially considering challenges such as varied lighting, water transparency, and dynamic backgrounds.
- Implement benchmark methods with around four images labeled as benchmarks, ensuring all labelers follow the same quality guidelines.
- Conduct weekly group meetings and regular performance feedback sessions to track progress and address discrepancies.
- Use custom workflows in tools like Keylabs to tailor annotation processes to specific project needs, ensuring consistent results.
Machine Learning Applications in Image Labeling
AI and deep learning advances have fundamentally changed the way we name submerged pictures, working on the investigation of marine information. They work with more precise and quicker handling of intricate visual data. The review analyzes these advances given genuine cases and fruitful instances of artificial brainpower applications, exhibiting their utility and adequacy.
Advancements in Technology
Recent technological advancements are expanding the possibilities in underwater image labeling. The use of Autonomous Underwater Vehicles (AUVs) and Remotely Operated Vehicles (ROVs) in various underwater tasks, such as environmental inspections and seabed resource development, is becoming more common. Yet, underwater imaging faces challenges like red light absorption, which affects image quality by altering color balance.
Advancements like Manufactured Gap Sonar (SAS) imaging are making progress. SAS utilizes sound waves to make high-goal submerged pictures; however, water conditions can influence its goal. New strategies for submerged picture improvement are additionally being created, resolving issues like fogginess and detail misfortune caused by suspended particles.
Summary
The effect of computer-based intelligence on submerged naming is significant. Computer-based intelligence advances, for the most part, deep learning, have shown critical advancement in developing submerged picture quality. Models given convolutional brain organizations (CNN) and generative antagonistic organizations (GAN) are becoming progressively famous for their adequacy. For example, the Zero-UAE strategy improves brilliance, differentiation, and subtleties without requiring matched or unpaired information, accomplishing top execution on late benchmarks.
Considering submerged picture naming progressions, continuous improvement, and development are essential. Marine conditions' dynamic nature requires our strategies to develop for exactness and viability. The significance of submerged picture upgrades in helping object location execution is inevitable.
The fate of submerged naming depends on manageable practices and intensive exploration. Deep learning-based upgrades have shown giant steps, with models prepared on nitty-gritty picture matches further developing submerged picture quality.
FAQ
What is underwater image labeling?
Submerged picture naming is the method of commenting on submerged visual information. It includes recognizing and labeling marine species and environments in pictures or recordings. This method is key for marine exploration, supporting the observation and preservation of oceanic environments.
Why is underwater image labeling important for marine research and conservation?
It's imperative for marine exploration as it gives definite information. This information improves how we might interpret oceanic biological systems. It upholds biodiversity concentrates by working with the exact distinguishing proof and checking of marine species and natural surroundings. This contributes fundamentally to preservation endeavors.
How does underwater image labeling support biodiversity studies?
By precisely marking and recognizing marine species in submerged symbolism, analysts can screen populace elements, track changes in biodiversity, and study living space use designs. This information is fundamental for surveying the strength of marine environments and illuminating preservation techniques.
What are the main differences between manual and automated annotation techniques?
Manual annotation involves human experts labeling images, which can be time-consuming but highly accurate. Automated annotation uses algorithms and machine learning to label images quickly and consistently. It may require significant training data and validation to achieve high accuracy.
What tools and software are commonly used for underwater image labeling?
Different specific devices and programming are accessible for submerged picture marking. Models incorporate CoralNet, BIIGLE, and Video Comment and Reference Framework (VARS). These instruments offer highlights like robotized species location, easy-to-use connection points, and incorporation capacities with other examination frameworks.
What are the challenges in annotating underwater imagery?
Clarifying submerged symbolism is challenging because of the visual intricacy of marine conditions and variable lighting conditions. Factors like water lucidity, cloudy waters, and the development of sea-going life can influence picture quality and clarity, confusing exact explanations.
What are the best practices for achieving high-quality underwater image annotation?
Best practices consolidate normalizing stamping models to ensure consistency. Combining ace data from ocean life scientists is critical, in a like manner. Diligently endorsing is crucial for refreshing the clarifications. Using trustworthy instruments and staying aware of meticulous records add to first-rate results.
How is machine learning applied in underwater image labeling?
AI, mainly deep learning procedures, is utilized to examine and mark enormous datasets of submerged pictures. These advances can mechanize the identification of marine species and environments, upgrading the speed and precision of information comments.
What role do citizen science initiatives play in marine annotation?
Resident science projects draw people in general into logical exploration by including volunteers in information assortment and explanation assignments. These drive the upgrade of information quality and exploration results. They increment the volume of clarified information, encourage local area associations, and bring issues to light about marine preservation.
How should annotated underwater imagery be managed for optimal use?
Appropriate information for the executives includes sorting out and deliberately putting away massive datasets. It guarantees they are effectively open and usable for analysts. This incorporates keeping up with express metadata, reinforcement frameworks, and archives for consistent information sharing and recovery.
Are there any successful case studies of underwater image annotation in marine conservation?
A few contextual analyses feature the outcome of submerged picture explanation in marine preservation projects. Cooperative endeavors among specialists and partners have prompted critical disclosures and preservation triumphs, which exhibit the significance of precise and nitty-gritty picture comment.
Comments ()