Data annotation to get self-driving cars on the road
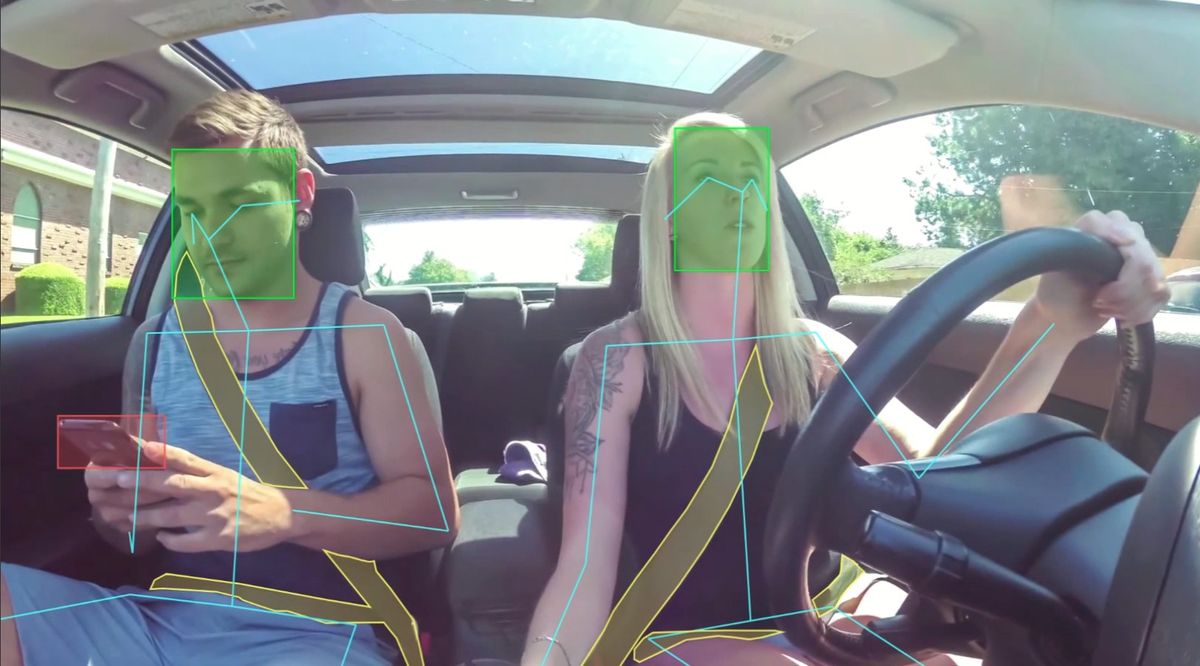
Self-driving cars have been the focus of technologists for a large part of the last two decades. It has featured heavily in science fiction and projections about the near future. In 2022, however, the self-driving revolution seems like it’s already old news. Major companies like Google’s Waymo and Tesla have brought the industry into the mainstream. The conversation should now shift to how self-driving cars actually work and the best practices for their development.
Data annotation is going to be the driving force behind success. With something like self-driving cars, a robust training data set can mean the difference between a functioning car and an accident waiting to happen. The best practices for this industry can vary significantly depending on the kind of data being collected. Knowing how these data sources are best addressed should be a focus from day one of your project.
What is the self-driving industry, exactly?
We most often think of the self-driving car itself when describing the industry. However, self-driving cars only compose a small part of a much larger entity. Self-driving cars are especially well-suited to act as members of larger fleets. Many have envisioned self-driving roads where only autonomous vehicles will be able to drive. These vehicles will be able to handle deliveries, taxi services, and a host of other yet unknown functions. Therefore, the needed dataset will not only pertain to the cars but systems of cars that can work together.
There are also many specific use cases for self-driving cars that will in some way incorporate data annotation. Many think these cars will operate in fleets. Many cars working together require different kinds of data to be annotated. Electric self-driving cars (which many consider the standard) will have to optimize self-charging for times of the day when energy is the cheapest. The data will be very heterogeneous and complex in each of these cases.
Lastly, safety must be a considerable focus of the self-driving vehicle industry. Cars are already heavily regulated by the government. They are fast, heavy, and have a huge potential for catastrophic failure. Training data will not only have to guide how cars are designed but also acknowledge these potential failures. This might appear in many different ways. In any case, having clearly annotated data will help prove that manufacturers have done their due diligence in designing their systems.
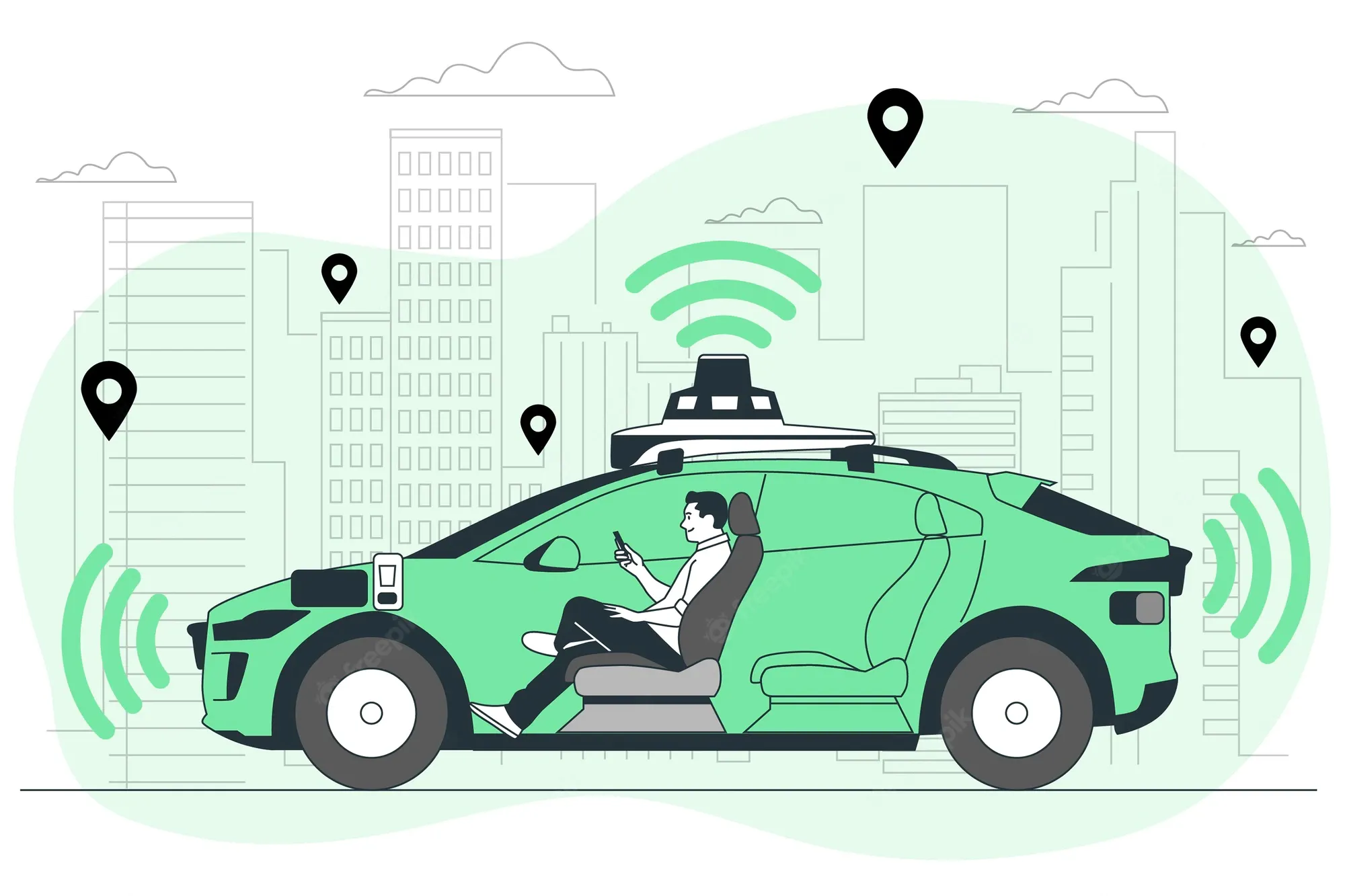
What kind of data will be annotated for self-driving cars?
As was mentioned, the kinds of data that might function as inputs to the “self-driving car industry” will come from a wide variety of sources and devices. The cars themselves will be data-analyzing and data-producing objects. This means that proper annotation will drive their ability to continue to function. The tools that will make this possible will have to be determined on a per-project basis.
The specific kinds of data can be bunched into a few broad categories. Each category will uniquely involve data annotation. Sources and uses of data also often overlap across these use cases. Some of these might include the following:
- Autonomous vehicle design/manufacturing
- Vehicle fleet dynamics
- People/supply chain dynamics
- Safety and regulatory controls
In the first situation, the application of data annotation is pretty clear. Self-driving vehicles use a considerable number of sensors to collect data about their surroundings. This includes things like radar and LiDAR, weather patterns, and camera data about surroundings.
Training datasets must correctly identify what these sensors are picking up and trigger the proper response in the vehicle. Categorizing these inputs is the first task of any autonomous vehicle manufacturer. This is also one of the most developed aspects of the industry right now.
Vehicle fleet dynamics takes those autonomous vehicles and adds one layer of abstraction. Instead of determining how one car operates, training data will be used to make predictions about how many cars will interact with each other. The goal of an effective fleet of vehicles would be to optimize how and where cars are operating.
This application is completely new, so training data will have to be annotated with specific end goals in mind. This would likely require a different use of the data already being collected by these vehicles and other kinds of sensors.
People and supply chain dynamics take the idea of the fleet even further. The vehicles will both work together and in response to external dynamics. Deliveries are a great example of this. Autonomous delivery vehicles could be trained on data surrounding when and where products are available to create the most optimized delivery path. Without human limitations constraining delivery times, this could completely revolutionize the speed of deliveries. The same could be said of vehicle fleets transporting people.
Safety and regulatory controls will use data about the safety and effectiveness of these vehicles to prevent catastrophic failure or accidents. This is critically important not only for safety but also for eventual mass adoption. Training datasets will need to define specifically the kinds of vehicle actions that lead to failures.
These data can then be implemented into the functions and practices of the cars themselves. Compared to the incredible danger of human-driven cars, this area alone could be one of the most prominent justifications for the transition to autonomous vehicles.
The state of the self-driving industry
As was mentioned before, the self-driving industry in 2022 is different than it has been in the past. We’re at a point where technology has all but been developed. Now it is the task of manufacturers to bring them into the mainstream. The use cases described above are just some of the applications of autonomous vehicles that people are discussing today. It’s possible to imagine uses in emergency response, agriculture, and other situations. Each of these areas will have its own training data requirements.
Data annotation is going to be a driving force behind whether these use cases ever come to fruition. If these cars do not work exceptionally well, naysayers will be able to paint them as dangerous. Change is hard, so technology often has to be more than just good. Effective training data from the start will help ensure that these projects are successful and inspiring. This will help mass fuel adoption in the future.
Comments ()