Custom Video Annotation Services for Actionable AI Insights
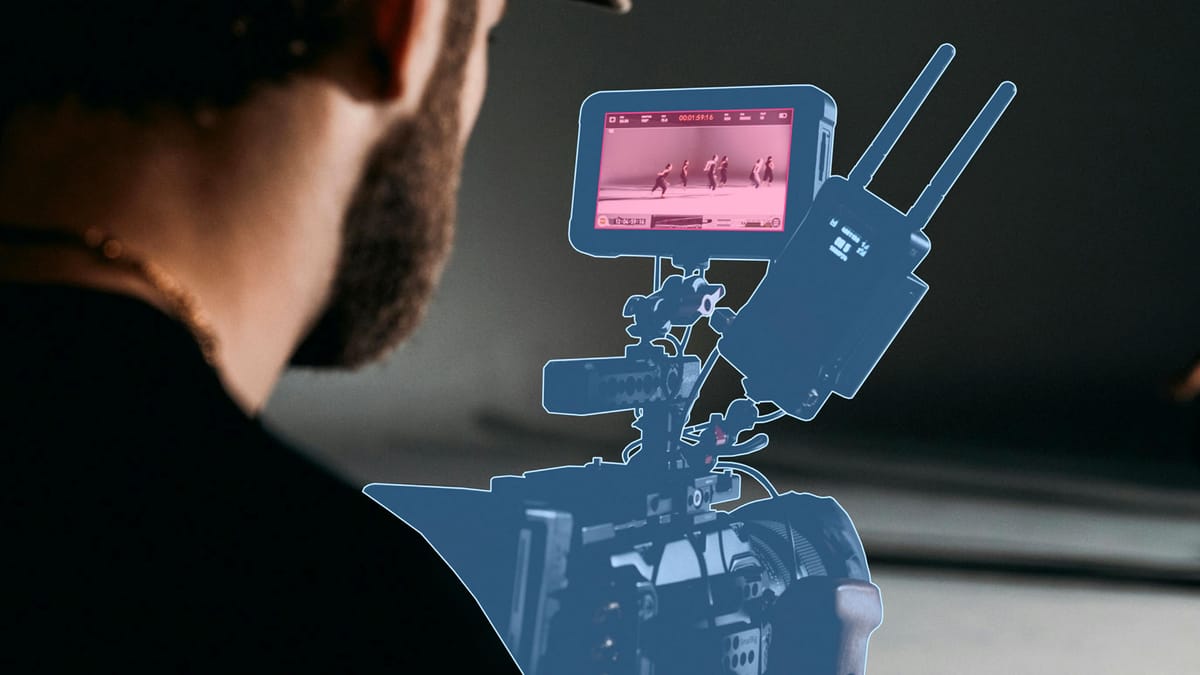
Video annotation is vital for refining AI systems. It involves tagging and labeling video content objects, actions, and scenarios. By employing various annotation methods, we improve AI model training. This ensures efficient labeling of vast data sets.
These methods are critical for self-driving vehicles, robotics, healthcare, and more. They support a wide range of industries. Scalable video annotation services deliver high-quality annotations, catering to startups, SMBs, and large enterprises. Ongoing annotation efforts enable AI systems to learn from updated data, leading to enhanced performance and results.
Key Takeaways
- Custom video annotation services enhance AI system precision and effectiveness.
- 40% of FAANG companies rely on industry-leading data powered by video annotation.
- Key techniques include 2D bounding boxes, 3D cuboid annotation, semantic segmentation, and keypoint annotation.
- Video annotation supports diverse industries, including autonomous vehicles and healthcare.
- Scalable services are tailored to meet the needs of different business sizes.
Understanding Video Annotation: An Overview
Video annotation is key for creating advanced AI, focusing on computer vision. It involves tagging video frames with structured data. This process boosts AI and machine learning model training. It's vital for extracting actionable insights and improving computer vision systems.
What is Video Annotation?
The term video annotation definition means adding labels to video content. It includes identifying and tagging objects, actions, or elements in each frame. This labeling transforms raw video data into insights, essential for AI model training.
Importance of Video Annotation for AI Models
Video annotation is critical for AI and machine learning. It acts as the 'ground truth' for AI model training. Accurate annotations provide context for object movements, improving tracking and detection.
It captures object continuity and interaction, vital for robust computer vision models. These models can analyze visual data for decisions, like detecting anomalies in medical imaging or improving autonomous vehicles.
The need for quality video annotation services is increasing. Driven by industries like agriculture, retail, healthcare, and entertainment. Accurate video metadata through precise annotations is essential for advancing AI and model performance.
Types of Video Annotation Techniques
In the realm of AI-driven industries, the variety of video annotation techniques is key. From object detection to keypoint annotation, these methods boost AI model capabilities. Let's explore the different annotation techniques and their importance.
Object Detection Annotation
Object detection annotation identifies and tracks objects in video frames over time. It's vital in areas like autonomous vehicles, where knowing object locations is key for navigation and decision-making. Companies like SunTec.AI specialize in creating top-notch datasets for accurate object detection.
Semantic Segmentation
Semantic segmentation classifies every pixel in video frames, providing a detailed analysis. It's highly beneficial in healthcare, used to annotate medical videos like ultrasounds. This enhances educational resources for medical students, leading to deeper insights for AI applications.
Instance Segmentation
Instance segmentation distinguishes between individual objects of the same class in a video. This precision is essential in retail for inventory management and analyzing customer behavior. It helps businesses understand stock levels and customer trends, guiding marketing strategies.
Keypoint Annotation
Keypoint annotation defines specific points within an object to understand its posture and movement. It's critical in sports analytics for evaluating athlete performance and in healthcare for analyzing patient movement. Human annotators use this to teach machines to accurately interpret complex motions.
Techniques like object detection, semantic segmentation, instance segmentation, and keypoint annotation are vital for developing advanced AI models. Tailored to specific needs, these methods ensure the creation of precise and high-quality datasets.
Applications of Video Annotation in Various Industries
Video annotation applications are transforming multiple industries. They bring precision and detail to various technological and operational processes. This includes autonomous driving, medical treatments, and performance analytics. Let's explore how these applications are making a significant impact in different sectors.
Autonomous Vehicles
In autonomous driving, video annotation is key for training machine learning algorithms. It helps identify and categorize objects like pedestrians, traffic signals, and vehicles. This detailed labeling enhances safety and reliability on the road.
For more on how video annotation supports autonomous driving, check out this detailed guide.
Healthcare Innovations
In healthcare, video annotation is vital. It's used to train AI models for medical imaging. This aids in detecting anomalies, classifying diseases, and predicting outcomes.
It also improves the precision of robotic surgeries and patient care. Annotated videos help recognize surgical patterns, leading to better techniques and outcomes. For more on video annotation in medical imaging, see this resource.
Sports Analytics
Sports analytics benefit greatly from video annotation. It helps analyze player performance, game tactics, and opponent strategies. AI systems can predict injury risks, suggest strategies, and identify areas for improvement.
This enhances player training and game outcomes. With the global market for data annotation tools expected to hit $3 billion by 2028, the demand for video annotation in sports analytics will grow.
These diverse applications highlight the versatility and importance of video annotation. It drives innovation, efficiency, and improved outcomes across various industries through actionable AI insights.
Custom Video Annotation Services: Why They Matter
Custom video annotation services are vital for businesses aiming to leverage AI's full power. They provide customized annotation solutions tailored to various industries. This ensures high adaptability and precision.
Tailored Solutions for Unique Needs
Each industry has unique video annotation needs. Our services offer tailored AI solutions to meet these specific demands. For instance:
- Autonomous vehicles use annotated videos to spot road signs, pedestrians, and lanes.
- In healthcare, video annotation helps in disease identification and surgical guidance.
- The retail sector benefits from video annotation for checkout-free stores and personalized product suggestions.
We use advanced tools for complex tasks like facial recognition and action detection. This improves AI project quality and efficiency. Our services also annotate visible objects for localization, critical in video analysis.
Enhancing Data Quality and Relevance
High data accuracy is key for AI model success. Our customized solutions ensure consistency and precision through continuous frame methods. Techniques like optical flow capture frame-by-frame changes, maintaining context and continuity. This supports better object tracking and labeling across frames.
Annotation Technique | Application |
---|---|
Bounding Boxes | Object detection in computer vision |
Polygon Annotation | Identifying object edges |
3D Cuboid Annotation | Object depth and dimensions identification |
Keypoint Annotation | Highlighting object variations |
Polyline Annotation | Balanced entity labeling |
Semantic Segmentation | Image segmentation in videos |
Our commitment to high data accuracy and tailored AI solutions boosts annotated data relevance. This directly influences AI project performance and success.
Choosing the Right Video Annotation Service Provider
When selecting a video annotation service, it's vital to consider several factors. This ensures your project's needs and goals are met. Evaluating the provider's expertise and conducting a thorough service evaluation significantly impacts your AI model's quality and performance. Here are key aspects to consider.
Key Factors to Consider
Choosing the right video annotation service requires understanding the provider's capabilities and resources. Several key factors should be evaluated:
- Technological Capabilities: Check if the service provider uses advanced tools and software compatible with popular machine learning frameworks like TensorFlow and PyTorch.
- Industry Expertise: Ensure the provider has experience in your specific industry. This expertise can enhance annotation relevance and accuracy.
- Scalability: Assess the provider's ability to scale services to meet project demands. Providers like Labellerr claim to be ten times more efficient in labeling video datasets compared to others.
- Turnaround Time: Rapid turnaround times are critical, even for large datasets. Evaluate the provider's delivery schedules and reliability.
- Data Security: Consider the security measures in place to protect data accuracy and privacy. Features like secure file access and restricted viewing rights are essential.
The Impact of Video Annotation on AI Performance
Video annotation is key to boosting AI performance by providing top-notch, well-labeled training data. This makes AI models more dependable and efficient in real-world use, critical for machine learning projects.
Improving Model Accuracy
Video annotation significantly boosts model accuracy. AI models need vast amounts of precisely labeled data to work right. For example, Waymo, a pioneer in self-driving cars, uses video annotation to tag objects like vehicles, pedestrians, and road signs. This increases model accuracy.
By consistently applying accurate annotations, AI models learn from high-quality data, improving their performance.
Diverse annotation methods, like bounding boxes, polygonal segmentation, and keypoint annotation, are vital. They mark detailed information across frames. These methods, used with care, are essential for building a strong dataset. This dataset enhances the learning process and AI performance.
Reducing Error Rates
Video annotation also plays a significant role in reducing AI errors. High-quality annotations cut down on misunderstandings and misinterpretations by AI models, leading to fewer mistakes. For instance, Deep Sentinel uses video annotation to train their AI to spot security threats accurately, lowering false alarm rates.
Effective machine learning heavily relies on error reduction, and video annotation is key to achieving this. Technologies like continuous frame methods, such as optical flow, ensure consistent object tracking. This eliminates human bias and maintains high accuracy standards across large datasets. Outsourcing annotation tasks to expert providers also boosts annotation quality and reliability.
In summary, video annotation greatly enhances AI performance and the success of machine learning in various sectors by ensuring high model accuracy and reducing errors.
Technology in Video Annotation
The evolution of video annotation technology has revolutionized AI model development. Through the use of automation tools and advanced annotation software, we can annotate videos quickly and accurately. This makes the process not only efficient but also reliable.
Automation in Video Annotation Processes
Automation is key in simplifying video annotation tasks. Machine learning and deep learning models automate the labeling of video clips, cutting down manual effort. Automatic video labeling, for instance, categorizes sequences using pre-trained models. This speeds up the annotation process while maintaining high accuracy.
Techniques like interpolation are used to create synthesized video data between existing frames. This improves the quality of video datasets and adds to existing frames, making datasets more complete. Methods like optical flow estimation, adaptive separable convolution, and Deep Voxel Flow are essential in these processes. They ensure smoother and more accurate frame transitions.
Tools and Software Used
The variety of tools and software for video annotation is vast and powerful. We use a mix of proprietary solutions and industry-standard platforms to meet project-specific needs. Key tools include:
- Bounding Boxes: Essential for object detection, enabling the identification and localization of objects within frames.
- Polygon Annotation: Used for annotating objects with complex shapes, making it invaluable in diverse industries like medical imaging and security surveillance.
- Keypoint Annotation: Facilitates the marking of specific points of interest, useful in pose estimation and action recognition applications.
- Manual and Auto-Annotation Software: Combines manual precision with automated efficiency, scaling annotation tasks without quality loss.
These annotation software solutions offer the precision needed for high-quality datasets. They also adapt to various project needs, ensuring versatility across fields like transportation, healthcare, and retail.
Best Practices for Effective Video Annotation
Effective video annotation is key for training accurate AI models. It requires a detailed approach to ensure top-notch data quality. By following video annotation best practices, we can boost both the reliability and efficiency of our AI solutions.
Ensuring Consistency
Consistency in annotation is vital for creating reliable datasets. It involves setting up standardized guidelines to reduce variability in annotations. This is critical for achieving consistent and accurate results from different annotators.
- Create detailed annotation guidelines.
- Use pre-defined annotation schemas.
- Hold regular training sessions for annotators.
- Use automated tools for ongoing quality checks.
Collaborating with Annotators
Annotator collaboration is another essential aspect of video annotation. A collaborative environment ensures all annotators are aligned, leading to better data quality.
- Encourage open communication and feedback loops.
- Use collaborative tools for real-time updates and corrections.
- Regularly review and discuss challenging cases with the team.
- Utilize tools like shared dashboards and project management software.
Adopting these video annotation best practices will not only improve data quality but also streamline the annotation process. This will positively impact AI model performance. Here’s a quick comparison of different annotation techniques:
Technique | Description | Common Uses |
---|---|---|
Bounding Boxes | Rectangular boxes are used to delineate objects in video frames. | Cars, pets, buildings |
Polygons | Flexible shapes used for annotating irregular objects. | Complex shapes, irregular objects |
Keypoint Annotations | Points used for tracking moving objects within video data. | Human and animal movement |
By incorporating these practices, we can ensure our video annotation projects produce high-quality datasets. This will help reduce error rates and enhance the accuracy of our AI models.
Challenges in Video Annotation
Video annotation faces several challenges, including managing vast video data volumes and addressing subjective annotation practices. Overcoming these hurdles is essential for high-quality AI model training.
Managing Large Volumes of Video Data
Data volume management is a major challenge in video annotation. Robust systems are necessary for processing, storing, and retrieving large video datasets. This is critical in multi-modal annotation, which combines video with audio, LiDAR, and GPS for richer context.
In sectors like autonomous vehicles, effective data management ensures accurate annotations. Privacy-preserving techniques are also emerging to address sensitive data concerns in healthcare, finance, and law enforcement.
Addressing Subjectivity in Annotation
To reduce subjectivity, the annotation process must be refined to minimize human bias. This is vital for maintaining data accuracy in AI models. Training annotators to be objective and consistent is key. Quality control and assurance are becoming more important, ensuring data reliability in healthcare and aerospace.
Semi-supervised learning and federated learning are emerging trends. Semi-supervised learning combines labeled and unlabeled data, aiding industries with limited annotated data, like agriculture. Federated learning enables collaborative annotation and model training without centralized data, benefiting medical research and climate monitoring.
Challenge | Solution | Application |
---|---|---|
Video Annotation Challenges | Robust Data Management Systems | AI Model Training |
Data Volume Management | Efficient Data Processing | Autonomous Vehicles |
Reducing Subjectivity | Training Annotators | Healthcare, Aerospace |
Stringent quality control ensures annotated frames are accurate and reliable. Popular annotation types include 2D bounding boxes for object detection and 3D bounding boxes for detailed object depiction. Structured annotation processes enhance computer vision model training, leading to more precise AI insights.
Future Trends in Video Annotation
The future of video annotation is rapidly evolving, driven by significant advancements in AI and the integration of real-time analytics. These emerging technologies are set to transform the capabilities and applications of video annotation. It's essential for various industries to stay updated on these trends.
Advancements in AI and Machine Learning
Looking ahead, AI advancements play a key role in the future of video annotation. Applications in autonomous vehicles, surveillance systems, and video content analysis are becoming more sophisticated. Deep learning algorithms rely heavily on the quality and quantity of annotated data for their effectiveness.
Upcoming trends include the integration of artificial intelligence to automate annotation processes. This will enhance efficiency and accuracy. Collaborative annotation platforms are also gaining traction, enabling distributed teams to work on large-scale video datasets seamlessly.
The incorporation of semi-supervised learning techniques into these tools will allow models to learn from partially labeled datasets. Incorporating features that enhance the explainability and interpretability of deep learning models is also a significant trend. As the global data volume is projected to reach 175ZB by 2025, with 90% being unstructured data, the demand for high-quality annotation tools will only increase.
Integration with Real-time Analytics
The integration of real-time analytics with video annotation tools is a game-changer for many industries. This integration allows for real-time processing, essential for applications requiring immediate feedback and decision-making. Automatic annotation technology is vital for handling the escalating volume of data, transforming how data is used across various sectors.
The demand for stringent quality standards in data annotation is on the rise, with sectors like healthcare and aerospace requiring data accuracy exceeding 99%. The global medical imaging market is anticipated to expand at a CAGR of 6.4% from 2022 to 2030. The need for high-quality medical data annotation is projected to grow, as sectors like digital commerce and social media heavily rely on visual data processing.
Unlocking the Potential of Video Annotation
Video annotation turns passive viewing into interactive learning, fostering viewer engagement. This is vital in sectors like autonomous driving and healthcare. In these fields, annotations enable AI to learn from various scenarios, improving performance. This leads to data-driven decisions, optimizing business strategies and operations.
Strategic video annotation use unlocks AI's full business solution capabilities. By ensuring visual consistency, using tools like Adobe Premiere Pro, and optimizing for search engines, businesses can lead in a competitive market.
FAQ
What is Video Annotation?
Video annotation transforms raw video into a structured dataset. It involves tagging video frames with labels. This makes the content understandable and usable for AI and machine learning algorithms.
Why is Video Annotation important for AI models?
Video annotation provides 'ground truth' for AI model training. It ensures they can accurately interpret visual data. This enhances AI model accuracy and effectiveness.
What are the different techniques used in Video Annotation?
Various techniques include: - Object Detection: Identifying and tracking objects over time. - Semantic Segmentation: Classifying all pixels of similar objects. - Instance Segmentation: Distinguishing between individual objects of the same type. - Keypoint Annotation: Defining points of interest within an object for posture and movement analysis.
How is Video Annotation applied in different industries?
Video annotation applications span multiple industries: - Autonomous Vehicles: Real-time decision-making for safer navigation. - Healthcare: Supporting medical imaging and patient monitoring. - Sports Analytics: Enhancing team strategies and player performance through detailed motion analysis.
What makes custom video annotation services valuable?
Custom video annotation services offer tailored solutions. They align with unique project needs, ensuring high data accuracy and relevance. They provide scalability and adaptability for complex scenarios, improving the quality and efficiency of AI projects.
What factors should be considered when choosing a video annotation service provider?
Key factors include: - Technological capabilities - Expertise in relevant industries - Ability to scale services as needed - Data accuracy and security measures - Turnaround time
How does video annotation impact AI performance?
Video annotation significantly enhances model accuracy. It reduces error rates in machine learning projects by providing well-labeled, high-quality training data. This allows AI models to perform more reliably and effectively in practical applications.
What are the best practices for effective video annotation?
Ensuring consistency across multiple annotators and facilitating open collaboration are key. Standardizing annotation guidelines and fostering a collaborative environment help maintain high-quality data. This results in more accurate AI model training.
What challenges exist in video annotation?
Challenges include managing large volumes of video data and addressing subjectivity in annotation practices. Overcoming these involves implementing robust data management systems and training annotators to enhance objectivity. This ensures AI models are trained on well-curated and unbiased datasets.
Comments ()