Data Annotation for Machine Learning from A to Z
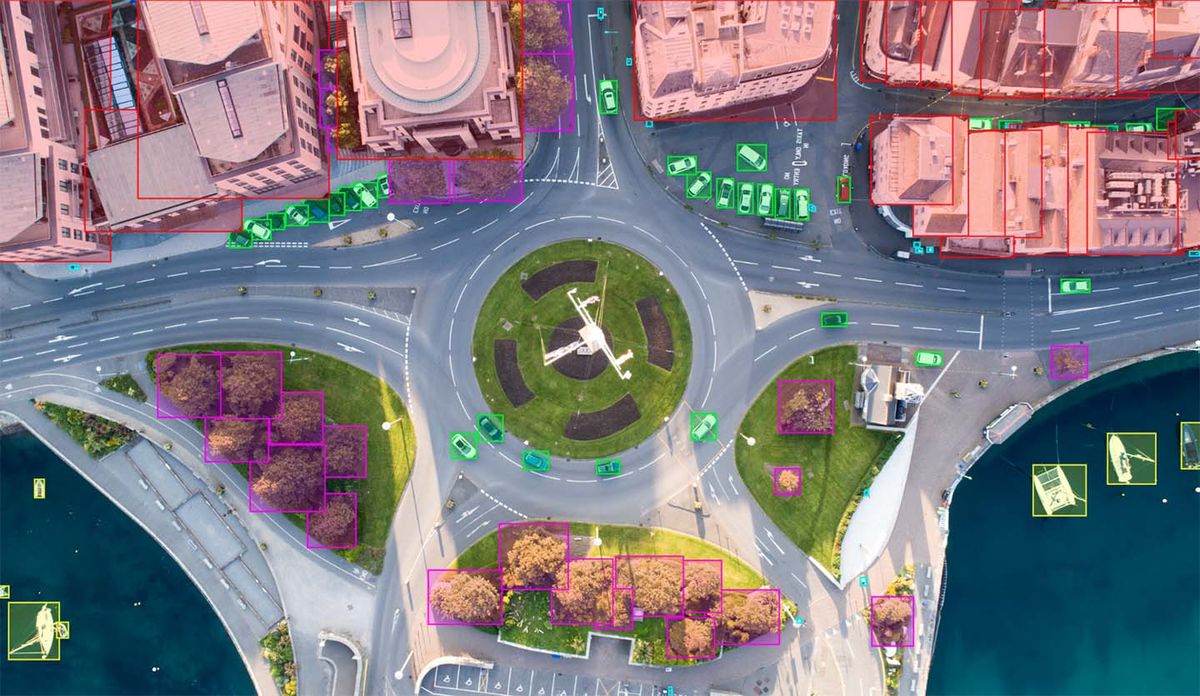
Companies use machine learning to train artificial intelligence. The algorithms used for machine learning need an immense amount of data that can take many forms. The most common kinds of data are video and images. Data can include any type of data that a computer can store.
Before that data is useful for machine learning, its users must label it, then tag and annotate it. That is due to the incredible amount of data that human beings must collect and annotate. This is why robust data annotation outsourcing services are needed.
That is still a job for human beings who work on a data annotation platform. The tremendous amount of data they need to train an AI can make it a challenge for companies to scale data annotation on their own. It can be a time intensive, tedious process that demands attention to detail.
It is also a task that valuable programmers and engineers waste much time and effort on, and they can not entirely automate it at the present time. A certain basic human intelligence and human eyes are still needed for primary object and pattern recognition. Data annotation for startups can be a challenge because then they need more employees and dedicated resources.
That is why outsourcing data annotation projects is a popular solution for companies developing innovative AI.
What is Data Annotation?
Data annotation is the tagging and labeling of data such as pictures and videos by human beings. That way, computer programs like machine learning algorithms can recognize the data that it needs to process and maintain the data.
The machine learning algorithm can then add the new information to build on its experience and better work to do things like making good decisions and more accurate predictions. That experience can even be suitable to transfer to other compatible AI algorithms.
There are different kinds of data annotation services for ml and all the various kinds of data.
Image annotation uses things like bounding boxes, polygons, cuboids, line skeletons, key points, and custom options. This ensures that the algorithm recognizes that an area of a picture contains a specific item and treats it that way. Captions, keywords, labels, and identifiers are additions that the ml algorithm can identify and understand their parameters and make use of this information. Semantic segmentation provides context.
Semantic segmentation assigns each pixel to a class of object. Instance semantic segmentation adds more detail by labeling every pixel belonging to the same class.
This type of data annotation is essential to use for autonomous vehicles and facial recognition, among other innovations.
Video Annotation is much like image annotation, only more so and more work. That is because a video is a stream of still images, sometimes with sound added, like any movie. It is a bit more complex and involves things like recognizing and tracking many different objects through many frames of video.
Video and image data annotation can include specialized images, like those from the James Webb Satellite Telescope. It is also used for medical data annotation, for example, MRI pictures used to diagnose injuries and diseases by doctors.
Finance data annotation involves labeling text, numbers, and other data to train AI to help with various tasks. These tasks can include:
- Risk Assessment
- Fraud Detection
- Regulatory Compliance
- Know Your Customers Laws
- Analyzing social media for public sentiment and trends
- Creating Chatbots
- Money making stock trading bots
Document data annotation is obviously related to Finance data annotation. It can be essential to train computer vision algorithms. They will learn how to recognize things like the issuer, phone numbers and addresses, dollar amounts, and due dates.
They will also face studying other common, critical information to speed up paperwork or its digital equivalent. Document and text data annotation is also vital for AI content moderation. Facebook is one the largest examples of the companies which are doing this.
The Future of Data Annotation
An estimated 500 exabytes of data created will need to store and annotate for various machine learning algorithms in the next 4 years. This is because AI algorithms can require billions of parameters. Furthermore, as these are still novel technologies, advance and progress are made in various industries. This means that ever more significant amounts of data will be required to process.
Larger and more diverse sample sizes and databases will help avoid bias and make for better artificial intelligence. These algorithms will be able to make more accurate predictions, with a greater degree of uncertainty known, and make better decisions.
As a result, data annotation will only become an exponentially larger job. Companies, especially exciting start-ups, will require the best data annotation outsourcing services possible.
Comments ()