Data Annotator Careers: Opportunities and Growth Prospects
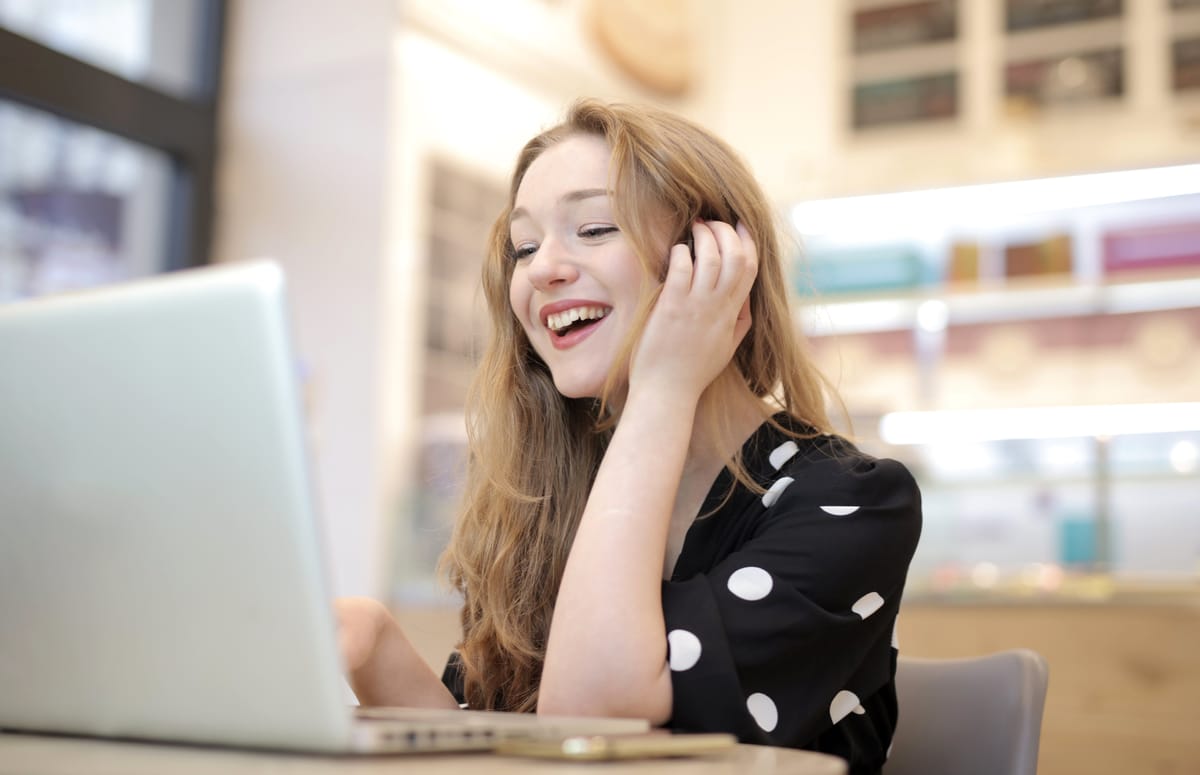
The field of data annotation has witnessed rapid growth and offers promising career prospects for individuals interested in AI and machine learning. Data annotation specialists play a vital role in the development of AI models by labeling data to train them to recognize specific patterns and information.
Data annotation has applications across various industries, including chatbot companies, finance, medicine, and government programs. With the increasing adoption of language models, training techniques, and AI tools, the demand for data annotators has soared. The market for AI and ML data labeling is projected to reach USD 3.5 billion by 2024, opening up numerous job opportunities in this field.
To succeed as a data annotator, individuals need to possess skills in analytical mathematics, ML libraries, programming languages like Python and Java, image analysis algorithms, and tools like OpenCV and Keras. Freshers can expect competitive packages ranging from INR 1.1 lakhs to INR 3 lakhs per annum.
Companies offering data labeling services, such as Acclivis Technologies, Keymakr, Zuru.ai, Cogito Tech, IMerit, and Wisepl, are actively hiring data annotators.
Key Takeaways:
- Data annotation is a critical step in supervised learning, teaching AI and ML models to recognize specific data types.
- The demand for data annotators has surged with the rise in language models, training techniques, and AI tools.
- Data annotation offers diverse career opportunities across sectors like chatbot companies, finance, medicine, and government programs.
- Skills in analytical mathematics, ML libraries, programming languages, and image analysis algorithms are essential for data annotator roles.
- Freshers can expect attractive packages ranging from INR 1.1 lakhs to INR 3 lakhs per annum.
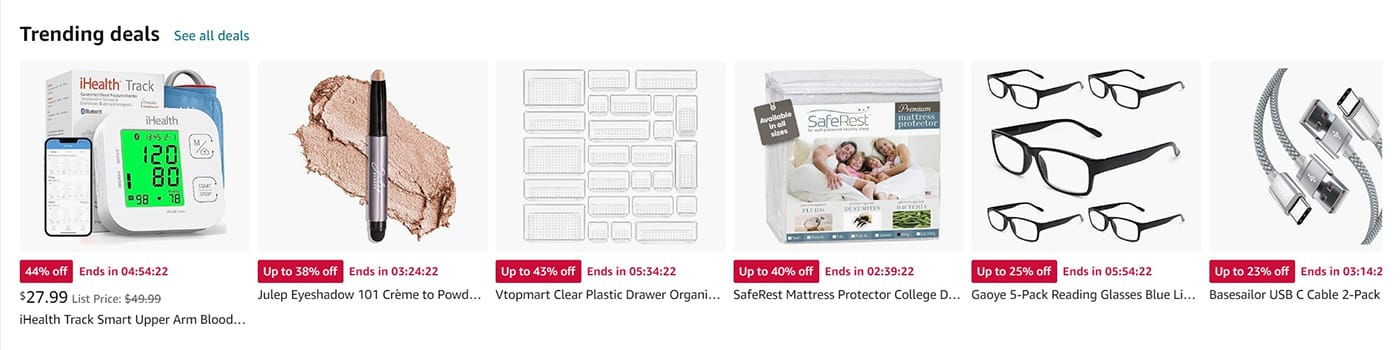
Types of Data Labelling
Data can be labelled in various ways to optimize AI/ML models. Different types of data require specific annotation techniques to train AI systems effectively. The three main types of data labelling are visual data annotation, audio data annotation, and text data annotation. Each type requires distinct skills and tools to ensure accurate and precise annotations.
1. Visual Data Annotation
Visual data annotation involves labelling images using techniques such as bounding boxes, polygons, or semantic segmentation. It is commonly used in computer vision applications, object detection, and image recognition.
Skills and tools required for visual data annotation:
- Mathematical analysis
- Machine learning libraries
- Programming languages such as Python and Java
- Image analysis algorithms
- Tools like OpenCV and Keras
2. Audio Data Annotation
Audio data annotation is used in various applications such as natural language processing, speech recognition, transcription, and virtual assistants. It involves annotating audio files to enable machine learning models to understand and interpret spoken language.
Skills and tools required for audio data annotation:
- Spectrogram analysis
- Machine learning libraries
- Programming languages
- Auditory database management
- Tools like Audacity and Adobe Audition
3. Text Data Annotation
Text data annotation is crucial for training AI systems to interpret written language and perform tasks such as sentiment analysis, named entity recognition, and text categorization. It involves labelling text documents with relevant annotations to create labeled datasets for machine learning models.
Skills and tools required for text data annotation:
- Computational linguistics
- Machine learning knowledge
- Database management
- Programming languages like Python and Java
- Tools like GATE and NLTK
Each type of data annotation requires specific skills, expertise, and tools to ensure accurate and meaningful annotations. Data annotators play a crucial role in improving AI/ML models and enabling them to perform tasks with precision.
Career Prospects in Data Annotation
The data analytics industry is currently experiencing a significant boom in India, creating a high demand for skilled professionals in various roles such as data engineers, data analysts, data labellers, and data scientists. Among these roles, data annotation specialists play a crucial role in training AI and ML models by providing accurate and comprehensive data labels.
Data annotators require a diverse skill set to excel in their role. Proficiency in machine learning is essential, as they need to understand and apply various ML algorithms to ensure precise data annotation. Additionally, knowledge of tools specific to the type of annotation, such as image analysis algorithms and programming languages like Python and Java, is crucial for efficient and accurate labeling.
Attention to detail is a key attribute for data annotators, as they are responsible for meticulously labeling data and ensuring its quality. The ability to focus on complex tasks for extended periods of time is also vital in handling different aspects of the machine training process.
Job prospects for data annotation specialists are promising, with a wide range of companies offering attractive packages based on skills and experience. Industry leaders like Siemens, Apple, and Google frequently hire data annotators to support their AI initiatives and enhance their machine learning models.
Implementing secure and cost-effective data labeling methods is essential for the smooth operation of AI models. Data annotation specialists play a vital role in shaping the future of AI and enabling advancements in various fields, such as healthcare, finance, and autonomous vehicles.
To showcase the demand for data annotator roles in the data analytics industry, the table below highlights the annual average salaries for data annotation professionals:
| Role | Average Salary |
| -------------------- | --------------- |
| Data Annotator | $70,000 - $90,000 |
| Senior Data Annotator| $90,000 - $120,000 |
The data above represents the average salaries in the United States, which can vary based on factors such as experience, location, and industry.
In conclusion, the career prospects for data annotation specialists are promising, with increasing demand in the data analytics industry. Professionals with the necessary skills and expertise have the opportunity to contribute to groundbreaking projects and work with renowned companies in the field of AI and machine learning. As the demand for AI technology continues to grow, data annotation specialists will play a crucial role in driving innovation and shaping the future of AI.
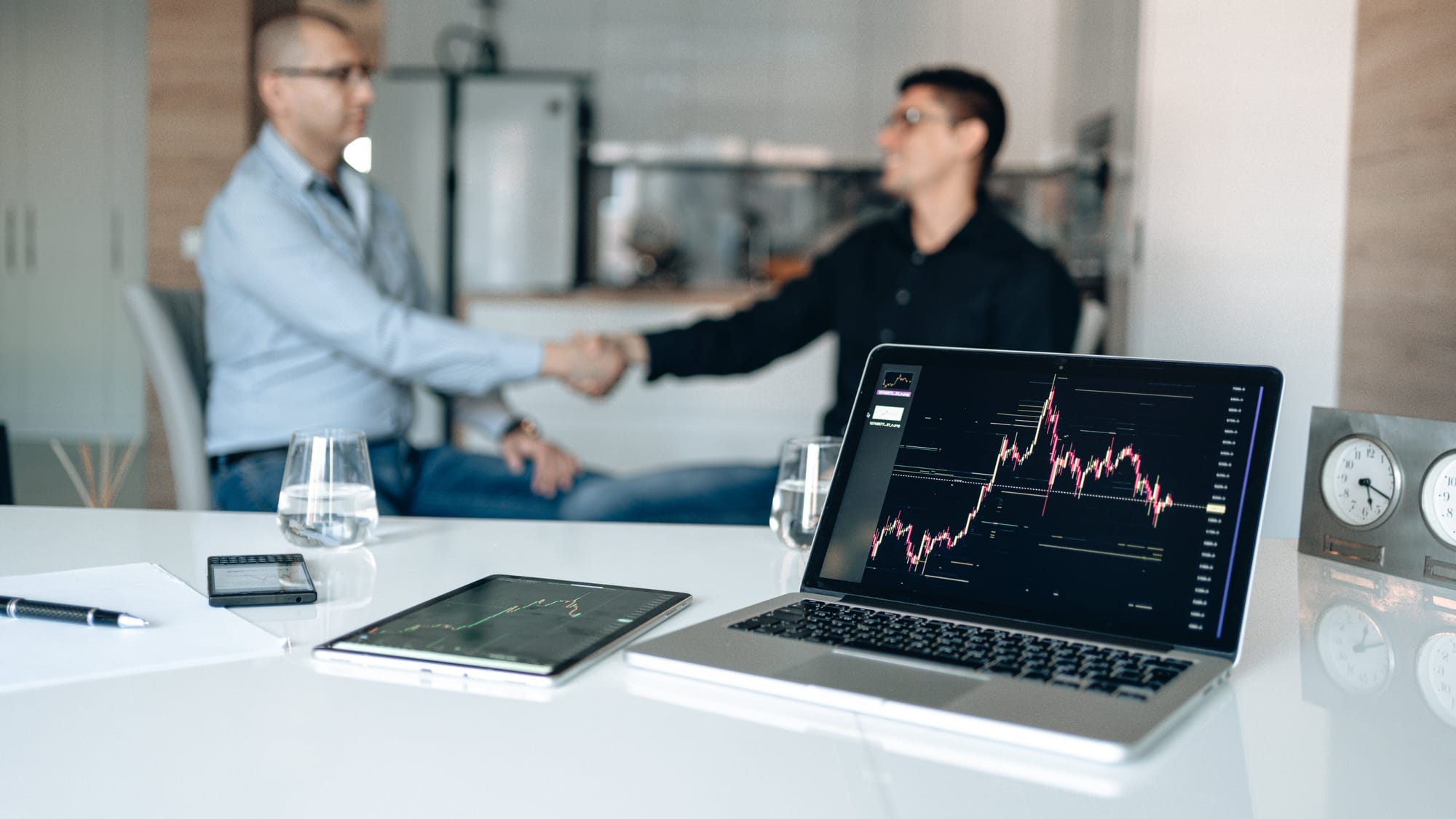
The Growth of Data Annotation Market
The data annotation market is witnessing significant growth and is projected to reach USD 8.22 billion by 2028, with a compound annual growth rate (CAGR) of 26.6% through 2030. This market expansion can be attributed to several factors driving the demand for annotated data.
Complex datasets for high-level machine learning (ML): As ML algorithms advance, there is a need for more intricate and diverse datasets to enhance the capabilities of AI models. Annotated data plays a crucial role in training these advanced models, enabling them to understand complex patterns and make accurate predictions.
Real-time data annotation requirements: With the rapid pace of data generation, real-time annotation has become essential. Industries such as healthcare, finance, and autonomous vehicles rely on up-to-date annotations to optimize their systems' performance and ensure accurate decision-making.
Trend towards automation in the labeling workflow: Automation is revolutionizing the data annotation process, making it more efficient and scalable. By leveraging automated annotation tools and algorithms, companies can accelerate the labeling process, reducing costs and increasing productivity.
Visual data annotation is a critical aspect of AI development, particularly in computer vision applications like object detection, facial recognition, and autonomous driving. Accurate annotation of visual data enables AI models to comprehend images and videos, leading to improved performance and reliability.
Generative AI's Impact on Data Annotation
As the data annotation market evolves, the emergence of generative AI technologies is expected to bring significant changes. Generative AI can automate tasks like image segmentation and the generation of synthetic data based on textual input, streamlining the annotation process and boosting efficiency.
Generative AI will revolutionize the data labeling market by automating dataset labeling and serving as a supplementary tool for human annotators. While algorithms for automatic data labeling are being developed, human supervision remains crucial for ensuring accuracy.
The adoption of generative AI will contribute to the increased demand for labeled data, as AI models become more sophisticated and require larger and higher-quality datasets.
Factors | Description |
---|---|
Complex datasets for high-level ML | Enables AI models to understand intricate patterns and make accurate predictions |
Real-time data annotation requirements | Essential for industries where up-to-date annotations are crucial for reliable decision-making |
Trend towards automation in the labeling workflow | Increases efficiency, scalability, and productivity of the data annotation process |
Visual data annotation | Crucial for computer vision applications, enhancing AI models' ability to comprehend images and videos |
Generative AI's Impact on Data Labeling
Generative AI, with its ability to create content in various formats, has revolutionized the field of data labeling, bringing automation to the forefront of data annotation processes. This groundbreaking technology streamlines the annotation workflow by automating tasks such as image segmentation and image generation from text. By leveraging generative AI, data annotation becomes more efficient and accurate, significantly reducing the time and effort required for manual labeling.
"Generative AI has brought remarkable advancements to the field of data labeling. Its automation capabilities have transformed the way we annotate data, allowing for faster and more precise results."
While algorithms for automatic data labeling are currently under development, human supervision remains crucial for maintaining accuracy and resolving complex labeling scenarios. Generative AI serves as a supplementary tool in the data labeling process, augmenting human efforts and enhancing the quality of annotations.
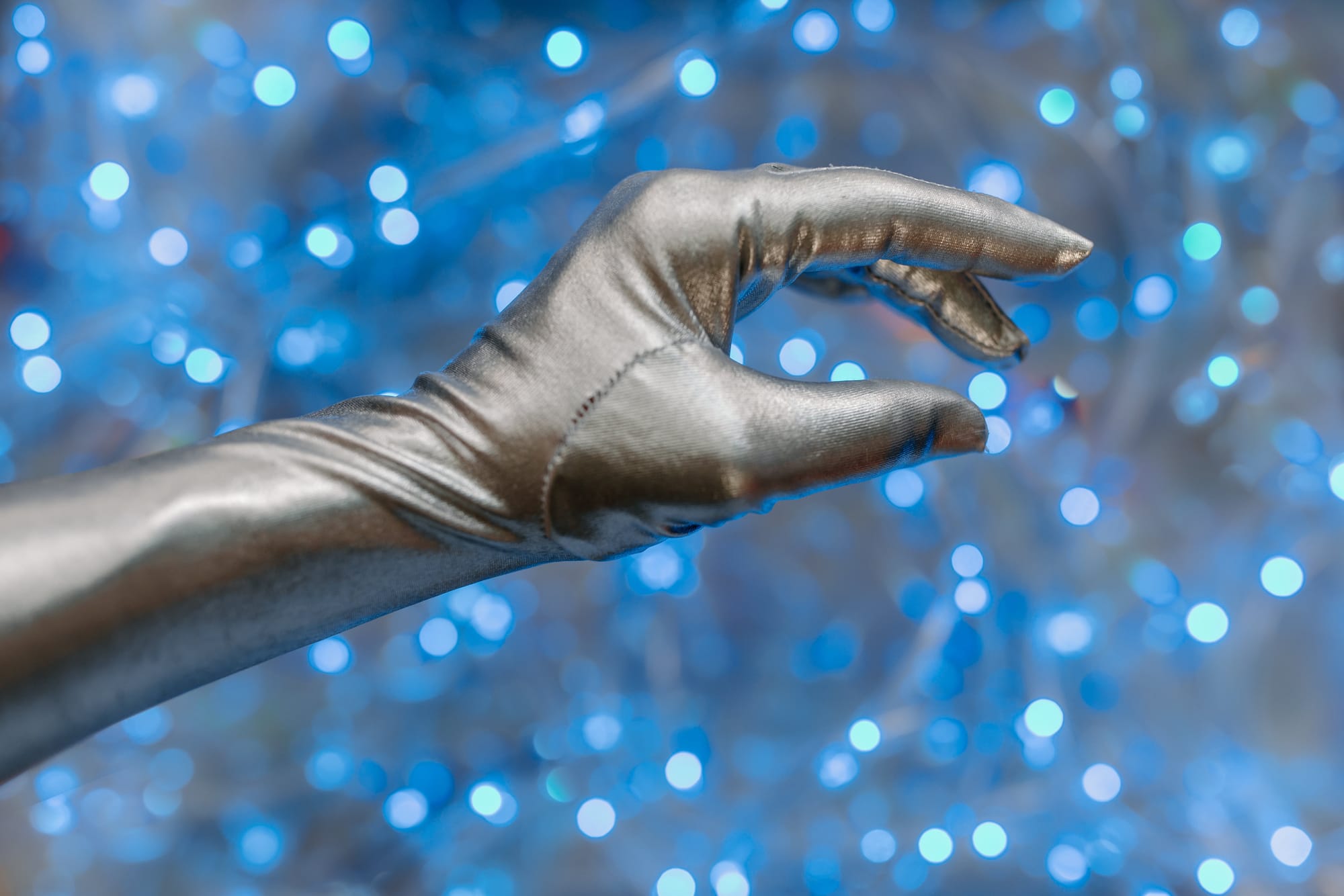
Automated Dataset Labeling
Generative AI enables automated dataset labeling, reducing the dependency on manual labeling tasks. With its ability to generate data labels based on predefined rules and patterns, generative AI facilitates the creation of labeled datasets at scale. This automation not only accelerates the data annotation process but also addresses the increasing demand for labeled data in the AI industry.
By automating certain aspects of the labeling workflow, generative AI empowers data annotators to focus on more complex and nuanced labeling tasks, ensuring that the annotated data is of high quality and aligns with specific AI objectives.
Enhancing High-Quality Annotation
The integration of generative AI in data labeling processes contributes to the production of high-quality annotations. With its advanced capabilities, generative AI enhances the accuracy and consistency of annotations, minimizing human errors and biases. This technology also improves the precision of image segmentation and enables the generation of diverse and realistic images from text, enhancing the overall quality of labeled datasets.
The implementation of generative AI in data labeling not only boosts efficiency but also drives innovation in AI and machine learning. It paves the way for advancements in semi-supervised learning and active learning, enabling models to learn from a large volume of unlabeled data with minimal human intervention.
Generative AI's impact on data labeling is a significant milestone in the field of AI and machine learning. It revolutionizes the way data is annotated, combining automation with human expertise to produce high-quality labeled datasets. As the demand for labeled data continues to grow, the integration of generative AI in data annotation processes will play a pivotal role in meeting the evolving needs of the AI industry.
Top Trends in Data Annotation Market
As the data annotation market evolves, several key trends are shaping its landscape. These trends include the boom in unstructured data, the rise of large language models, the importance of visual data annotation, the impact of generative AI, the role of automation in the labeling workflow, and the more stringent data requirements for AI. Let's explore each trend in detail:
1. Boom in Unstructured Data
The proliferation of unstructured data, such as text, images, audio, and videos, presents both opportunities and challenges in the data annotation market. Companies are focusing on handling unstructured data to enhance the intelligence of AI systems and unlock valuable insights. Proper annotation and labeling of unstructured data facilitate accurate analysis and interpretation by AI models.
2. Rise of Large Language Models
Large language models, such as GPT-3 and BERT, have gained significant prominence in natural language processing tasks. These models require high-quality annotations and labeled data to understand and generate human-like language. Accurate annotation plays a crucial role in training and fine-tuning these models, ensuring their optimal performance in various applications like language translation, sentiment analysis, and chatbots.
3. Importance of Visual Data Annotation
Visual data annotation plays a vital role in tasks like image recognition, object detection, and autonomous driving. It involves the precise labeling of images, utilizing techniques like bounding boxes, polygons, or semantic segmentation. Accurate annotation of visual data enables AI models to accurately identify and understand objects, shapes, and patterns, leading to improved performance in computer vision applications.
4. Impact of Generative AI
Generative AI, which can create content in various formats, has revolutionized the data annotation process. It can automate tasks like image segmentation and even generate new images based on textual inputs. While generative AI offers exciting possibilities for automating the annotation workflow, human supervision is still essential to ensure accuracy and quality. With the integration of generative AI, the data labeling process becomes more efficient and scalable, addressing the increasing demand for labeled data.
5. Role of Automation in Labeling Workflow
Automation is reshaping the labeling workflow by streamlining time-consuming and repetitive tasks. Automated tools and algorithms enable faster and more efficient annotation, reducing human effort and speeding up the overall process. By automating certain aspects of the labeling workflow, data annotators can focus on more complex and nuanced tasks, ensuring higher accuracy and productivity.
6. Stricter Data Requirements for AI Systems
With the advancement of AI systems, the data requirements for training and fine-tuning models have become more stringent. High-quality annotations are imperative to ensure the reliability and robustness of AI systems. Data must be labeled accurately and consistently, adhering to predefined standards and guidelines, to achieve optimal performance and minimize potential bias or errors.
These trends are reshaping the data annotation market, driving innovation and advancements in AI technology. Keeping pace with these trends is crucial for both data annotators and organizations to leverage the full potential of AI and meet the evolving demands of the industry.
Comparison of Trends in Data Annotation Market
Trend | Description |
---|---|
Boom in Unstructured Data | The exponential growth of unstructured data, requiring effective annotation methods. |
Rise of Large Language Models | The increasing prominence of large language models and their need for high-quality annotations. |
Importance of Visual Data Annotation | The critical role of accurate visual data annotation in computer vision tasks. |
Impact of Generative AI | The revolutionary impact of generative AI in automating annotation tasks and improving efficiency. |
Role of Automation in Labeling Workflow | The integration of automated tools and algorithms to streamline the annotation process. |
Stricter Data Requirements for AI Systems | The need for higher quality and standardized annotations to enhance AI system performance. |
Major Technological Trends in the Next Decade
In the next decade, the data annotation industry will be shaped by several major technological trends. These trends will have a significant impact on the way data is labeled and annotated, leading to advancements in AI and machine learning. Some of the key technological trends to watch out for include:
The Massive Generation of Data
With the advent of new technologies and the increasing adoption of digital platforms, the generation of data is reaching unprecedented levels. The rapid growth of data from various sources such as social media, IoT devices, sensors, and machine-generated content will provide abundant opportunities for data annotation. This trend will require scalable and efficient annotation techniques to handle the massive influx of data.
Rising Popularity of Facial Recognition Technology
Facial recognition technology is gaining traction across various industries, including law enforcement, retail, and banking. This technology relies heavily on accurate and reliable data annotation to train AI models to recognize and identify faces. As facial recognition becomes more ubiquitous, the demand for high-quality annotated facial data will continue to grow.
Increasing Demand for Autonomous Driving Solutions
The automotive industry is experiencing a significant shift towards autonomous driving solutions. Data annotation plays a crucial role in training AI models for autonomous vehicles, enabling them to recognize objects, pedestrians, and road signs. As the demand for autonomous driving solutions continues to rise, data annotation will play a pivotal role in ensuring the safety and reliability of these systems.
Evolving AI and ML Trends
The field of AI and machine learning is constantly evolving, with new algorithms, techniques, and models being developed. As AI and ML technologies become more sophisticated, the need for accurate and diverse annotated data will become paramount. This trend will drive the development of advanced data annotation methodologies and tools to meet the evolving requirements of AI and ML applications.
The data annotation industry will continue to witness significant growth and innovation driven by these technological trends. To keep up with the evolving landscape, specialized annotation services tailored to specific domains will emerge, providing industry-specific expertise and ensuring the production of high-quality annotations.
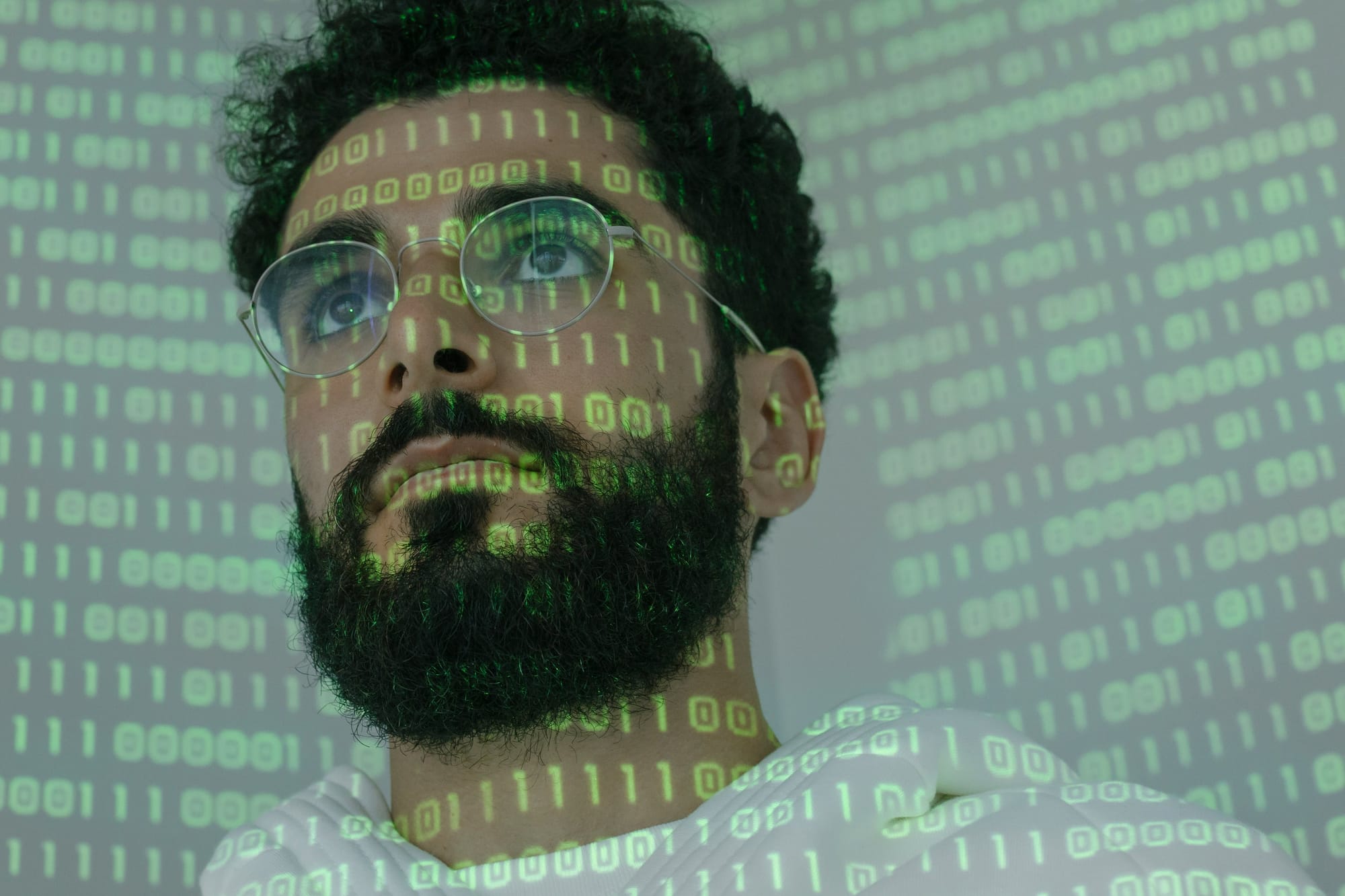
Remote Data Annotator Jobs: Opportunities and Requirements
Remote data annotator jobs provide individuals with the flexibility to work from the comfort of their homes or any location of their choice. These positions present an excellent opportunity to gain experience in the exciting fields of AI and machine learning. Additionally, remote work allows individuals to enjoy the benefits of a flexible schedule, as part-time positions are often available, allowing them to work at their desired hours.
Top companies, such as Expertia AI, Cognitive Collective, and Motive, are actively seeking remote data annotators to support their projects and initiatives. These companies offer attractive compensation packages and the chance to work on cutting-edge projects at the forefront of AI and machine learning advancements.
Requirements
To excel in remote data annotator roles, companies typically seek candidates who possess the following:
- Relevant Background: A bachelor's degree in a field related to data annotation, AI, or machine learning is often preferred. Fields such as computer science, data science, or mathematics provide a solid foundation for understanding the intricacies of the job.
- Attention to Detail: As a data annotator, meticulous attention to detail is essential to accurately label and annotate data. This ensures the quality and integrity of the annotated datasets used to train machine learning models.
- Strong Communication Skills: Effective communication is crucial in remote settings. Clear and concise communication helps facilitate collaboration with team members and project stakeholders, leading to successful data annotation projects.
- Technical Aptitude: Familiarity with programming languages and libraries commonly used in data annotation, such as Python and TensorFlow, is advantageous. Knowledge of machine learning concepts and algorithms is also valuable in understanding the context and requirements of the annotation task.
By meeting the requirements and showcasing their abilities, individuals can position themselves for success in remote data annotator roles and pave the way for exciting opportunities in the AI and machine learning industry.
Importance of Remote Data Annotation
Remote data annotation plays a crucial role in training AI models and is essential for the success of various industries. It enables AI systems to improve their performance by accurately understanding and interpreting complex information. The ability to annotate data remotely offers tremendous flexibility, allowing individuals to work from the comfort of their homes and maintain a healthy work-life balance. This section highlights the benefits of remote data annotator jobs and sheds light on the valuable opportunities they provide.
Benefits of Remote Data Annotator Jobs
Remote data annotator jobs offer numerous advantages to individuals seeking to gain experience in AI and machine learning. Here are some key benefits:
- Flexibility: Remote data annotators enjoy the freedom to work from any location, eliminating the need for commuting and providing the flexibility to choose their own working hours. This level of autonomy allows individuals to tailor their work schedules to fit their lifestyle and personal commitments.
- Healthy Work-Life Balance: With remote data annotation, individuals have the opportunity to strike a balance between their professional and personal lives. By working from home, they can save time on commuting, spend more time with loved ones, and pursue personal interests outside of work.
- Career Growth: Remote data annotation jobs offer a pathway for individuals to grow their careers in the exciting fields of AI and machine learning. By gaining hands-on experience in data annotation, individuals can develop valuable skills and expand their knowledge in this rapidly evolving industry.
- Competitive Compensation: Remote data annotator roles often come with competitive compensation packages. Companies recognize the importance of skilled annotators and are willing to offer attractive remuneration to attract top talent. As the demand for remote data annotators continues to grow, there is potential for earning higher salaries and additional benefits.
Remote data annotation provides a unique opportunity for individuals to contribute to cutting-edge AI projects while enjoying the benefits of remote work. The combination of flexibility, work-life balance, career growth, and competitive compensation makes remote data annotator jobs highly sought after in the AI industry.
Benefits of Remote Data Annotator Jobs |
---|
Flexibility |
Healthy Work-Life Balance |
Career Growth |
Competitive Compensation |
Conclusion
Remote data annotator jobs offer exciting opportunities for individuals passionate about the field of AI and machine learning. As the demand for data annotation specialists continues to grow, so does the potential for career growth in this industry. Remote data annotators play a crucial role in the development of AI models, contributing to groundbreaking projects and driving innovation.
One of the key benefits of remote data annotator jobs is the flexibility they offer. With the ability to work from home or any location, individuals can enjoy a better work-life balance while still being part of an industry that is at the forefront of technological advancements.
Moreover, remote data annotator jobs provide competitive compensation packages, reflecting the high value placed on these professionals within the industry. As AI technology continues to advance, the demand for remote data annotation will only continue to increase, making it an attractive career path for those seeking rewarding and fulfilling work opportunities. With the potential for career growth and the opportunity to contribute to cutting-edge developments, remote data annotator jobs are an excellent choice for individuals looking to make their mark in the field of data annotation.
FAQ
What is data annotation?
Data annotation is the process of labelling data to teach AI and ML models to recognize specific data types. It is a critical step in supervised learning.
What are the different types of data labelling?
There are three main types of data labelling: visual data annotation, audio data annotation, and text data annotation.
What skills are required for a data annotator role?
Data annotators require skills in analytical mathematics, ML libraries, programming languages like Python and Java, image analysis algorithms, and specific tools depending on the type of annotation.
What are the job prospects in data annotation?
The demand for data annotation specialists is increasing, and career opportunities can be found in various sectors such as chatbot companies, finance, medicine, and government programs.
What is the growth potential of the data annotation market?
The data annotation market is projected to reach USD 8.22 billion by 2028, with a CAGR of 26.6% through 2030.
What is the impact of generative AI on data labeling?
Generative AI can automate tasks like image segmentation and streamline the annotation process. It will increase the demand for labeled data and contribute to high-quality annotation.
What are the top trends in the data annotation market?
The top trends include the boom in unstructured data, the rise of large language models, the importance of visual data annotation, the impact of generative AI, automation in the labeling workflow, and more stringent data requirements for AI systems.
What are the major technological trends in the data annotation industry?
Major technological trends include the massive generation of data, the popularity of facial recognition technology, the demand for autonomous driving solutions, and the evolving AI and ML trends.
What are the requirements for remote data annotator jobs?
Requirements typically include a bachelor's degree in a relevant field and attention to detail. Remote data annotator jobs offer flexibility and the opportunity to gain experience in AI and machine learning.
Why is remote data annotation important?
Remote data annotation is crucial for training AI models and plays a vital role in various industries. It offers flexibility, the ability to work from home, and opportunities for career growth.
Comments ()