Beyond Images: Can Segment Anything Be Used for Other Data Types?
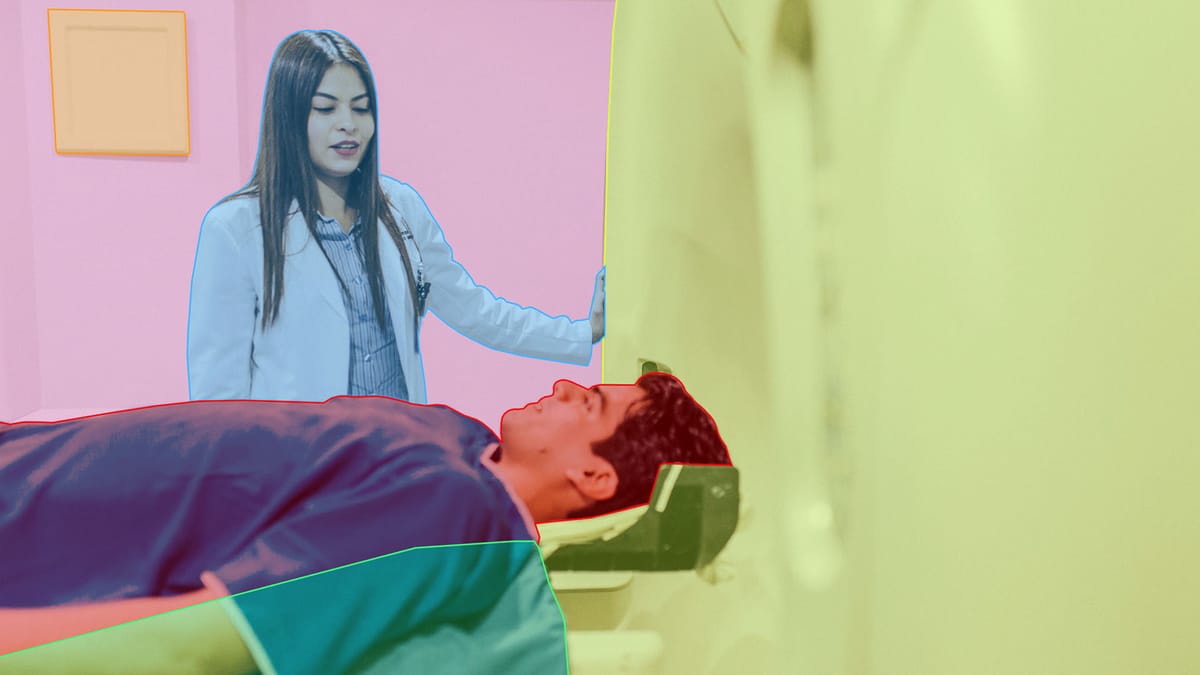
The Segment Anything Model (SAM) can automatically create segmentation masks for over 11 million images. This model, developed by Meta AI, is revolutionizing computer vision tasks. It can segment objects with just a click or an interactive selection, showcasing its power.
SAM uses a large dataset, the Segment Anything 1-Billion mask dataset (SA-1B), to segment objects it has never seen before. This highlights its exceptional zero-shot transfer capabilities. By releasing SAM and the SA-1B dataset under an open license, Meta AI is making segmentation more accessible. It reduces the need for specialized expertise and labor-intensive annotations.
Key Takeaways
- SAM focuses on promptable segmentation tasks, empowering users to interactively select objects to segment.
- Meta's Segment Anything Model can generate multiple valid masks, especially under uncertainty.
- The SA-1B dataset supports SAM's vast training, comprising over 1 billion images.
- Released as open source, SAM and its dataset aim to enhance research and application in computer vision.
- SAM's architecture combines image and prompt encoders with a mask decoder, employing a transformer-based approach derived from advanced NLP models.
Introduction to Segment Anything
The Segment Anything Model (SAM), a groundbreaking achievement from MetaAI's Segment Anything project, is revolutionizing image segmentation. It seamlessly blends interactive and automatic segmentation techniques. This makes SAM a versatile and powerful tool for diverse segmentation tasks. Furthermore, it is supported by the SA-1B dataset, the largest of its kind, with over 1 billion masks across 11 million images.
Background and Development
SAM emerged from the fusion of cutting-edge deep learning and extensive annotation datasets. It was created to overcome the shortcomings of traditional segmentation approaches. The model's architecture combines an image encoder, a prompt encoder, and a lightweight mask decoder. This synergy enables efficient, real-time image segmentation.
The iterative training process with the SA-1B dataset has significantly enhanced SAM’s performance. SAM can produce segments in just 50 milliseconds, with each mask annotation taking about 14 seconds. This speed makes SAM ideal for real-time applications and large-scale dataset creation, outperforming its predecessors.
Initial Applications in Image Segmentation
SAM's initial applications span a wide range of fields. It enhances scientific research by segmenting cell microscopy images and revolutionizes digital content creation through photo editing. SAM's ability to auto-generate diverse masks in response to prompts simplifies tasks that were once labor-intensive.
- Scientific Analysis: SAM's robust capabilities are being utilized to segment complex cell structures in imaging. This aids researchers in conducting faster, more precise analyses.
- Digital Media: In the realm of digital media, SAM's interactive segmentation tools significantly streamline tasks such as photo editing and video processing, enhancing workflow efficiency.
- Auto-annotation: SAM auto-generates high-quality masks, reducing the need for manual labeling. This cuts down on time and effort required for dataset creation.
With these advancements, SAM is setting the stage for new paradigms in image segmentation. It showcases that the future of adaptable and efficient image processing is already here. The foundation models like SAM are also opening up new possibilities and paving the way for innovative solutions in various image segmentation applications.
The Concept of Promptable Segmentation in SAM
The Segment Anything Model (SAM) is a groundbreaking tool that simplifies and improves the segmentation process. It combines interactive and automatic segmentation techniques. Released in April 2023 under the Apache 2.0 license, SAM offers a promptable interface. This interface adapts easily to new tasks, empowering users.
Interactive vs. Automatic Segmentation
SAM has blended the strengths of interactive and automatic segmentation. Users can guide the process with prompts like clicks, boxes, and text. This approach ensures accurate masks for specific needs. On the other hand, SAM's automatic segmentation is boosted by the Segment Anything Dataset (SA-1B), which includes over 11 million images and about 1.1 billion masks. This dataset allows SAM to create masks independently, with metrics like segmentation area and predicted IOU.
Zero-shot and Few-shot Learning
SAM's strength is enhanced by its zero-shot and few-shot learning capabilities. Zero-shot learning lets SAM segment new data types without extensive retraining. It has shown performance that rivals or beats fully supervised methods, using 1 billion masks from 11 million images. Few-shot learning enables SAM to adapt quickly to new tasks with minimal data. This makes it a crucial tool for rapid prototyping and deployment across various applications.
Model | Parameters | Speed | Accuracy | Release Date |
---|---|---|---|---|
SAM | ViT-B: 91M, ViT-L: 308M, ViT-H: 636M | 50 ms per segment | High | April 2023 |
FastSAM | 2% of SA1-B dataset | Faster than SAM | Less accurate | July 2023 |
As a versatile segmentation tool, SAM's core is its promptable interface. This interface combines interactive and automatic segmentation. By using zero-shot and few-shot learning, SAM adapts easily to new tasks. It maintains efficiency and accuracy.
Segment Anything Data Types: Expanding Beyond Images
The Segment Anything Model (SAM) goes far beyond just visual data, opening up new possibilities across various fields. Its ability to adapt makes it ideal for working with different data types. SAM's advanced segmentation techniques could change how we analyze images, text, and even AR/VR technologies.
Potential Applications in Textual Data
With SAM's powerful segmentation, textual data can be processed more accurately than ever. It combines deep learning with complex models to understand and segment text. This breakthrough could lead to better data analysis, making information extraction and categorization more precise.
Researchers and industries can use this to enhance natural language processing systems. These systems will become more efficient and responsive.
Uses in Multi-modal Learning
Integrating SAM into multi-modal learning environments is a game-changer. It blends visual and textual data for a deeper understanding of data. This approach improves performance in tasks that involve multiple data types.
In education, SAM can make learning experiences richer, leading to better engagement and understanding. It's a powerful tool for educational technology.
Beyond AR/VR: New Frontiers in Data Usage
SAM's applications also reach into AR/VR, where it can handle complex tasks. By combining text and computer vision, SAM enhances user interactions and 3D object manipulation. This is key for developing innovative AR/VR solutions in gaming, simulations, and education.
How Segment Anything Can Be Applied to Scientific Data
The Segment Anything Model (SAM) is transforming the analysis of scientific data. It goes beyond simple image segmentation, showing great promise in cell microscopy and environmental monitoring.
Segmenting Cell Microscopy Images
In cell microscopy, SAM's advanced segmentation is key. It accurately identifies microscopic structures, offering new insights into cellular structures. Researchers can now analyze cells with unmatched precision.
Interactive tools like point selection and bounding boxes allow for real-time segmentation in 50 milliseconds. This speeds up the analysis significantly. SAM's image encoder, based on the Masked AutoEncoder model, reconstructs complex images with ease. This is vital for biological research, where understanding cell components at a microscopic level is essential.
Applications in Environmental Monitoring
Environmental monitoring also benefits greatly from SAM. Its segmentation techniques, such as fast mask decoding, allow for detailed analysis of natural phenomena. This helps track environmental changes like deforestation, pollution, and wildlife populations.
With its ability to process large datasets, SAM is a powerful tool for researchers and environmentalists. Its efficiency and accuracy make it crucial for accurate and timely environmental monitoring.
SAM in Medical Imaging: Prospects and Challenges
The advent of the Segment Anything Model (SAM) in medical imaging heralds a new era for diagnostic precision and cost reduction in data annotation. SAM's integration into segmenting medical images, such as X-rays or MRIs, is set to revolutionize the accuracy of identifying anomalies and pathologies. This advancement will significantly benefit both medical professionals and patients.
Enhancing Diagnostic Procedures
By leveraging SAM for medical imaging, the accuracy of segmenting medical data is significantly enhanced, which is essential for precise diagnostic procedures. This model excels in predicting multiple output masks from a single prompt, thereby improving the detection of lesion regions across diverse anatomical structures and imaging modalities. SAM's extensive dataset, including the SA-1B dataset with over 11 million images and a billion masks, underscores its capability in processing and understanding complex medical images. Moreover, SAM's zero-shot transfer potential allows it to adapt to new diagnostic tasks without extensive retraining, thus streamlining medical imaging workflows.
Reducing Annotation Costs in Medical Data
The potential of SAM extends to significantly reducing the high annotation costs associated with medical data. With datasets like MedSAM featuring over 1.5 million image-mask pairs across various imaging modalities and cancer types, SAM excels in automating data annotation tasks. This automation not only reduces the workload of healthcare professionals but also ensures consistent and accurate mask generation. Furthermore, SAM's performance across 86 internal and 60 external validation tasks highlights its robustness and adaptability in diverse medical imaging scenarios. By minimizing the need for manual intervention, SAM has the potential to lower healthcare costs and expedite the diagnostic process. The precision of SAM’s generated masks meets the high accuracy demands of medical data annotation.
Below is a comparative overview of SAM and the curated medical segmentation datasets employed for evaluation.
Aspect | SAM | Curated Medical Segmentation Dataset |
---|---|---|
Image-Mask Pairs | 1 Billion Masks | 6033K Masks |
Image Modalities | 18 Modalities | 10 Modalities |
Cancer Types | Multiple Types | Over 30 Types |
Training Sets | 11 Million Images | 1,050K 2D Images |
Validation Tasks | Unlimited Potential | 86 Internal, 60 External |
Enhancing Content Creation and Digital Media with SAM
The Segment Anything Model (SAM) by Meta AI is transforming content creation and digital media with its advanced segmentation capabilities. It enables new possibilities in image and video editing, as well as generating real-time visual effects. These features streamline workflows, leading to unprecedented creativity and efficiency.
Image and Video Editing
SAM excels in generating precise segmentation masks, trained on over 11 billion such masks. This makes it invaluable for image and video editing. It allows for effortless object isolation, making complex alterations and seamless compositing possible in projects. The model's architecture, featuring an image encoder, a prompt encoder, and a mask decoder, ensures high accuracy and flexibility.
Generating Real-time Visual Effects
For digital media, creating real-time visual effects is crucial for interactive experiences and advanced video editing. SAM stands out by using its prompt encoder to handle various human prompts, including points, bounding boxes, and text prompts. This capability enhances real-time visual effects, making workflows more efficient and significantly enriching the creative process.
Integrating SAM into your content creation and digital media workflows simplifies complex tasks, accelerates production timelines, and opens up new avenues for innovation. Whether fine-tuning the model for specific datasets or leveraging its zero-shot performance, SAM emerges as a versatile tool in today's digital media and content creation realms.
Integrating SAM with Autonomous Systems
The integration of the Segment Anything Model (SAM) with autonomous systems is set to revolutionize various fields. This advanced model excels in object detection and enhances perception in autonomous vehicles and robotics. Since its inception, SAM has shown remarkable efficiency, speed, and accuracy in segmentation tasks crucial for autonomous vehicles and robotics in industrial settings.
SAM's impact on autonomous systems goes beyond mere enhancements; it significantly reduces annotation time, boosting model performance. Data shows SAM can process pixel-level segmentation much faster than traditional methods. Its swift conversion of bounding boxes to instance masks highlights its efficiency. This rapid annotation minimizes label noise, enhancing model performance well beyond the capabilities of simpler annotation forms like bounding boxes.
Object Detection in Autonomous Vehicles
Object detection is essential for the safety and efficiency of autonomous vehicles. SAM's promptable segmentation capability enables vehicles to accurately identify and respond to their environment. This is crucial in real-world scenarios where quick detection and reaction can prevent accidents and improve road safety.
Robotics and Industrial Applications
In robotics and industrial applications, SAM's integration into autonomous systems is transformative. The model's real-time detection and interaction with objects are key for tasks needing high precision and adaptability. As automation evolves in industries, SAM enables robots to perform complex operations with greater accuracy and speed.
Industrial applications also greatly benefit from SAM's high accuracy in detecting diverse images and objects. Its extensive dataset, trained on 1 billion masks (SA-1B), supports improved object recognition capabilities. SAM's integration into Kognic's platform ensures easy adoption and flexibility across various industrial contexts.
The integration of SAM into autonomous systems, from vehicles to robotics and industrial applications, highlights its revolutionary potential. With its high-speed performance, accuracy, and efficiency, SAM is set to become crucial in the future of autonomous systems. It will drive advancements in numerous sectors and applications.
Training and Fine-tuning SAM for New Data Types
Training and fine-tuning the Segment Anything Model (SAM) for new data types is crucial. This process involves adjusting the model to fit specific datasets and tasks. It boosts the model's performance and makes it more adaptable across different domains. For example, fine-tuning SAM on 200–800 images typically yields the best results, showcasing the model's versatility.
The architecture of SAM includes an image encoder, prompt encoder, and mask decoder, facilitating effective segmentation adaptation. Using control points and bounding boxes as prompts during training is a notable approach. Recent studies indicate that point prompts outperform bounding boxes for tasks like river segmentation. This makes SAM a dependable tool for certain environmental applications.
Thanks to resources like Google Cloud Platform (GCP)'s free $300 credit and an Nvidia T4 GPU through Google Colab, even the largest SAM model, sam-vit-huge, can be trained efficiently. For instance, training on 1000 images for 50 epochs can be finished in under 12 hours. This makes deep learning techniques like SAM more accessible to a wide audience.
The continuous adaptation of SAM through fine-tuning keeps it relevant in both academic research and commercial settings. Its open-source nature allows users to tailor SAM for their specific needs. This versatility cements its importance in future data segmentation and analysis.
FAQ
Beyond Images: Can Segment Anything Be Used for Other Data Types?
Yes, Segment Anything (SAM) extends beyond image segmentation. Its framework supports various data types, showcasing its prowess in textual data and multi-modal learning. This broadens its use beyond traditional computer vision and deep learning tasks.
What is the background and development of Segment Anything?
Meta AI developed Segment Anything to revolutionize image segmentation. The SAM model and the SA-1B dataset, the largest for segmentation, aim to make segmentation more accessible. They reduce the need for specialized expertise and labor-intensive annotations.
What are the initial applications of Segment Anything in image segmentation?
Initially, SAM was applied in scientific image analysis and photo editing. It has transformed tasks by automatically generating various masks without large volumes of manually annotated data. This showcases its advanced generalization capabilities.
What is the concept of promptable segmentation in SAM?
Promptable segmentation in SAM enables user-guided segmentation through different prompts like clicks, boxes, and text. This interactive capability supports more accurate mask generation. It enhances the model’s adaptability and precision in segmentation tasks.
How does SAM differ between interactive and automatic segmentation?
SAM combines interactive and automatic segmentation methods. Users can guide the segmentation process with prompts. At the same time, SAM’s advanced algorithms ensure accurate and automatic segmentation. This merges person-guided interactions with automated analysis.
What are zero-shot and few-shot learning in SAM?
Zero-shot and few-shot learning enable SAM to adapt quickly to new data types and tasks without extensive retraining. These learning capabilities allow SAM to generalize from minimal examples. This makes it highly efficient and versatile in various applications.
What are the potential applications of SAM in textual data?
SAM’s versatile architecture allows it to be applied to textual data for analysis and segmentation. This capability enhances the model’s utility in natural language processing and other AI systems that integrate visual and text-based information.
How does SAM contribute to multi-modal learning?
SAM’s ability to handle multi-modal data facilitates learning from combined visual and textual inputs. This opens avenues for more comprehensive data interpretation. It is particularly beneficial for AI systems requiring integrated analysis from various data sources.
What are some new frontiers in data usage beyond AR/VR with SAM?
Beyond AR/VR, SAM’s promptable segmentation enhances user interactions and 3D object manipulations in immersive technologies. It also offers potential in fields like digital media, scientific research, and more for enriched, interactive experiences.
How is SAM applied to segmenting cell microscopy images?
In scientific applications, SAM is adept at segmenting cell microscopy images. This allows researchers to perform detailed analysis and interpretation of biological data. It enhances the capability to study microscopic entities with precision.
What are the applications of SAM in environmental monitoring?
SAM can significantly improve environmental monitoring by accurately segmenting and analyzing natural phenomena. Its precision aids in tracking and studying environmental changes. This contributes to a better understanding and management of natural resources.
How does SAM enhance diagnostic procedures in medical imaging?
SAM enhances diagnostic procedures by accurately segmenting medical images like X-rays and MRIs. This capability helps healthcare professionals to pinpoint anomalies and pathologies with greater precision. It improves diagnosis and patient care.
How can SAM reduce annotation costs in medical data?
By automating the generation of precise masks in medical imaging, SAM reduces the reliance on extensive manual annotation. This can lower healthcare costs and streamline the diagnostic process. It enhances the efficiency of medical data analysis.
How does SAM enhance content creation and digital media?
SAM aids content creators by making image and video editing more efficient through its advanced mask generation. It facilitates real-time visual effects, streamlining production timelines. This allows for more creative freedom in digital media projects.
What are the applications of SAM in object detection for autonomous vehicles?
SAM’s promptable segmentation helps autonomous vehicles accurately detect and respond to their surroundings. This capability enhances the safety and operational efficiency of autonomous systems. It is critical for reliable automotive technologies.
How does SAM integrate with robotics and industrial applications?
In robotics and industrial applications, SAM can perform real-time detection and interaction with various objects. This precision is vital for automated tasks requiring high adaptability. It revolutionizes practices in industrial automation.
How can SAM be fine-tuned for new data types?
SAM’s framework allows it to be trained and fine-tuned for new data types. This adaptability ensures its ongoing relevance across diverse fields. Users can customize the model for specific industry needs or research goals, broadening its impact.
Comments ()