Choosing the Right Outsourcing Partner for Data Labeling.
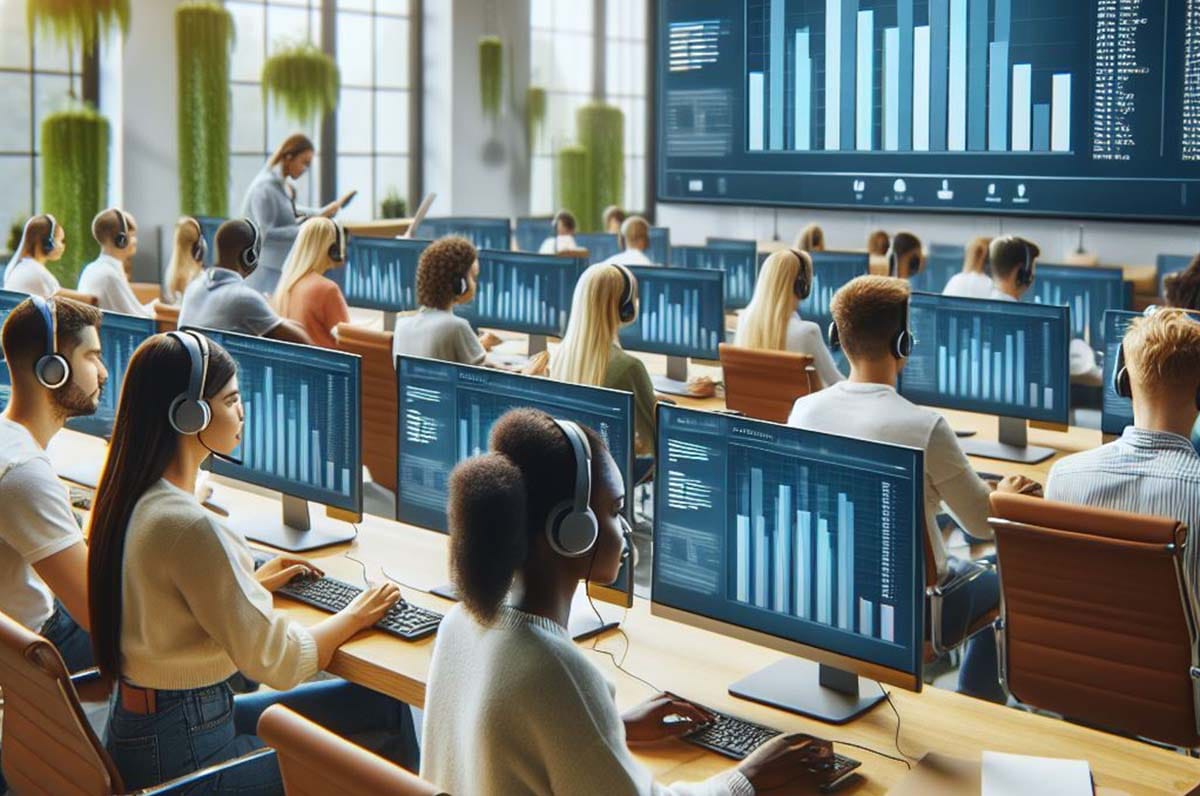
Data labeling plays a crucial role in AI and machine learning projects, as it involves annotating and tagging data to train and improve algorithms. However, data labeling can be a time-consuming and resource-intensive task for businesses. That's where outsourcing data labeling services comes into play. By partnering with the right outsourcing provider, companies can streamline their data labeling processes and achieve more accurate and reliable results.
When it comes to outsourcing data labeling, making the right choice is essential. The success of your AI and machine learning initiatives depends on the expertise and capabilities of your outsourcing partner. That's why it's crucial to consider several key factors before making a decision.
Key Takeaways:
- Outsourcing data labeling services can help businesses streamline their data labeling processes and achieve more accurate results.
- Choosing the right outsourcing partner is critical for the success of your AI and machine learning projects.
- Consider factors such as expertise, quality control, scalability, security, and cost-effectiveness when selecting an outsourcing partner.
- Different types of data labeling services, including data annotation, computer vision labeling, image labeling, and text annotation, are available.
- Following best practices, such as training data annotators and leveraging automation, ensures high-quality labeled data.
Understanding the Need for Outsourcing Data Labeling
As businesses increasingly rely on data-driven technologies and advancements in artificial intelligence (AI), the need for accurate and reliable labeled data has become paramount. However, the process of data labeling is not without its challenges. In-house data labeling can be time-consuming, resource-intensive, and prone to errors. That's where outsourcing data labeling services comes into play.
Outsourcing data labeling services to specialized data labeling companies has gained popularity among businesses looking for efficient and cost-effective solutions. By leveraging the expertise and infrastructure of external partners, companies can ensure high-quality labeled data for their AI and machine learning projects. Moreover, outsourcing data annotation tasks allows organizations to focus on their core competencies while reducing operational costs.
"Outsourcing data labeling services not only addresses the challenges of in-house labeling, but it also provides access to a skilled and dedicated workforce that can handle large volumes of data."
Outsourcing data labeling offers several key advantages. Firstly, it allows businesses to tap into a vast pool of trained data annotators who possess domain-specific knowledge. These skilled professionals are experienced in handling diverse data types, such as images, videos, text, and sensor data, and can accurately label the data according to specific requirements. Additionally, data labeling companies often have stringent quality control measures in place to ensure the accuracy and consistency of the labeled data.
Outsourcing data annotation services also provides scalability and flexibility to meet the changing needs of AI projects. As the volume of data increases or project requirements evolve, outsourcing partners can quickly scale up their resources to handle the workload effectively. This scalability is particularly important in applications such as computer vision labeling, where large datasets need to be labeled with precision and efficiency.
Furthermore, partnering with a data labeling company can enhance data security and compliance. These outsourcing providers prioritize data privacy and confidentiality, implementing robust security measures to protect sensitive information. This is especially crucial when dealing with proprietary data or datasets containing personally identifiable information (PII).
Overall, outsourcing data labeling services offers a comprehensive solution to overcome the challenges associated with in-house labeling. It enables businesses to streamline their AI projects, access expert knowledge and resources, maintain data security, and achieve higher accuracy in labeled data.
Key Considerations When Selecting an Outsourcing Partner
When it comes to outsourcing data labeling services, choosing the right partner is crucial for the success of your AI and machine learning projects. To ensure the highest quality results and a smooth collaboration, there are several key considerations to keep in mind.
Expertise
First and foremost, evaluate the expertise of potential data labeling companies. Look for partners with experience in your specific domain and data types. A deep understanding of computer vision, natural language processing, or other relevant technologies is essential. Consider the complexity of the labeling tasks you require and assess if the outsourcing partner has the necessary skills and knowledge to handle them.
Quality Control
Quality control is a critical aspect of data labeling services. Check if the outsourcing partner has robust quality assurance processes in place. Look for companies that employ well-trained annotators and follow industry best practices. Their guidelines should be clear, ensuring consistency and accuracy in the labeled data. Feedback loops and validation processes should also be implemented to continually improve the quality of the annotations.
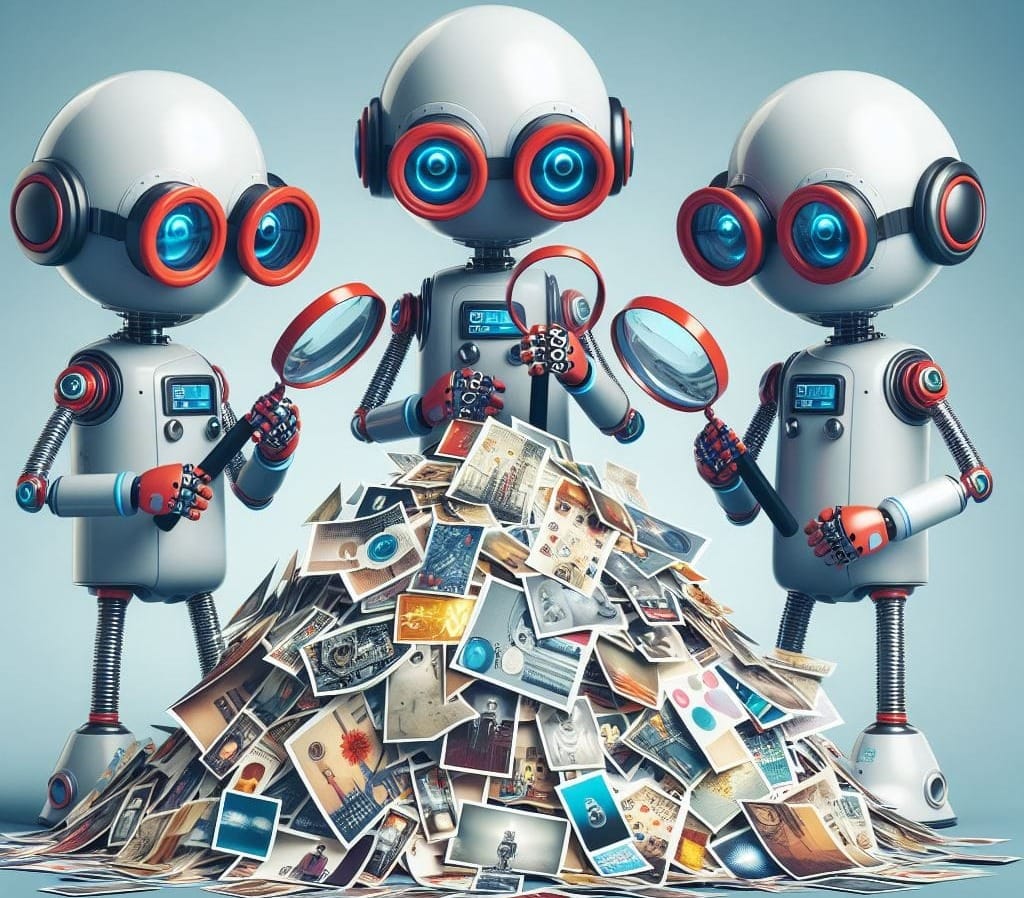
Scalability and Security
Scalability is important, especially if you anticipate working with large volumes of data. Make sure the outsourcing partner has the capacity to handle your project requirements efficiently and within the agreed-upon timelines. Additionally, data security is paramount when sharing sensitive information. Ensure the partner adheres to strict security protocols, including secure data transfer, storage, and access controls.
Cost-effectiveness
Consider the cost-effectiveness of the outsourcing arrangement. Compare pricing models offered by different data labeling companies and assess the value you will receive in return. Remember, low cost does not necessarily equate to high quality. Look for partners that offer competitive pricing while maintaining the standards and expertise you require.
By considering these key factors when selecting an outsourcing partner for data labeling, you can make an informed decision that aligns with your project goals, resources, and requirements.
Types of Data Labeling Services Available
When it comes to data labeling, outsourcing partners offer a range of specialized services to meet the diverse needs of AI and machine learning projects. These services include data annotation outsourcing, computer vision labeling services, image labeling services, and text annotation services.
Data annotation outsourcing involves the process of manually labeling data to provide accurate annotations for training AI models. Whether it's categorizing objects in images, transcribing audio, or annotating text, data annotation services play a crucial role in generating high-quality labeled datasets.
Computer vision labeling services focus specifically on image and video data. Skilled annotators meticulously annotate objects, regions of interest, and other visual elements to train computer vision models. These services enable the development of robust image classification, object detection, and segmentation models.
Image labeling services are essential for projects that require precise and detailed annotations on images. Whether it's highlighting specific features in medical imaging, identifying facial expressions in emotion detection systems, or marking objects in autonomous vehicle datasets, image labeling ensures accurate training data for AI algorithms.
Text annotation services involve labeling and categorizing textual data, such as documents, customer reviews, or social media posts. Annotators extract key information, perform sentiment analysis, or identify entities to create labeled datasets. These services are vital for natural language processing, sentiment analysis, and text classification applications.
Choosing the Right Service for Your Project
When selecting a data labeling service, it's important to assess the specific requirements of your project and choose an outsourcing partner with expertise in the relevant domain. Whether you need precise image annotations, accurate text analysis, or comprehensive data annotations, finding the right service provider will ensure the success of your AI initiatives.
By leveraging data annotation outsourcing, computer vision labeling services, image labeling services, and text annotation services, businesses can access high-quality labeled datasets that fuel the development and training of AI models.
Data Labeling Service | Description |
---|---|
Data Annotation Outsourcing | Manual labeling of data to provide accurate annotations for training AI models. |
Computer Vision Labeling Services | Precise annotation of image and video data for computer vision model training. |
Image Labeling Services | Detail-oriented annotations on images to enable robust AI algorithms. |
Text Annotation Services | Categorization and labeling of textual data for natural language processing applications. |
Best Practices for Data Labeling
Data labeling companies play a critical role in providing accurate and high-quality labeled data for AI and machine learning projects. To ensure the effectiveness of data labeling processes, it is essential for these companies to follow best practices that optimize efficiency and maintain data integrity. Here, we will discuss some of the key best practices that data labeling companies should implement.
Training and Expertise
One of the crucial aspects of data labeling is having well-trained data annotators who understand the specific requirements of the project. Data labeling companies should invest in comprehensive training programs that cover various labeling techniques, domain-specific knowledge, and adherence to guidelines. This ensures consistent and accurate labeling results.
Clear Guidelines and Instructions
Establishing clear labeling guidelines and instructions is essential for consistent and standardized data labeling. These guidelines should define labeling criteria, quality standards, and any specific labeling conventions. Clear instructions help data annotators understand the labeling requirements and produce consistent labeled data.
Leveraging Automation Technologies
The use of automation technologies can significantly enhance the efficiency and accuracy of data labeling processes. Data labeling companies should integrate automated data labeling tools that leverage machine learning algorithms and AI models to reduce manual effort and improve label consistency. Automation technologies also enable scalability to handle large volumes of data.
Continuous Quality Control
Implementing robust quality control processes is crucial for data labeling companies to ensure the accuracy and reliability of labeled data. This includes regular checks, validations, and feedback loops to identify and rectify any labeling errors or inconsistencies. Continuous quality control measures help maintain the integrity of the labeled dataset.
Collaboration and Communication
Effective collaboration and communication between data labeling companies and their clients are vital for successful data labeling projects. Regular communication helps address any ambiguities, clarifications, or additional requirements, ensuring that the final labeled dataset reflects the client's objectives accurately.
Security and Confidentiality
Data labeling companies also need to prioritize data security and confidentiality. Implementing robust measures to secure client data, establish access controls, and comply with data protection regulations is essential. This instills trust and confidence in clients that their data is handled with utmost care and confidentiality.
To summarize, data labeling companies can optimize their processes and deliver high-quality labeled data by adhering to best practices that encompass well-trained annotators, clear guidelines, automation technologies, continuous quality control, effective collaboration, and robust data security measures.
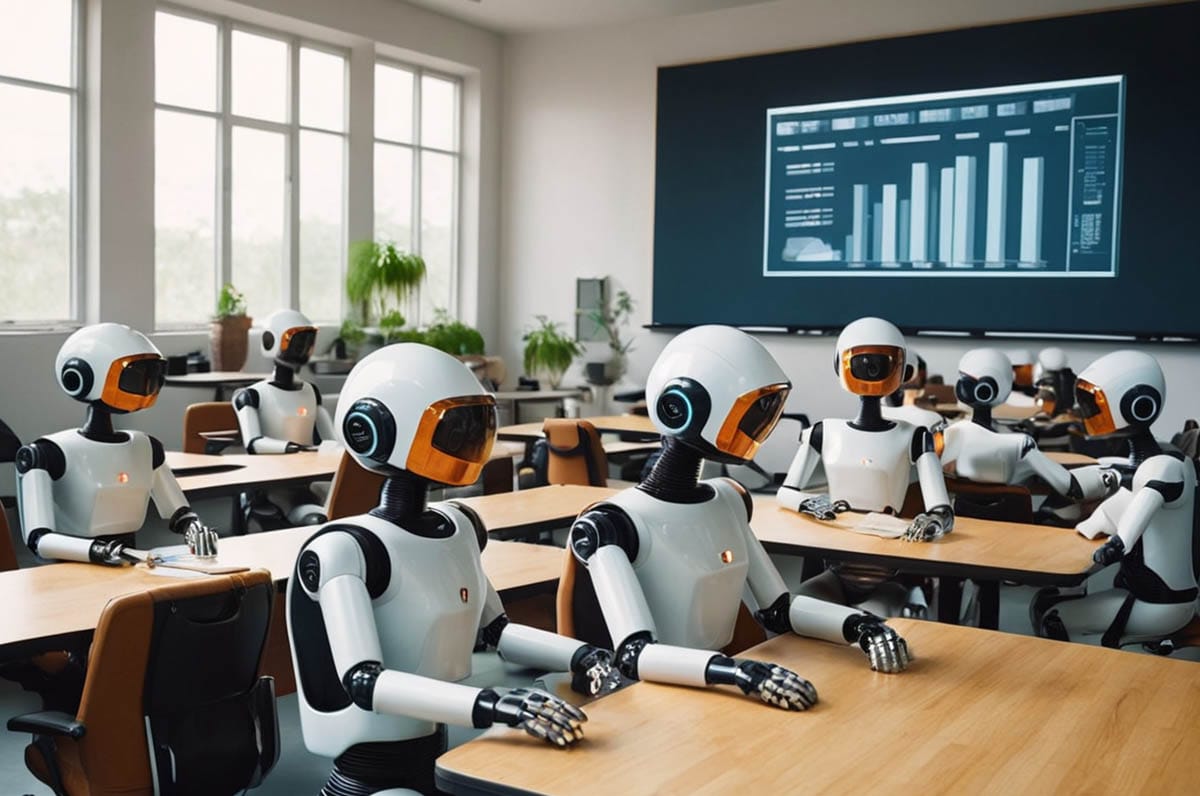
Assessing the Expertise of Outsourcing Partners
When choosing an outsourcing partner for data labeling, assessing their expertise becomes crucial in ensuring the success of your AI and machine learning projects. By evaluating the knowledge, skills, and capabilities of potential partners, you can make an informed decision and find the right match for your specific needs.
Evaluating Knowledge and Experience
One of the key factors to consider when assessing an outsourcing partner is their domain-specific knowledge. Look for companies that have experience in your industry or niche, as they will have a deeper understanding of the data labeling requirements and challenges specific to your field.
Additionally, consider the types of data they specialize in labeling. Whether it's images, text, videos, or audio, make sure the outsourcing partner has a proven track record in handling similar data formats. This expertise will ensure accurate and relevant annotations that contribute to the overall success of your AI models.
Technical Capabilities and Technologies
Another crucial aspect of expertise evaluation is the outsourcing partner's technical capabilities. Check if they have a robust infrastructure and access to the latest data labeling tools and technologies. This ensures that they can efficiently handle large volumes of data and provide advanced labeling solutions.
Moreover, consider their ability to handle complex labeling tasks. Look for partners who have experience in handling intricate annotation requirements, such as object detection, image segmentation, or natural language processing. Their expertise in these areas will directly impact the quality of the labeled data and the performance of your AI models.
"Finding an outsourcing partner with domain knowledge and technical expertise is essential for accurate and reliable data labeling."
Quality Assurance Processes
It's important to assess the quality assurance processes of potential outsourcing partners. Look for companies that have stringent quality control measures in place to ensure the accuracy and consistency of the labeled data.
Ask about their validation processes and how they handle feedback loops. This shows their commitment to continuous improvement and their ability to adapt based on your specific requirements. A reliable outsourcing partner should be able to provide transparent insights into their quality assurance practices.
Case Studies and Client Reviews
One effective way to gauge the expertise of an outsourcing partner is by reviewing their case studies and client testimonials. Look for concrete examples of their work in similar projects or industries, as this will provide valuable insights into their capabilities and results.
Client reviews and testimonials can also give you a glimpse into the outsourcing partner's reliability, communication, and overall experience. Pay attention to feedback related to expertise, responsiveness, and the ability to meet project deadlines.
Choosing the Right Partner
Assessing the expertise of potential outsourcing partners is crucial for finding the right fit for your data labeling needs. By evaluating their knowledge, experience, technical capabilities, quality assurance processes, and client reviews, you can make an informed decision that aligns with the goals of your AI and machine learning projects.
Ensuring Data Security and Compliance
When it comes to outsourcing data labeling services, data security and compliance should be top priorities for organizations. The sensitive nature of the data being labeled, including personal information and proprietary data, requires robust measures to ensure its privacy, confidentiality, and adherence to regulatory requirements.
Partnering with reputable data labeling companies that prioritize data security is essential. These companies should have stringent security protocols in place to protect against unauthorized access, data breaches, and other cyber threats. They should also have a clear understanding of data protection laws and regulations to ensure compliance.
One measure that outsourcing partners should implement is the use of secure data transfer protocols. This includes encryption methods, such as SSL/TLS, to safeguard data during transit. Additionally, they should have secure storage systems in place that utilize encryption and access controls to prevent unauthorized disclosure of data.
Another crucial aspect is the establishment of strict access controls and authentication mechanisms. By implementing role-based access controls, outsourcing partners can ensure that only authorized personnel have access to sensitive data. This helps minimize the risk of data leaks and ensures that labeling activities are performed by trusted individuals.
Data anonymization techniques should also be employed to protect the privacy of individuals whose data is being labeled. By removing personally identifiable information from the data, outsourcing partners can mitigate the risk of re-identification and unauthorized use.
Outsourcing partners should have comprehensive data handling policies and procedures that are in line with industry best practices and regulatory requirements. These policies should address data retention, data disposal, and data breach response protocols to handle any potential incidents effectively.
Moreover, it is crucial for outsourcing partners to comply with relevant regulations and standards, such as the General Data Protection Regulation (GDPR) or the Health Insurance Portability and Accountability Act (HIPAA), depending on the nature of the data being labeled. Compliance with these regulations helps ensure that organizations are not exposed to legal and reputational risks.
By partnering with outsourcing companies that prioritize data security and compliance, organizations can confidently leverage their expertise in data labeling, knowing that their data is handled with the utmost care and confidentiality.
Remember, the responsibility of ensuring data security and compliance does not solely lie with the outsourcing partner. Organizations should also establish clear contractual agreements that outline the security measures to be implemented and conduct regular audits to ensure compliance.
Data Security and Compliance Checklist:
- Select outsourcing partners with robust data security protocols
- Ensure secure data transfer protocols, such as encryption methods
- Implement role-based access controls and authentication mechanisms
- Use data anonymization techniques to protect privacy
- Establish comprehensive data handling policies and procedures
- Comply with relevant regulations and standards
- Establish clear contractual agreements and conduct regular audits
Data Security Measures | Compliance Framework |
---|---|
Data encryption during transit and storage | Adherence to data protection regulations |
Strict access controls and authentication mechanisms | Compliance with relevant industry standards |
Data anonymization techniques | Alignment with privacy regulations |
Comprehensive data handling policies and procedures | Adherence to legal requirements |
Regular security audits and assessments | Establishment of contractual agreements |
In conclusion, ensuring data security and compliance is essential when outsourcing data labeling services. Organizations should prioritize partnering with outsourcing companies that have robust measures in place to protect data privacy, confidentiality, and comply with relevant regulations. By following best practices and conducting regular audits, organizations can minimize the risks associated with data labeling while maximizing the benefits of outsourcing.
Scalability and Flexibility in Data Labeling Services
When it comes to outsourcing data labeling, scalability and flexibility are two crucial factors that organizations must consider. The ability to handle large volumes of data and adapt to changing project requirements can significantly impact the success of an AI or machine learning initiative.
Data annotation outsourcing partners with expertise in computer vision labeling services are well-equipped to tackle the scalability challenges associated with data labeling. With a dedicated team of skilled annotators and the right infrastructure in place, these outsourcing partners can efficiently handle and process large datasets.
Automated data labeling is another essential aspect that contributes to both scalability and flexibility. By leveraging advanced algorithms and machine learning technology, outsourcing partners can automate the data labeling process, accelerating the speed and efficiency of labeling tasks. This not only saves time and resources but also ensures consistent and accurate results across the board.
"Outsourcing data labeling services to specialized companies enables businesses to tap into a vast pool of resources and expertise. These partners can quickly scale up or down based on project needs, ensuring efficient data annotation and labeling processes."
Furthermore, outsourcing partners often have the necessary infrastructure and resources to handle diverse labeling requirements. Whether it's image labeling services, text annotation services, or other specialized labeling needs, these partners can adapt to different data types and labeling methodologies.
One effective way to gauge the scalability and flexibility of an outsourcing partner is by assessing their track record and client portfolio. Look for case studies or testimonials that demonstrate their ability to handle complex projects and deliver high-quality labeled data within tight deadlines.
Benefits of Scalability and Flexibility:
- Efficient handling of large volumes of data
- Adaptability to changing project requirements
- Accelerated data labeling process with automation
- Consistent and accurate results across datasets
- Ability to handle diverse labeling requirements
Comparison of Scalability and Flexibility of Data Labeling Services
Criteria | Data Annotation Outsourcing | Computer Vision Labeling Services | Automated Data Labeling |
---|---|---|---|
Scalability | High | High | Extreme |
Flexibility | High | Medium | High |
Speed | Medium | High | High |
Accuracy | High | High | High |
Cost | Varies | Varies | Varies |
By partnering with an outsourcing company that exhibits strong scalability and flexibility, businesses can ensure the smooth execution of their data labeling projects. This allows them to focus on core competencies while harnessing the power of accurate and labeled data for AI and machine learning advancements.
Quality Control and Assurance Processes
In the world of data labeling services, ensuring the accuracy, consistency, and reliability of the labeled data is of utmost importance. Quality control and assurance processes play a crucial role in achieving these goals. By implementing rigorous validation processes and feedback loops, data annotation outsourcing companies can maintain high-quality standards. Let's explore some of the key methodologies that are commonly employed:
1. Validation and Verification
Validation and verification are essential steps in the quality control process. Verification involves cross-checking the labeled data by comparing it with ground truth or previously labeled data to ensure consistency and accuracy. Validation, on the other hand, involves verifying the quality of the labeling by checking against predefined quality metrics and criteria. This helps identify any errors, inconsistencies, or deviations in the labeled data.
2. Inter-Annotator Agreement
Inter-annotator agreement is a process that measures the consistency and reliability of annotations performed by different annotators. It helps assess the level of agreement between annotators and determine the overall quality of the labeling. By analyzing the inter-annotator agreement, data labeling companies can identify areas where the guidelines may need clarification or where further training of the annotators might be necessary.
3. Continuous Feedback Loops
Establishing a feedback loop with annotators is crucial for maintaining consistent quality. Providing timely feedback on their work helps them understand their strengths and areas for improvement. It also ensures that any issues or challenges in the data labeling process are addressed promptly. This iterative feedback loop contributes to achieving a higher overall quality of the labeled data.
"Continuous feedback loops enable data labeling companies to refine their processes, address emerging challenges, and maintain a high standard of quality throughout the annotation process."
4. Quality Assurance Metrics
Implementing quality assurance metrics allows data labeling companies to measure and monitor the quality of their labeling services. These metrics can include accuracy, precision, recall, and F1 score, among others, depending on the specific requirements of the project. By regularly measuring these metrics, companies can track their performance and make necessary adjustments to achieve the desired quality levels.
Additionally, data labeling companies may also employ specific techniques depending on the type of data being labeled. For example, in image labeling services, techniques like bounding box overlap analysis or object recognition algorithms can be used to ensure accurate annotations.
By implementing robust quality control and assurance processes, data labeling companies can deliver high-quality labeled data that meets the requirements of AI and machine learning projects. These processes contribute to building reliable and trustworthy datasets, enabling the development of accurate and efficient AI models.
Benefits of Quality Control and Assurance | Challenges Overcome |
---|---|
|
|
Cost-Effectiveness of Outsourced Data Labeling
Evaluating the cost-effectiveness of outsourcing data labeling services is a crucial consideration for businesses looking to enhance their AI and machine learning projects. By partnering with a reputable data labeling company, organizations can optimize their resources and achieve significant cost savings.
When assessing the cost-effectiveness of outsourcing data labeling services, various factors come into play. Let's explore some key considerations:
1. Pricing Models
Data labeling companies offer different pricing models to suit the diverse needs of businesses. These may include per-hour rates, per-task pricing, or project-based costs. Understanding the pricing models available and aligning them with your budgetary requirements is essential in making an informed decision.
2. Cost Factors
Outsourcing data labeling can help reduce operational costs associated with hiring and managing an in-house team. By outsourcing, businesses eliminate expenses related to recruitment, training, infrastructure, and employee benefits. Additionally, outsourcing allows companies to access skilled professionals at a fraction of the cost compared to building an internal team.
3. Return on Investment (ROI)
Partnering with a data labeling company can yield a significant return on investment. Accurate and high-quality labeled data enhances the performance and accuracy of AI and machine learning models. This improvement in performance translates into operational efficiency, better decision-making, and ultimately, increased revenue and customer satisfaction.
By leveraging the expertise and experience of data labeling professionals, businesses can accelerate their AI initiatives and achieve faster time-to-market, resulting in competitive advantages.
Consider this quote from an industry expert:
"Outsourcing data labeling allowed us to focus on our core competencies while ensuring the accuracy and quality of our training data. We witnessed remarkable improvements in AI model performance, which directly translated into better business outcomes. The cost savings associated with outsourcing were an added bonus."
Ultimately, the cost-effectiveness of outsourcing data labeling services can be evaluated by analyzing the pricing models, cost factors, and the potential return on investment. Businesses that prioritize accuracy, quality, and efficiency while optimizing costs are more likely to experience success in their AI and machine learning endeavors.
Factors to Consider Before Making a Decision
Before finalizing an outsourcing partner for data labeling services, organizations should carefully consider several key factors. By taking these aspects into account, businesses can ensure they choose a reliable and competent partner that aligns with their specific needs. Here are some crucial factors to consider:
1. Expertise and Experience
Verify the outsourcing company's expertise and experience in the field of data labeling. Look for evidence of their knowledge in areas such as AI data labeling services, image labeling services, and text annotation services. A reputable company should have a proven track record of successfully completing similar projects.
2. Quality Control Measures
Assess the outsourcing partner's quality control processes. Ensure they have robust mechanisms in place to maintain the accuracy and consistency of data labeling. This can include review cycles, validation techniques, and feedback loops to address any errors or inconsistencies.
3. Scalability and Flexibility
Consider the scalability and flexibility offered by the data labeling company. They should be able to handle large volumes of data and adapt to changing project requirements, ensuring a seamless workflow without compromising on quality or deadlines.
4. Data Security and Confidentiality
Ensure that the outsourcing partner has stringent data security measures in place. Confidentiality agreements, secure data transfer protocols, and adherence to regulatory guidelines should be part of their standard procedures. This is particularly important when dealing with sensitive data.
5. Cost-effectiveness
Evaluate the cost-effectiveness of the outsourcing solution. Compare pricing models and consider the value provided by the data labeling company. Look beyond the cost per annotation and consider factors such as accuracy, turnaround time, and overall project success rate.
6. Communication and Collaboration
Assess the communication and collaboration channels offered by the outsourcing partner. Open lines of communication and a collaborative approach are essential for a smooth and productive partnership. Look for a company that prioritizes regular updates, feedback, and collaboration throughout the data labeling process.
Choosing the right outsourcing partner for data labeling services is a decision that can significantly impact the success of AI and machine learning projects. By considering these key factors, organizations can make an informed decision and ensure a fruitful partnership.
When evaluating potential outsourcing companies, it's important to conduct thorough research, review case studies, and even request sample labeling to assess the quality of their work. By taking a systematic and diligent approach, businesses can find the right partner to support their data labeling needs and accelerate their AI initiatives.
Conclusion
Choosing the right outsourcing partner for data labeling is essential for AI and machine learning projects. By outsourcing data labeling services, businesses can leverage the expertise of specialized companies and ensure accurate and high-quality labeled data. This, in turn, enhances the performance of AI algorithms and models, leading to more reliable and precise outcomes.
Throughout this article, we have explored the various aspects of outsourcing data labeling. We discussed the benefits of data annotation outsourcing, computer vision labeling services, and text annotation services, highlighting the value they bring to organizations. We also explored the importance of scalability, flexibility, and data security when selecting an outsourcing partner.
An effective outsourcing partner for data labeling should possess expertise in AI data labeling services and provide data labeling solutions that align with the unique needs of the project. They should have robust quality control and assurance processes in place to ensure accuracy and consistency, and they should offer cost-effective services that provide a solid return on investment.
By carefully considering these factors and selecting the right outsourcing partner, businesses can unlock the full potential of their AI and machine learning initiatives. Outsourcing data labeling services enables organizations to focus on their core competencies while leveraging the expertise of dedicated data labeling companies, ultimately accelerating the development and deployment of AI-powered solutions.
FAQ
Why is choosing the right outsourcing partner for data labeling important?
Choosing the right outsourcing partner for data labeling is important because it can significantly impact the success of AI and machine learning projects. The expertise and capabilities of the outsourcing partner can ensure accurate and high-quality labeled data, which is vital for training and improving AI models.
Why should businesses consider outsourcing data labeling services?
Businesses should consider outsourcing data labeling services because it offers several advantages. It allows companies to tap into the specialized knowledge and experience of data labeling companies, saves time and resources, enables scalability to handle large volumes of data, and ensures access to a diverse pool of data annotators.
What are the challenges of in-house data labeling?
In-house data labeling can pose several challenges for businesses. It requires significant investments in terms of infrastructure, technology, and skilled annotators. Managing and coordinating the labeling process internally can be time-consuming and distract from core business activities. In addition, it can be difficult to ensure consistency and quality control in the labeling process.
What factors should be considered when selecting an outsourcing partner for data labeling?
When selecting an outsourcing partner for data labeling, several factors should be considered. These include the partner's expertise in specific domains and technologies, their ability to handle complex labeling tasks, the quality control measures they have in place, their scalability and flexibility to adapt to project requirements, and the cost-effectiveness of their services.
What types of data labeling services are available from outsourcing partners?
Outsourcing partners offer various data labeling services to cater to different needs. These services may include data annotation, computer vision labeling, image labeling, text annotation, and more. These services involve labeling or tagging different types of data such as images, videos, texts, or audio to train AI models and enhance their accuracy.
What are the best practices for data labeling?
Data labeling companies should follow several best practices to ensure accurate and high-quality labeled data. These practices may include proper training of annotators, establishing clear labeling guidelines, leveraging automation technologies to enhance efficiency, implementing validation processes and feedback loops for quality assurance, and regularly updating the labeling guidelines based on feedback or changes in project requirements.
How can the expertise of outsourcing partners be assessed?
To assess the expertise of outsourcing partners, businesses can evaluate their knowledge and experience in specific domains, data types, and technologies relevant to the project. It is crucial to review their track record, case studies, and client testimonials to gauge their competence in handling similar projects. Communication with the potential partner and requesting sample annotations can also provide insights into their skills and understanding.
How do outsourcing partners ensure data security and compliance?
Data security and compliance are critical considerations when outsourcing data labeling services. Reliable outsourcing partners have comprehensive security measures in place to protect data confidentiality and privacy. These measures may include strict access controls, secure data transmission protocols, encrypted storage systems, and adherence to applicable data protection laws and regulations.
How can outsourcing partners handle scalability and flexibility in data labeling services?
Outsourcing partners should have the capability to scale their operations to handle large volumes of data within tight deadlines. They should have the flexibility to accommodate changes in project requirements and adapt their processes accordingly. This may involve efficiently allocating resources, utilizing cloud-based infrastructure, and implementing agile project management methodologies.
What is the role of quality control and assurance in data labeling services?
Quality control and assurance are crucial to ensure accurate and reliable labeled data. Outsourcing partners should have robust quality control processes in place, including thorough checks for accuracy, consistency, and adherence to labeling guidelines. Validation processes and feedback loops help identify and address any issues or discrepancies, ensuring the highest quality of labeled data.
How can the cost-effectiveness of outsourced data labeling be evaluated?
Evaluating the cost-effectiveness of outsourced data labeling requires considering various factors. These include the pricing models offered by the outsourcing partner, such as per-hour rates or per-data unit rates, and comparing them with the costs of in-house labeling. Additionally, businesses should consider the potential return on investment in terms of improved AI model performance, time savings, and resource optimization.
What factors should organizations consider before finalizing an outsourcing partner for data labeling?
Before finalizing an outsourcing partner for data labeling, organizations should consider factors such as the partner's expertise, experience, and track record in relevant domains and technologies. They should also assess the partner's scalability, pricing models, quality control processes, security measures, and ability to handle changing project requirements. Seeking referrals, conducting interviews, and reviewing case studies can help make an informed decision.
Comments ()