Enhance Automation with Robotics Image Annotation for AI Systems
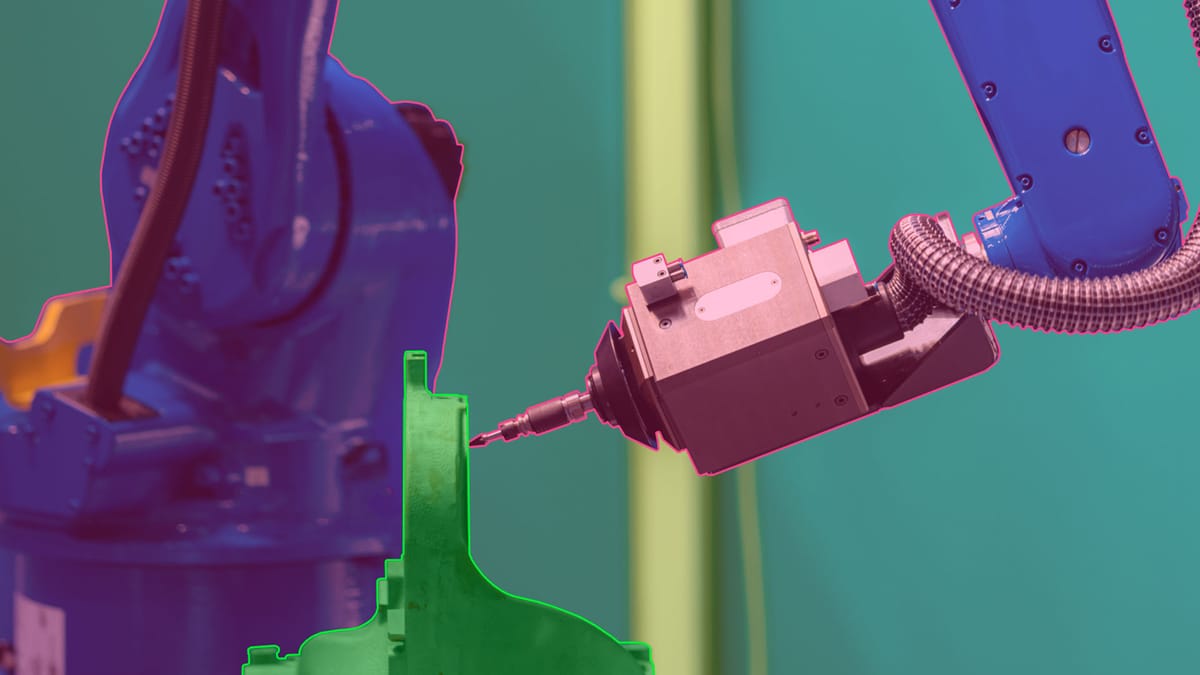
Merging robotics image annotation with AI systems is at the forefront of innovation. This move significantly boosts automation in numerous sectors. By giving machines the power to understand and interact with their surroundings, we see a leap in AI's precision and speed. This integration is set to revolutionize how industries function, opening doors to more sophisticated uses of robotics in daily life.
Key Takeaways
- Annotated images and videos are vital for boosting automation in fields like manufacturing and home appliances.
- Annotation companies provide a range of annotation methods for robotics AI, including Bounding Box and Semantic Segmentation.
- High-quality data labeling boosts manufacturing efficiency and safety in both production lines and homes.
- Proper labeling enables robots to spot defects and grasp human behavior more effectively.
- Robotics AI gains from image and video annotation for tasks like object detection, environmental sensing, and quality control.
- Accurate video annotation ensures enhanced factory safety and more efficient processes.
Introduction to Robotics Image Annotation
Robotics image annotation is a key technique in AI, where visual data is labeled for machine learning models. This step is vital for AI to understand its environment like humans do. It's the foundation of computer vision tasks. By identifying and marking digital image elements, it provides essential training data for AI models.
What is Robotics Image Annotation?
Robotics image annotation labels images for AI to recognize and interpret elements. It's essential for applications like autonomous driving, where models need to spot pedestrians, traffic lights, and other vehicles. Techniques include bounding boxes, polygons, polylines, and key-points, each suited for different tasks.
High-quality data annotation is critical for training effective machine learning models. It's vital in fields like security, agriculture, medical imaging, and wildfire detection. Using diverse annotations and trained annotators on a robust platform is key to project success.
Importance in AI Development
The role of robotics image annotation in AI development is immense. The quality and diversity of annotated images greatly affect machine learning algorithms' performance. Accurate annotations enable models to tackle complex tasks, from object detection to real-time decision-making. For instance, auto annotation tools, using pre-trained algorithms, can handle complex tasks with high precision.
As AI advances, the need for thorough data annotation will increase. Effective AI development relies on access to precisely labeled data, as highlighted by experts in the field. The combination of skilled annotators and advanced tools is essential for meeting these evolving demands. This ensures AI systems remain accurate, efficient, and reliable across various applications.
Key Technologies in Robotics Image Annotation
Robust robotics image annotation technologies are essential for AI systems to tackle complex tasks. The integration of machine learning algorithms, computer vision techniques, and process automation tools boosts AI accuracy in various fields.
Machine Learning Algorithms
Machine learning algorithms are key to improving robotics image annotation. They process large datasets to enhance predictive accuracy. Through analyzing annotated images, these algorithms learn and improve continuously. This ongoing process is vital for tasks like object detection, recognition, and localization.
Computer Vision Techniques
Computer vision techniques allow machines to interpret visual data like humans do. These techniques are essential for automating tasks, quality control, and gesture recognition. By using computer vision, precision in handling components improves, leading to better product quality and safety.
Process Automation Tools
Process automation tools make the annotation workflow more efficient and less manual. These tools automate visual inspection and real-time monitoring, cutting down labor costs and manual effort. The integration of these tools ensures high-quality and accurate annotations, critical for complex AI tasks.
Feature | Benefits |
---|---|
Identification and Localization | Crucial for tasks like picking, sorting, and assembly. |
Automated Visual Inspection | Detects defects in products for high-quality output. |
Failure Prediction | Predicts equipment failures for timely maintenance. |
Safety Improvements | Reduces accidents by enabling robots to respond to human movements. |
Route Optimization | Improves productivity by optimizing routes for autonomous robots. |
Benefits of Robotics Image Annotation for AI
Robotics image annotation is key to advancing AI systems. It boosts accuracy, efficiency, and cuts costs. Through precise annotation, AI models learn to process images better. This leads to higher operational efficiency in automation.
Improved Accuracy and Efficiency
Robotics image annotation significantly enhances AI accuracy. Training AI on detailed images improves its ability to recognize complex patterns. This boosts efficiency in automation, making AI systems more effective. For example, the autonomous vehicle industry benefits greatly, meeting the demand for self-driving cars.
The healthcare sector also relies on this technology for tasks like interpreting medical images. This aids in patient diagnosis and treatment.
Reduced Human Error
Another major advantage is the reduction of human error. Automated tasks lead to fewer mistakes. Robotics image annotation enables AI to handle these tasks with minimal human input. This is critical in fields like medical diagnostics and industrial automation, where precision is essential.
The transportation sector uses bounding boxes to monitor traffic and optimize signal timings. This reduces errors in manual monitoring.
Cost-Effectiveness
Robotics image annotation also offers cost-effective AI solutions. It automates repetitive tasks, freeing up resources for more critical areas. Retail industries benefit from improved inventory management and customer experience.
Automated inventory tracking ensures accurate stock levels and timely replenishment. Services like Amazon Mechanical Turk provide a cost-effective way to outsource image labeling tasks globally. This reduces operational costs, allowing businesses to focus on innovation and growth.
Here is an illustrative table showing various applications and the corresponding industries benefiting from robotics image annotation:
Industry | Application |
---|---|
Autonomous Vehicles | Training algorithms for self-driving cars with bounding boxes |
Healthcare | Medical imaging annotation for X-rays and MRIs |
Retail | Automated inventory management and personalized marketing |
Transportation | Monitoring traffic flow and optimizing signal timing with bounding boxes |
Agriculture | Analyzing crop types and health using annotated satellite or drone images |
Applications of Robotics Image Annotation
Robotics image annotation is vital across various fields. It drives AI advancements, transforming our daily lives and industrial operations.
Autonomous Vehicles
In the field of autonomous vehicles, image annotation is key. It creates the large datasets needed to train AI driving software. By labeling objects in images accurately, these systems can:
- detect obstacles and navigate
- steer and detect lanes
- monitor in-cabin behavior and ensure safety
- track movement and use LiDAR sensing
With annotated data, autonomous vehicles can make safe and efficient decisions. They can identify vehicles, pedestrians, traffic signs, and lanes.
Healthcare Robotics
In healthcare robotics, annotated images are essential for medical diagnostics and precise surgeries. They are used for:
- assisting in precise surgeries
- analyzing medical images like MRIs and CT scans
- improving patient outcomes through better interventions
Image annotation provides a rich source of training data. It helps healthcare robotics evolve, leading to more efficient healthcare solutions.
Industrial Automation
Image annotation boosts smart AI applications in industrial automation. It simplifies complex manufacturing processes:
- ensures quality control by detecting defects
- optimizes production lines and boosts efficiency
- guides robotic arms in precise assembly
- facilitates automated warehouse operations and inventory management
Robotics image annotation significantly impacts industrial automation. It leads to increased productivity and lower error rates.
By providing precise, annotated data, robotics image annotation is a critical tool across sectors. It drives efficiency and innovation in areas like autonomous vehicles, healthcare robotics, and industrial automation.
Challenges in Implementing Robotics Image Annotation
Exploring the complexities of AI management reveals unique hurdles in robotics image annotation. These obstacles are critical to achieving reliable AI performance and scalable systems.
Data Quality Issues
Data quality is essential in robotics image annotation. Low-quality data can skew AI training, resulting in faulty models and unreliable AI. Manual annotation introduces biases and errors, while automated tools face label noise and quality issues. Ensuring high data quality demands a strong framework for audits and feedback from annotators, continually improving annotation methods.
Scalability Concerns
Scalability is vital for AI system deployment. As data volumes increase, systems must process larger datasets efficiently. High-speed annotation is necessary but must not sacrifice accuracy, as errors can spread quickly. Time and financial constraints exacerbate these issues, highlighting the need for strategic prioritization and possibly professional services.
Integration with Existing Systems
Integrating new AI systems with legacy infrastructure poses significant technical challenges. Legacy systems often require complex adaptation to align with advanced AI models. Seamless integration is key for scalable AI systems and reliable performance across diverse applications.
Challenge | Description | Solution |
---|---|---|
Data Quality | Issues with label noise and accuracy variation in annotations. | Implement HITL approach and continuous quality checks. |
Scalability | Handling larger datasets while maintaining accuracy. | Utilize high-speed annotation tools without compromising quality. |
Integration | Adapting new AI systems to coexist with existing infrastructure. | Develop bespoke integration strategies for seamless operation. |
The Role of Data Labeling in AI Systems
In AI systems, the role of data labeling is critical. Accurate labels are essential for precise AI training, ensuring models function as expected. Effective labeling involves adding tags to data like images, text, and audio. This enables algorithms to identify patterns and make accurate predictions.
Importance of Accurate Labels
Accurate labeling is key for training dependable AI models. In supervised learning, the quality of labeled data greatly affects model performance and learning speed. High-quality data is critical for systems needing machine-driven decisions to ensure accurate outcomes. For example, chatbots and speech recognition systems rely heavily on precise annotations.
The data annotation process includes several steps, from data collection to exporting annotated data. Proper guidelines and quality checks ensure the accuracy and consistency of the data. Choosing the right annotation tools or vendors is vital for successful labeling initiatives.
Manual vs. Automated Labeling
Manual labeling offers high accuracy but is impractical for large datasets due to time constraints. Automated annotation technologies are essential, speeding up the labeling process without sacrificing accuracy. Human-in-the-loop labeling combines automated labeling with human oversight, improving both speed and accuracy.
Automated annotation techniques efficiently process large datasets, vital for training advanced AI models. High-quality annotated data supports applications like facial recognition, computer vision, and robotic vision. It also aids in audio and video annotation, enabling algorithms to understand movement patterns.
Advancements in AI and ML
Deep learning technologies, like CNNs and GANs, have transformed image annotation. They've greatly improved accuracy and quality. Transfer learning allows for using pre-trained models for new tasks, saving time and data. These advancements are key in areas where precise annotations are essential, such as autonomous driving and healthcare.
Real-time Annotation Techniques
Real-time annotation is gaining importance, mainly for applications like autonomous driving and surveillance. These methods enable quick data processing and decision-making, vital for dynamic environments. Self-learning annotation tools are also emerging, improving accuracy by learning from past mistakes.
Impact of Edge Computing
Edge computing is transforming image annotation processes. It reduces latency by processing data near its source, leading to faster operations. This is highly beneficial for real-time applications, such as IoT, where immediate responses are critical.
The increasing demand for high-quality, scalable annotation services is evident across various sectors. From smart cities to industrial automation, the need is clear. The combination of advanced AI and edge computing will revolutionize AI's interaction with real-world data. This will significantly enhance the use of robotics image annotation in many industries.
Selecting the Right Tools for Robotics Image Annotation
By carefully selecting annotation tools, we can enhance the accuracy and efficiency of machine learning models.
Key Features to Consider
When evaluating key features in annotation tools, several factors stand out:
- Versatility in handling diverse data types
- Precision to ensure accurate annotations
- Scalability for large datasets
- Automation capabilities for faster processing
Effective annotation solutions must incorporate these attributes to streamline workflows and enhance productivity. The market is expected to grow at a Compound Annual Growth Rate (CAGR) of 27% from 2024 to 2032.
Popular Tools Available
The market offers a variety of popular annotation software with robust functionalities. Keylabs and Keymakr are among the widely recognized tools, famous for their extensive solutions. For instance, Roboflow Annotate is employed by over 250,000 engineers and is renowned for its swift labeling workflows. Labelbox was named in Forbes' 2022 Top AI 50 and has secured multiple high-profile contracts.
AI training tools must cater to different industries' unique requirements. For example, the automotive sector relies heavily on annotated datasets for autonomous driving systems. Selecting annotation tools requires an understanding of specific industry needs and regulatory frameworks.
Below is a comparison table of some popular annotation tools:
Company Name | Key Features | Notable Use Cases |
---|---|---|
Keylabs | Versatility, Precision, Scalability, Automation | Autonomous Vehicles, Healthcare |
Keymakr | Comprehensive Solutions, Teams & Management | Retail, Robotics |
Roboflow Annotate | Swift Labeling Workflows | Engineering, AI Research |
Labelbox | Extensive Toolset, Collaboration | Defense, Innovation Research |
The image annotation tool market's growth is driven by the rapid adoption of AI and machine learning technologies. Enterprises are leveraging automated annotation features and collaborative workflows to boost efficiency and compliance.
The Importance of Continuous Learning in Robotics Image Annotation
Continuous learning in AI is essential for the ongoing success of robotics image annotation. It's vital to regularly update and retrain AI systems. This ensures they stay effective in the face of changing data and real-world scenarios.
Updating Models
Updating AI models is key in robotics image annotation. It involves refining algorithms with the latest data to boost decision-making and operational efficiency. Industries like manufacturing and retail see major benefits, as AI systems can manage inventory and monitor shelves more accurately.
Retraining with New Data
Retraining AI systems with new data is equally critical. It keeps the data relevant, enabling models to handle new scenarios. Adaptive AI technologies are vital in sectors like security and surveillance. Here, accurate traffic monitoring and face identification are essential.
Industry | Application | Impact |
---|---|---|
Manufacturing | Inventory Management | Improves Efficiency and Maintenance |
Retail | Product Catalog Scanning | Automates Shelf Monitoring |
Agriculture | Crop Yield Estimation | Enhances Livestock Management |
Security | Traffic Monitoring | Enhances Security Measures |
The Future of Robotics Image Annotation
As we wrap up our exploration of robotics image annotation, it's evident that this field is vital for AI's future. It enables the creation of training data, essential for developing advanced visual perception models. These models are critical for AI and machine learning (ML) to accurately recognize and predict objects. This is key for progress in sectors like automotive and retail.
By investing in advanced image and data annotation, companies can lead the AI transformation. This will secure a strong position in the future of AI.
FAQ
What is Robotics Image Annotation?
Robotics image annotation involves tagging digital images with labels for AI and machine learning models. It's about identifying and marking elements in images. This process provides essential training data for robotic systems to understand and interact with the visual world.
Why is Robotics Image Annotation important in AI Development?
It's vital for AI development as it boosts the accuracy and efficiency of AI models. With well-annotated images, AI can recognize objects, detect defects, and make decisions in real-time. This enhances automation across various industries.
What are the key technologies involved in Robotics Image Annotation?
Key technologies include advanced machine learning algorithms, computer vision, and automation tools. These technologies improve AI accuracy, streamline the annotation process, and enable real-time analysis and decision-making.
How does Robotics Image Annotation improve AI accuracy and efficiency?
It trains AI models with precise images, leading to more accurate and efficient tasks. This reduces errors and allows AI to handle repetitive tasks with minimal human oversight. It makes operations cost-effective and efficient.
In which industries is Robotics Image Annotation commonly applied?
It's used in autonomous vehicles for obstacle detection and navigation, healthcare robotics for surgeries and diagnostics, and industrial automation for manufacturing and quality control.
What are the main challenges in implementing Robotics Image Annotation?
Challenges include ensuring training data quality, addressing scalability, and integrating AI with existing technologies. Overcoming these is key for reliable AI performance and seamless functionality.
What is the role of data labeling in AI systems?
Data labeling is critical for training reliable AI models. Accurate labels ensure AI systems perform as intended. While manual labeling is precise, automated technologies are essential for large datasets without sacrificing accuracy.
What future trends are expected in Robotics Image Annotation?
Future trends include refined real-time annotation, AI and machine learning advancements, and edge computing integration. These will revolutionize AI interactions with real-world data, improving AI capabilities in dynamic environments.
How do I choose the right tools for Robotics Image Annotation?
Consider versatility, precision, scalability, and automation when choosing tools. Tools like Keylabs and Keymakr offer robust functionalities that streamline the annotation process.
Can you provide examples of successful implementations of Robotics Image Annotation?
Successful implementations include advancements in autonomous driving, improving vehicle safety and reliability. In healthcare, precise annotations have enhanced robotic surgeries, leading to better patient outcomes.
Why is continuous learning important in Robotics Image Annotation?
Continuous learning keeps AI models effective and relevant. Regular updates and retraining with new data allow AI to adapt to evolving conditions. This ensures they handle novel scenarios accurately and efficiently.
Comments ()