Data Annotation: Types and Use Cases for Machine Learning
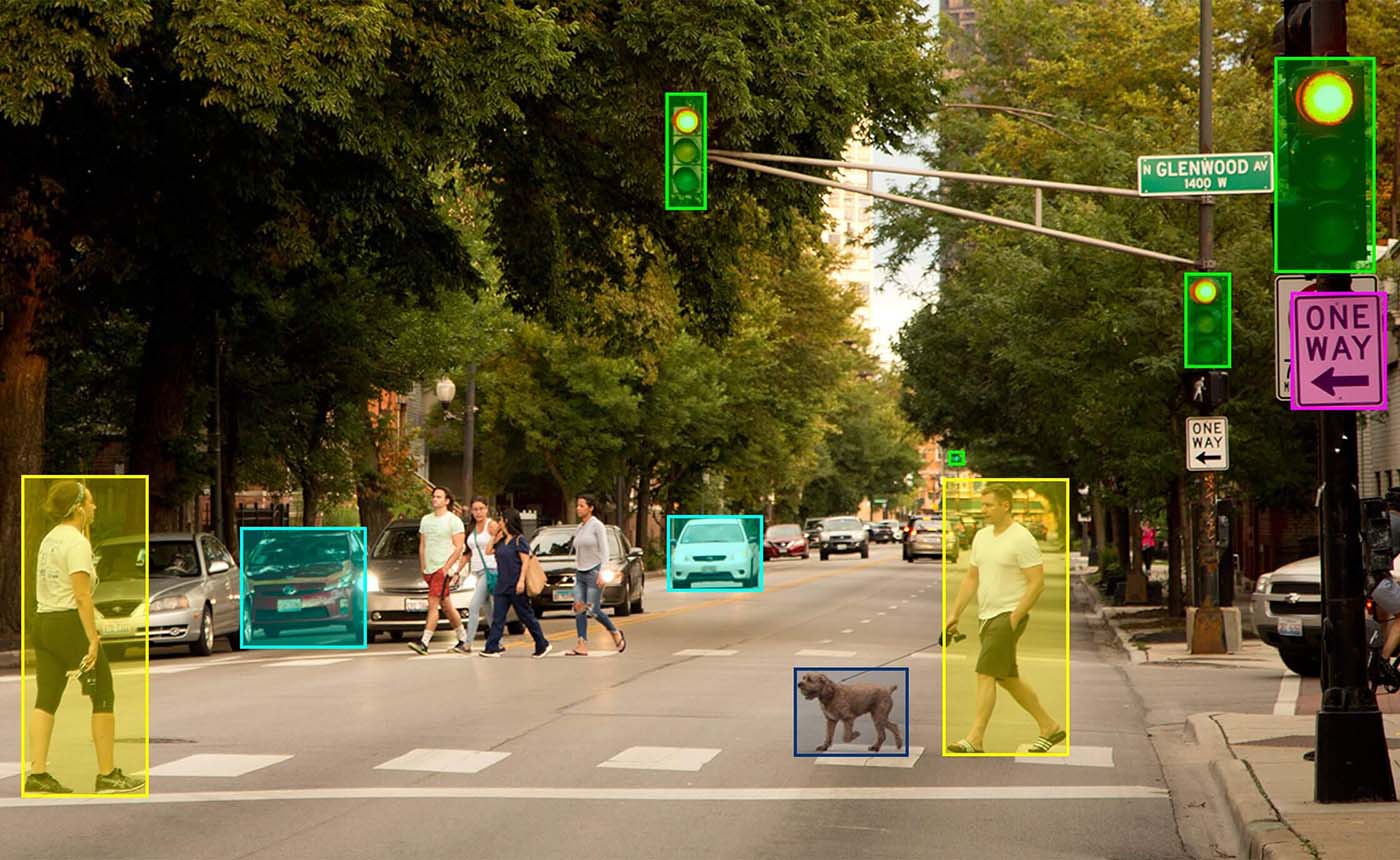
Machine learning is how AI is trained. It is different from regular computer programming used to create AI. For AI to be able to make use of data, humans must manually add even more metadata. That process is known as data annotation.
Just as many kinds of AI automate different kinds of expertise, there are many types of data annotation. No matter the type of data or AI, an awful lot of data is normally needed to reduce bias and increase accuracy. Therefore, expert data annotation services are required to automate expertise with machine learning.
For example, any project that uses computer vision will need human eyes to recognize and tag or label objects in pictures or videos. Text and documents often need extra metadata too. Semantic data annotation helps provide context to data and teaches the algorithm abstract concepts.
This all has a wide range of practical applications. For example, an autonomous vehicle project benefits from data annotation for self-driving cars. Insurance companies looking to use an algorithm to aid decision-making or computer vision to find facts would want insurance data annotation.
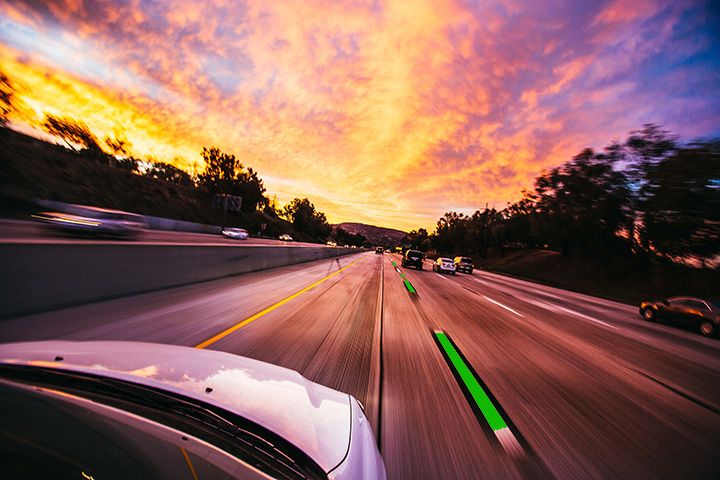
In creating the application, the end product is always an incredible feat. Behind those incredible products is a lot of work and moving parts. Machine learning often takes specialized hardware like neural networks. It also takes a large amount of data, even larger than measured in terabytes.
All that data needs the right metadata added with the right data annotation service to be useful. That is key to techniques like supervised learning. AI is sometimes not as trusted as it should be because humans can not always understand how the AI makes a decision.
The goal of machine learning is to create an AI that can make inferences. A machine that can learn and adapt. It shouldn’t always need explicit instructions for each step.
Deep learning is exciting. That is because deep learning and neural networks allow AI to extract higher functions from datasets. It is how emergent properties are formed. For example, consciousness is understood to be an emergent property. It could arise from sufficiently advanced causational programming.
In supervised learning, the machine learning algorithm is given all of the right answers. It then learns how to come up with the same correct answers. It also learns what is useful, relevant information, the signal, and what is not, the noise found in very large datasets. It is helpful for creating an AI that is more trusted by expert humans, like doctors.
Of course, what is helpful for one AI is not valid for another. There are many kinds of data and data annotation techniques that are used in many different exciting applications, products, and services.
Types of data annotation services and applications:
● Image Annotation
● Video Annotation
● Medical Data Annotation
● Financial Data Annotation
● Data Annotation for Self Driving Cars and Autonomous Vehicles
● Robotics Data Annotation
● Retail Data Annotation
Garbage and Data Collection
There are, of course, far too many use cases for AI, ML, robotics, and automation than can be mentioned in a single article. Waste Management is a growing problem and a growing field. Different kinds of waste may be usefully recycled. Other kinds present significant health hazards.
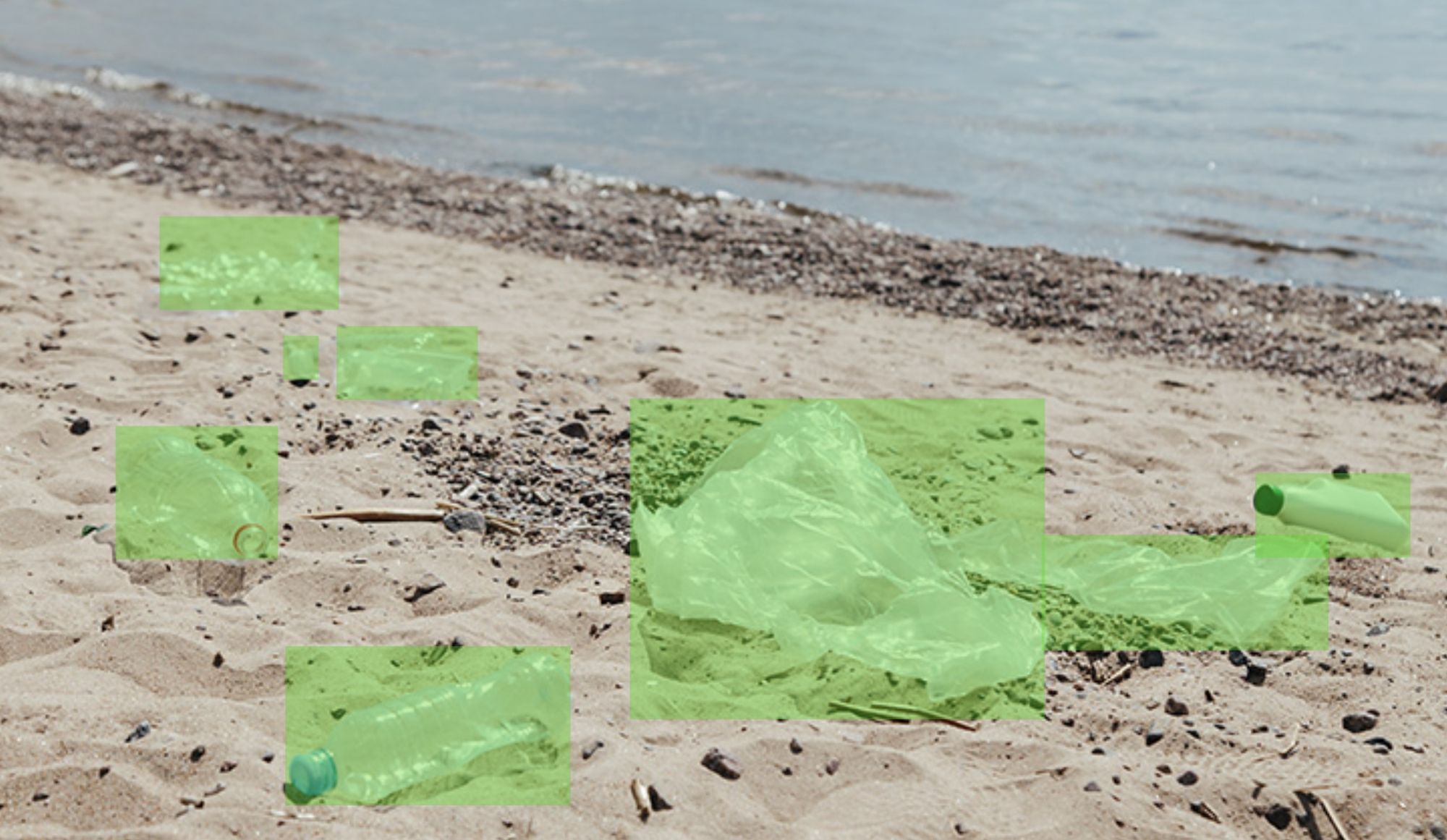
Machine vision with enough metadata behind it can recognize recycling markings, hazard markings, various materials, and all kinds of trash. Unfortunately, technology, industry, and societies in late-stage capitalism produce a tremendous amount of garbage.
All of the fast fashion bottles, cans, packages, and so forth that wind up being thrown away have to be sorted and recycled or disposed of properly. It is a respectable but dirty job that no human really wants to do, mainly because of the health risks.
Just as we create more products, we create more kinds of garbage. That means that over time any attempts to automate garbage collection, sorting, and recycling will constantly need more data annotation services. The environmental impact is also a serious problem. So is the sustainability of production. This is an industry that grows even faster than landfills.
Waste management is also an industry in which automation is highly desirable. The less humans handle hazardous and toxic waste, the better. Humans, at least in the USA, really don’t like sorting their garbage.
The Future of Data Annotation and Machine Learning
The future of data annotation and machine learning is bright. We live in societies that grow ever more complex. We live in a world with all manner of new things being invented and sold. Automation and capital will never entirely replace human labor. But, humans working with AI and using more complex tools is obviously the way forward. More specialized data annotation will be necessary as more specific data is added.
There are legal regulations and ethical standards regarding data collection and use in AI. Different AI products and services have different special needs. One need remains constant, and that is more data to increase the accuracy of AI. It is important to keep adding to the data used for machine learning.
The extra metadata generated by the human labor of data annotation companies provides depth and context. Machines are very "literal minded." Deep learning techniques take deep data at a large scale.
With all the complexity, moving parts, and human labor that go into creating an innovative industrial AI, it is a good idea to outsource your data annotation project. That way, you know that it is all in compliance with ethical standards and regulations.
You can also ensure that all the fine details and useful information aren't lost in the noise. That way, your machine learning algorithms can make the most of the most amounts of data.
No matter the final AI product, that is critical for accuracy and successful results. Supervised learning can help increase trust in any AI project. Deep learning can help machines accumulate the equivalent of years of expert human experience.
Behind that, AI and ML are a vast amount of detailed data and metadata. That all still takes human intelligence and labor working with the best tools available. That is part of the value that data annotation services provide.
Comments ()