Comprehensive Data Annotation Solutions for Industry-Specific Applications
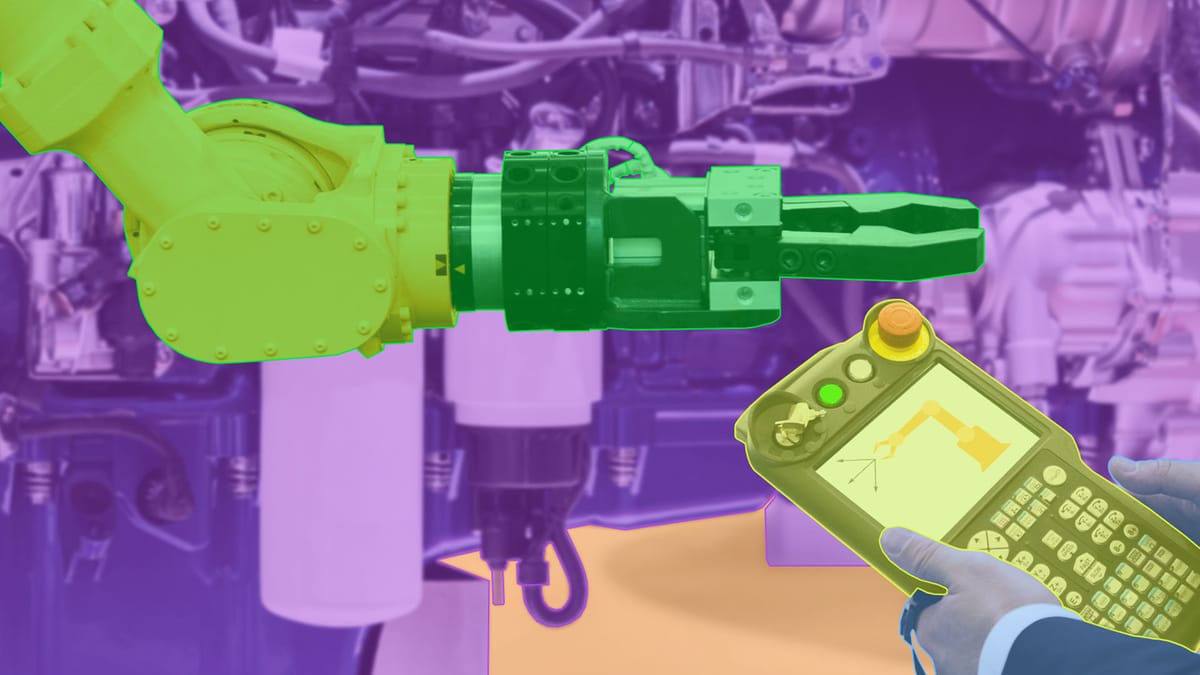
Across industries like healthcare, automotive, e-commerce, and more, specialized data annotation is vital. It allows for the customization of AI training data to specific sector requirements. These services combine the expertise of skilled annotators with AI technologies. This combination is vital for improving annotation quality and speeding up model development, all while upholding ethical AI standards and robust data security.
Key Takeaways
- High-quality training data is critical for AI and machine learning success.
- Data annotation solutions are key to boosting AI across industries.
- Specialized data annotation meets sector-specific AI training needs.
- Collaboration between human annotators and AI technologies enhances quality.
- Emphasis on ethical AI and data security is vital for reliable annotation.
Understanding Data Annotation Services
Data annotation services are key to the success of machine learning data annotation. They transform raw data into formats AI can understand. By labeling data, these services prepare AI models to recognize patterns and make accurate predictions.
Definition and Importance
Data annotation labels data, including text, audio, images, and videos, for AI model training. High-quality annotations are vital for AI model accuracy and performance. The demand for these services has skyrocketed in the last 5-10 years, driven by AI and machine learning's widespread use. Annotated data helps AI models recognize patterns, benefiting healthcare, automotive, and e-commerce.
Key Types of Data Annotation
There are several essential types of data annotation for AI applications:
- Image Annotation: Adds captions, identifiers, and keywords to images, vital for facial recognition, computer vision, and robotic vision.
- Object Recognition/Detection: Labels specific objects in images, enabling AI to recognize objects in real-world scenarios.
- Text Annotation: Includes semantic, intent, sentiment, and entity annotation, refining text data for sentiment analysis and language processing.
- Audio Annotation: Timestamps and labels verbal cues, aiding algorithms in understanding language, emotions, and behavior.
- Video Annotation: Captures key video points, teaching AI concepts like motion blur and object tracking.
The data labeling process includes data collection, preprocessing, quality assurance, and data export. Effective dataset management and advanced annotation techniques enhance annotation efficiency. Techniques like bounding boxes, polygons, keyphrases, and sentiment labels are critical.
Industries Utilizing Data Annotation Services
Data annotation services are vital across various sectors, revolutionizing operations and outcomes. These services are essential for industries to enhance their AI applications and achieve superior results. We explore three key sectors that greatly benefit from data annotation.
Healthcare
In healthcare, healthcare data annotation is critical for applications like cancer screening, patient diagnosis, and drug development. Accurate annotation of medical images trains AI to detect diseases early, improving patient care and diagnosis speed. It's also vital in drug discovery, where annotated data predicts a compound's efficacy and side effects.
Automotive
The automotive sector relies on automotive AI solutions powered by data annotation for autonomous driving advancements. Annotated images and videos train AI models to recognize road signs, pedestrians, and other vehicles. This speeds up the development of self-driving cars, making transportation safer and more efficient. Annotation also supports predictive maintenance, identifying vehicle failures before they happen.
E-commerce
In e-commerce, e-commerce AI applications use annotated data to personalize shopping experiences and improve customer interactions. Accurate tagging of product images and customer behavior data enables algorithms to suggest products based on user preferences. Annotated datasets also enhance chatbots and virtual assistants, leading to better customer service. By leveraging data annotation in industry, e-commerce platforms can optimize operations and boost user engagement.
Benefits of Professional Data Annotation Services
Professional data annotation services bring several key advantages to the table. They are vital for the success of machine learning models. By using these services, businesses can achieve higher accuracy and efficiency. They also gain the benefit of scalable options.
Accuracy and Efficiency
The quality of data annotation directly affects AI and machine learning model performance. Industry statistics show that 70-80% of AI or ML models fail due to subpar training data. Professional data annotation services address this issue by delivering accurate and high-quality data labeling, tagging, or categorizing for AI or ML projects.
- Improved Accuracy: High-quality data annotation enhances the reliability of ML models, boosting overall efficiency and effectiveness.
- Reducing Bias: Inaccurate or incomplete data annotation can lead to biased ML models with severe consequences. Professional annotation services help minimize labeling bias, ensuring more precise predictions.
Investing in top-notch data annotation services saves businesses time and resources. It allows teams to concentrate on more critical tasks like model optimization and strategy development. This approach to data annotation reduces the need for rework and corrections, saving both time and money in the long run.
Scalability
Professional data annotation services also offer scalability benefits. Handling growing volumes of data effectively is essential for expanding businesses. Collaborating with a specialized service provider enables companies to adjust resources as needed without sacrificing output quality.
- Flexible Resource Allocation: Outsourcing these services offers the flexibility to scale up or down based on project demands, avoiding the limitations of fixed internal capacities.
- Cost-Effectiveness: Compared to training internal staff or investing in expensive annotation tools, outsourcing is often more cost-effective. It provides access to expertise and scalable data annotation services.
Common issues like human error or unclear instructions become easier to manage when businesses can scale resources and expertise on-demand. Given the resource-intensive and time-consuming nature of providing quality data at scale, outsourcing is a strategic advantage. It helps companies streamline their data annotation processes while maintaining high standards of quality and accuracy.
Common Data Annotation Techniques
In the world of data annotation, various methods are used to prepare data for machine learning models. These techniques are essential for sectors aiming to use AI, providing precise insights. Key methods include image and video annotations, text annotations, and audio annotations. Let's explore each.
Image and Video Annotation
Image annotation tools are vital for computer vision, enabling systems to understand visual data. By labeling images, models can categorize and identify objects. Techniques like bounding boxes are key for object detection, pinpointing object locations in images.
Semantic segmentation labels every pixel in an image, distinguishing between different classes. Instance segmentation differentiates between objects of the same class. Keypoint annotation focuses on specific points for precise measurements. Polygon annotation delineates objects with clear shapes, aiding in accurate object recognition in complex backgrounds.
Text Annotation
Text annotation methods are critical in natural language processing (NLP). They involve tagging and labeling text data to train models for language understanding. This is essential for applications like chatbots or translation services.
Annotation tasks include identifying parts of speech, named entities, sentiment, or intent behind a user query. This structured labeling is vital for developing models that can comprehend and interact using human language. It pushes the boundaries of what's possible with NLP.
Audio Annotation
Audio data annotation is key for developing speech recognition and voice-activated systems. It involves labeling components within sound files, such as spoken words, intonations, or background noises.
Such annotations are critical for training models to accurately transcribe and understand spoken language. From creating voice user interfaces to improving automated customer service systems, reliable audio annotation is essential. It underpins the effectiveness of these advanced solutions.
Technique | Description | Application |
---|---|---|
Image Labeling | Assigning tags to complete images for categorization | Basic image classification |
Bounding Boxes | Drawing boxes around objects to specify location | Object detection models |
Semantic Segmentation | Labeling every pixel in an image according to class | Classifying regions in images |
Instance Segmentation | Differentiating between objects of the same class | Detailed object annotation |
Keypoint Annotation | Marking specific points on objects | Facial recognition |
Polygon Annotation | Delineating objects with clear paths or shapes | Detailed object recognition |
Understanding and applying the right data annotation techniques is vital for developing high-performing AI models. By using advanced video annotation techniques, text annotation methods, audio data annotation practices, and expert image annotation tools, industries can significantly improve the accuracy and reliability of their AI solutions.
Choosing the Right Data Annotation Provider
In the rapidly expanding field of AI development, choosing a data annotation provider is critical. It can significantly impact your project's success. Before making a decision, assess various factors. This ensures consistent data annotation quality and leverages the expertise of experienced data annotators.
Factors to Consider
When choosing a data annotation provider, several critical aspects must be considered. Key elements to not overlook include:
- Accuracy Rates: Inquire about the provider's accuracy metrics and their quality assurance processes.
- Security Protocols: Ensure the provider complies with international standards such as ISO 27001.
- Turnaround Times: Evaluate their ability to meet your project deadlines.
- Scalability and Flexibility: The provider should manage large volumes efficiently without compromising data annotation quality.
- Transparent Pricing: Focus on value and check for any hidden costs.
- Industry Knowledge: Opt for providers with a track record in your specific industry.
- Quality Control Measures: Ensure robust quality control processes are in place.
- Technology and Tools: Understand the technology and tools used, including AI integration.
- Data Security and Privacy: Ensure the provider agrees to sign NDAs and has clear data handling policies.
- Customer Support: Verify they offer responsive support throughout the project lifecycle.
- Regular Updates: The provider should keep you informed about progress and address challenges promptly.
Evaluating Quality and Experience
Evaluating the data annotation quality and experience of a provider is essential. Look at their project portfolio and experience with similar data types and complexities. High-quality data annotation services include multi-tier quality checks, ensuring an accuracy rate of over 95%. This is vital for building reliable AI models.
Another critical aspect is the expertise of experienced data annotators. They must understand the nuanced requirements of your industry. Outsourcing data annotation to professionals can result in a 50% quicker turnaround time and up to a 40% error reduction compared to internal teams. This significantly fuels the efficiency of your AI projects.
Lastly, consider the cost-benefit aspect. Outsourcing data annotation services can save up to 60% in overall project costs while producing a consistently high-quality dataset. Professional annotation services lower overhead costs by up to 40% compared to establishing an internal annotation team. This makes them a cost-effective choice for scalability and timely project completion.
The Role of AI in Data Annotation
In our increasingly data-driven world, the role of AI in data annotation is becoming ever more critical. This transformation is revolutionizing various industries by significantly increasing the speed and volume of data processed. It directly impacts the accuracy and efficiency of machine learning models.
Automation Impact
Annotation automation uses advanced algorithms to manage large datasets efficiently. Automated tools not only speed up the process but also reduce human errors. These errors are often critical in high-stakes environments like healthcare.
For example, accurate medical imaging annotations are essential for boosting disease detection rates. Automation significantly aids in achieving this precision. By automating repetitive tasks, AI allows human experts to focus on more complex annotation challenges. This improves overall project outcomes.
Human Oversight
Despite the impressive capabilities of automation, human oversight in AI remains indispensable to ensure quality and accuracy. Human annotators bring invaluable expertise and contextual understanding that machines currently lack. Transparency and privacy considerations in data handling are also best managed with substantial human involvement.
Collaborative efforts between academia and tech companies drive advancements in AI annotation techniques. This further optimizes the process. The integration of human oversight ensures that the final annotated datasets meet high standards. This enhances the reliability of AI models used in various applications.
Challenges in Data Annotation Services
The success of machine learning models heavily relies on the quality of their training data. Ensuring data quality while managing vast amounts of information is a major challenge. The accuracy of data annotation directly impacts the performance and reliability of AI and ML applications.
Ensuring Data Quality
Data quality assurance is critical in the annotation process. Poor quality data can severely hinder the performance of machine learning models. Key challenges include:
- Consistency Across Annotations: Maintaining uniformity across large datasets is imperative to avoid biases.
- Qualified Annotators: The scarcity of skilled annotators can lead to inconsistent and inaccurate labeling. Businesses must ensure that their annotators possess domain knowledge to reduce errors.
- Privacy and Security Concerns: Handling sensitive information necessitates stringent privacy policies and security measures to prevent data breaches.
Managing Large Datasets
Managing big data in annotation tasks presents another primary challenge. As data complexity and volumes increase, quality consistency drops due to annotator fatigue, and lack of adequate tools can further disrupt project timelines. ML and AI models require copious amounts of accurately labeled data, demanding a substantial workforce:
- Infrastructure Investment: High-quality data annotation necessitates advanced tools and technologies, resulting in significant capital expenditure.
- Annotation Tools: Domain-specific and multi-modal datasets require sophisticated annotation tools to tackle complex tasks.
- Human Resource Management: A large team of annotators is essential for handling extensive datasets, which adds layers of complexity in staffing, training, and management.
Platforms like Keylabs offer efficient solutions with robust quality assurance frameworks, secure data handling measures, and skilled annotators ensuring superior data quality. They provide end-to-end support for various annotation demands, helping organizations maintain balance amidst growing complexities.
Challenge | Description | Solution |
---|---|---|
Consistency Across Annotations | Maintaining uniform labeling standards across extensive data | Employing trained, domain-specific annotators and regular quality checks |
Qualified Annotators | Scarcity of skilled professionals leading to errors | Outsourcing to specialized firms with experienced annotators |
Privacy and Security Concerns | Risk of data breaches and compliance issues | Adopting stringent privacy policies and utilizing secure annotation platforms |
Infrastructure Investment | High costs associated with cutting-edge annotation tools | Leveraging affordable, scalable solutions from established platforms |
Annotation Tools | Need for sophisticated tools to handle complex and multi-modal data | Using advanced platforms |
Human Resource Management | Challenges in managing a large workforce for extensive datasets | Implementing effective project management systems and ongoing training |
Cost Considerations for Data Annotation Services
Investing in data annotation services requires a grasp of different pricing models and budgeting strategies. This knowledge helps businesses allocate resources wisely, ensuring high-quality annotations without overspending.
Pricing Models
Several data annotation pricing models exist:
- Per-Hour Pricing: This model charges by the hour, ideal for tasks with unpredictable time requirements.
- Per-Project Pricing: A fixed cost for the entire project, suitable for projects with clear timelines and requirements.
- Subscription-Based Pricing: A recurring fee for ongoing data annotation services, beneficial for long-term projects.
Budgeting for Annotation Projects
Effective budgeting for data annotation is essential for managing costs. Key considerations include:
- Data Complexity: More complex data requires detailed and precise annotation, affecting the cost. For instance, semantic segmentation—a highly complex task—can cost around $0.84 per unit.
- Annotation Task Type: Costs vary by annotation type. Bounding boxes are priced at approximately $0.035 per unit, while keypoint annotation starts at around $0.20 or less per task.
- Volume of Data: Larger datasets often benefit from economies of scale, potentially lowering the cost per unit.
Task Type | Approximate Cost per Unit |
---|---|
Bounding Boxes | $0.035 |
Polygons | $0.036 |
Semantic Segmentation | $0.84 |
Keypoint Annotation | $0.20 |
Understanding these cost factors and statistics enables businesses to make informed decisions and manage their data annotation budgets effectively. Adopting best practices such as clear annotation guidelines and consistent communication can also optimize efficiency and reduce costs.
Evolving Technologies
Emerging technologies like Generative AI and synthetic data generation are reshaping data annotation. Generative models, such as GPT and GANs, are key tools for labeling data and creating synthetic datasets. They are beneficial in automotive and robotics, providing cost-effective and rapid training for AI models. Grand View Research forecasts a 40.4% CAGR for the NLP market, reaching $439.85 billion by 2030. This highlights the importance of evolving annotation technologies in AI and data services.
Integration with Machine Learning
Integrating ML with data annotation enhances AI systems' efficiency and sophistication. Techniques like multimodal data annotation, which annotate text, images, audio, and video together, are becoming popular. These methods create versatile models for different industries. In healthcare, accurate annotation of medical images is vital for detecting anomalies like tumors, improving diagnostic accuracy.
Retail companies use personalized data annotation to predict customer behavior, improving shopping experiences and sales. The rise of high-quality annotation standards, influenced by healthcare and finance, ensures rigorous validation and testing. This is critical for minimizing errors. Ethical considerations and data privacy during annotation are also becoming more important to avoid biases and comply with regulations like GDPR. As we move forward, integrating ML and evolving technologies will be essential for meeting the demand for high-quality, efficiently labeled datasets.
FAQ
What are data annotation services?
Data annotation services label data to make it understandable for AI models. They transform raw data into structured datasets for AI processing.
Why is data annotation important for AI and machine learning?
Quality training data is essential for AI and machine learning success. Data annotation structures data, improving algorithm efficiency and accuracy. This makes AI applications more reliable and effective.
What are the key types of data annotation?
Key types include image and video, text, and audio annotation. These support AI applications like autonomous driving and automated customer service.
How do different industries utilize data annotation services?
Data annotation is vital in various sectors: - Healthcare: For patient diagnosis and treatment plans. - Automotive: For autonomous vehicles. - E-commerce: For personalized shopping experiences and chatbots.
What are the benefits of professional data annotation services?
Professional services improve AI model accuracy and efficiency. They ensure reliability and scalability, handling large data volumes without quality compromise.
What are the common techniques used in data annotation?
Techniques include: - Image and Video Annotation: Frame-by-frame tagging for object identification. - Text Annotation: Handling natural language for chatbots and translation. - Audio Annotation: Labeling sound files for voice-activated assistants.
What factors should be considered when choosing a data annotation provider?
Consider their technology, security, and data privacy standards. Also, evaluate their track record and customer reviews for quality and experience.
How does AI impact the data annotation process?
AI automates annotation, increasing data processing speed and volume. Yet, human oversight is critical for quality and accuracy.
What challenges are commonly faced in data annotation services?
Challenges include maintaining data quality and consistency. Effective management involves sophisticated systems and continuous annotator training.
What are the pricing models for data annotation services?
Models include per-hour, per-project, and subscription-based. These help businesses budget effectively, ensuring quality without cost compromise.
What are the upcoming trends in data annotation?
AI advancements and machine learning integration will improve annotation tools. These trends will lead to more efficient AI systems and enhanced data annotation capabilities.
Comments ()