Enhance Machine Learning Models with High-Quality Medical Data Annotation
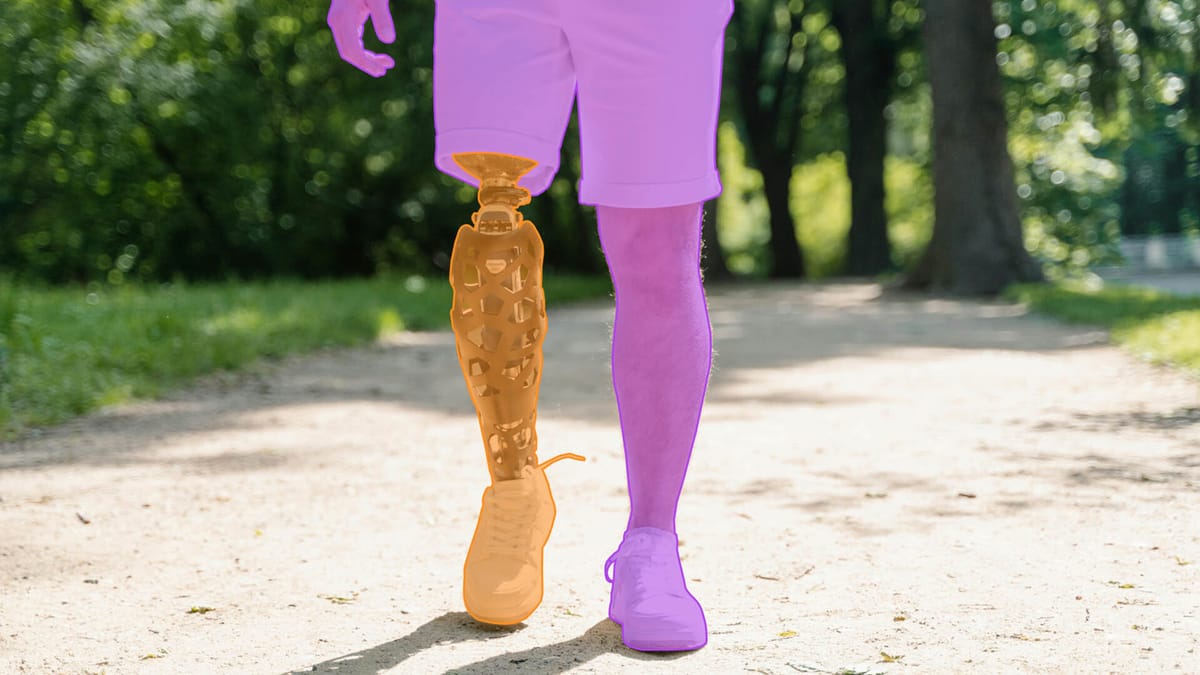
The importance of medical data annotation cannot be overstated. It provides accurately labeled data that boosts model performance in tasks like image recognition, natural language processing (NLP), and video analysis. Poor quality data often leads to underperforming AI/ML models, resulting in inconsistent or subpar results. So, delivering quality training data is key to the success of healthcare AI enhancement.
Key Takeaways
- Medical data annotation is vital for high-performing machine learning models.
- High-quality data sets are essential for AI applications in healthcare.
- Poor quality data can lead to unreliable and inconsistent AI outcomes.
- Accurately labeled data enhances model performance, significantly in diagnostic and patient care applications.
- Investing in quality training data is fundamental to maximizing the benefits of healthcare AI technologies.
Understanding the Importance of Medical Data Annotation
In today's healthcare world, medical data annotation is key to creating strong AI systems. It involves accurately labeling various medical data types, like text, images, and videos. This is to train algorithms for predictive analysis, diagnosis, and making decisions on their own.
What is Medical Data Annotation?
Medical data annotation is the detailed process of labeling medical datasets. This makes the data structured for machine learning models to use. It includes annotating medical images, clinical records, and audio recordings. This ensures algorithms get high-quality, accurately labeled data to learn from and make reliable predictions.
Why Medical Data Annotation Matters
The role of data annotation in healthcare is vital. Accurate medical data annotation is essential for training AI models that improve diagnosis. Properly labeled data helps in accurate diagnosis, personalized treatment plans, and better patient outcomes. It allows medical professionals to extract valuable insights, streamline diagnostic processes, and reduce patient wait times.
Also, combining data annotation with AI technologies is transforming patient care. Predictive analytics help healthcare providers anticipate health risks, tailor treatments, and prevent conditions from worsening. Accurate annotations, whether audio, text, or images, greatly contribute to better patient care, enhanced diagnostic accuracy, and healthcare efficiency in the United States.
Yet, challenges like data privacy, the complexity of medical terms, and tool robustness must be tackled for successful healthcare data labeling. It's critical to follow strict privacy and security measures to protect patient information and meet HIPAA guidelines.
The Role of Medical Data Annotation in Machine Learning
In healthcare, medical data annotation is essential. It trains machine learning models with precise data, boosting their performance. This process improves diagnostic accuracy and ensures AI fairness.
Enhancing Algorithm Accuracy
Enhancing algorithm accuracy is a key benefit of medical data annotation. Companies like AnnotationBox.com are transforming healthcare data management in the USA. Experts label medical images, helping AI spot patterns and anomalies quickly and accurately.
Reducing Bias in Machine Learning Models
Diverse data sets are vital for reducing bias in AI. Annotating a wide range of medical data trains models on representative data, ensuring fairness in AI-driven healthcare. Clinical data annotation is key for equitable treatment and efficient patient data management.
Medical Natural Language Processing (NLP) Annotation interprets clinical documentation, transforming unstructured texts into structured formats. This supports AI systems in making informed decisions, highlighting the role of data annotation in healthcare transformation.
Field | Annotation Type | Impact |
---|---|---|
Radiology | Image Annotation | Early diagnosis, AI-assisted detection |
Clinical Trials | Text Annotation | Improved patient data management |
Surgical Procedures | Video Annotation | Instrument tracking, precision in interventions |
Types of Medical Data Annotations
In the rapidly changing world of medical data annotation, various annotation types are vital for AI's growth in healthcare. Accurate annotations lead to better diagnostics, efficient patient care, and AI's role in complex medical tasks.
Image Annotation in Radiology
Medical image annotation is key in radiology, helping AI systems spot malignant from benign conditions. This is vital for creating precise diagnostic tools that aid both radiologists and patients.
Text Annotation for Clinical Records
Clinical text annotation transforms vast clinical records into usable data. This process allows healthcare providers to monitor diseases, manage patient outcomes, and enhance healthcare delivery.
Video Annotation in Surgical Procedures
Video annotation in healthcare, focusing on surgical procedures, brings about major advancements. Annotated videos train AI to assist or even perform surgeries autonomously. This technology can greatly enhance surgical precision and patient results.
The medical data annotation process involves several types, including polygonal annotation, image segmentation, image classification, and named entity recognition (NER).
The Annotation Process: Step-by-Step
Understanding the annotation process in healthcare is key to improving AI model accuracy and efficiency. It involves several steps, including data collection and preparation, tool selection, and quality control. These steps are critical for achieving high-quality annotations.
Data Collection and Preparation
The first step in the annotation process is data collection and preparation. This involves gathering high-quality data specific to the project goals. This data can include images, videos, audio files, or text. To enhance the dataset, data augmentation techniques are used.
Having a diverse and representative dataset is essential. It helps reduce bias and improves model accuracy. A well-prepared dataset is the foundation for a robust AI model.
Choosing Annotation Tools
Selecting the right annotation tools is critical for precision and efficiency. Consider factors like user interface, annotation capabilities, and collaboration features. The tools should integrate well with AI models and be scalable.
For medical data, tools must support DICOM formats and provide structured export options. This ensures compliance with privacy and data handling standards. The right tools facilitate seamless interaction, making annotation easier.
Here is a detailed comparison of essential features to consider when selecting annotation tools:
Feature | Importance | Examples |
---|---|---|
User Interface | High | Simplicity, Ease of Use |
Annotation Capabilities | Critical | Bounding Box, Polygon |
Collaboration | Moderate | Team Access, Real-Time Updates |
Integration with AI Models | High | API Support, Structured Outputs |
Scalability | High | Cloud Support, Parallel Processing |
Quality Control and Validation
Ensuring data integrity through quality control and validation is vital. This involves regular checks and the use of gold-standard data. Providing detailed guidelines and feedback to annotators is essential for maintaining consistency and accuracy.
Automated tools and manual review help refine annotations. This makes them reliable for training AI models in healthcare. Quality control improves AI model accuracy and ensures compliance with healthcare standards.
Regular review and updates of annotations are necessary. This keeps them relevant and accurate, supporting AI model improvement.
Challenges in Medical Data Annotation
Medical data annotation is key for training precise and unbiased machine learning models in healthcare. Yet, several challenges in data annotation hinder professionals. These hurdles include variability in data quality, the intricacy of medical terminology, and the need to balance speed with precision.
Variability in Data Quality
Ensuring data quality is a major concern in annotating medical data. Low-quality data can severely impact machine learning model performance, resulting in incorrect predictions and diagnoses. It's estimated that poor data quality can cost companies about 15% of their revenue. To address this, regular quality checks and testing are vital.
The Complexity of Medical Terminology
The complexity in medical annotations stems from the specialized and detailed nature of medical terminology. Annotators with extensive knowledge of medical terms and conditions are essential. Tools like Labelbox, which offer customizable quality review workflows, are invaluable in this context.
Balancing Speed and Accuracy
Another significant challenge is balancing the speed of annotation with accuracy. Manual annotation, though precise, is time-consuming and expensive. Automated methods, while scalable and cost-effective, may not be as precise. A hybrid approach, combining automation with manual reviews, can efficiently manage large data volumes while maintaining high-quality outputs.
Annotation Method | Advantages | Challenges |
---|---|---|
Manual Annotation | High Accuracy | Labor-Intensive, Costly |
Automated Annotation | Scalability, Cost-Effectiveness | Precision Issues |
Hybrid Approach | Cost-Effective, Quality Control | Requires Coordination |
Best Practices for High-Quality Medical Data Annotation
In today's fast-paced world, ensuring the accuracy of medical data annotation is vital for AI model success. The market for data annotation is expected to skyrocket, growing at 26.5% annually until 2030. This growth will reach USD 5,331.0 million. Adopting best practices in data annotation is essential for improving AI system reliability. This makes this section critical for both decision-makers and tech professionals.
Training and Utilizing Expert Annotators
Investing in the training of medical annotators is key. These experts must deeply understand medical data complexities. They need extensive education, collaboration with healthcare professionals, and ongoing workshops to stay updated.
Properly trained annotators significantly boost dataset accuracy. This, in turn, enhances the performance of medical AI models.
Implementing Robust Review Processes
A structured annotation review process is vital for maintaining consistency and precision. Organizations must have clear annotation guidelines and standardized protocols. Regular reviews by subject matter experts help identify and correct errors, ensuring high-quality data.
Using dual-annotation techniques, where two annotators review the same data, further increases reliability. Managing the annotation workflow effectively is also critical. This includes balancing workload, using advanced tools, and integrating human oversight. Such practices improve project success rates and model accuracy, essential for staying competitive in AI-driven healthcare.
- Key Practices:
- Establishing clear guidelines
- Continuous training for annotators
- Dual-annotation techniques
- Regular review and feedback cycles
Best Practices | Benefits |
---|---|
Rigorous Training Programs | Ensures deep understanding of medical data nuances |
Structured Review Processes | Maintains consistency and accuracy across datasets |
Advanced Annotation Tools | Enhances workflow efficiency and model accuracy |
Clear Annotation Guidelines | Boosts reliability and reduces biases |
By adhering to these best practices, organizations can significantly improve AI model quality and reliability. This ensures superior healthcare outcomes.
The Impact of AI on Medical Data Annotation
The healthcare sector is rapidly evolving, making the use of AI in annotation processes more critical than ever. The integration of automation in data annotation has the power to transform medical data management and interpretation. This transformation could streamline healthcare delivery and improve diagnostic accuracy.
Automation vs. Manual Annotation
The shift towards automated annotation in medical data handling is significant. Automation offers advantages like increased speed and accuracy in processing large volumes of medical data. This reduces the administrative workload on healthcare professionals. High-quality annotated data is vital for training machine learning models. These models can detect early disease signs, like various cancers, and precisely identify dental issues such as decay.
Despite these benefits, the complexity and variability of medical data require human validation for accuracy. Automated methods excel in tasks like organizing electronic health records and improving radiology and pathology practices for disease detection. Yet, the expertise of trained professionals is essential for nuanced tasks, such as annotating complex surgical procedures or medical terminology.
The Future of AI in Healthcare Annotation
AI's role in annotation will continue to evolve, incorporating advanced technologies to reduce human intervention. This will enhance data processing speed and accuracy. For instance, AI-powered anomaly detection in health imaging has reached human physician levels of accuracy. AI is also being used to identify conditions like COVID-19 and breast cancer through thermal image annotation.
The future of AI in healthcare will depend on a balanced approach. It will combine the precision of automated systems with the expertise of human annotators. This synergy will ensure AI models accurately represent diverse demographics, diseases, and conditions.
Case Studies: Successful Applications of Medical Data Annotation
High-quality medical data annotation is revolutionizing healthcare by boosting the accuracy and speed of machine learning models. Through case studies in radiology imaging and clinical trials data management, we see the significant positive impact on patient care and outcomes. Precise data annotation is key to this transformation.
Radiology and Diagnostic Imaging
In radiology, AI models trained on detailed images spot subtle anomalies in X-rays, CT scans, and MRIs with high precision. This has achieved a "perfect accuracy level," thanks to advancements in medical annotation. Tools like Label Studio from HumanSignal support annotation for various imaging modalities, significantly boosting diagnostic accuracy and research efficiency. It has garnered 18.2k stars on GitHub for its medical image labeling capabilities.
Clinical Trials and Patient Records
Clinical trials data management also benefits greatly from high-quality data annotation. Annotated data is vital in tracking patient responses and ensuring the reliability of outcomes. Precise annotation of patient records simplifies managing vast data, allowing researchers to monitor progress and gain insights.
Medical annotation not only streamlines data collection but also enhances the accuracy and relevance of clinical trial results. For example, accurate annotation of patient records improves tracking of adverse effects and response patterns. This ensures more reliable and consistent results. The improvement in data quality, driven by AI and manual annotation, directly enhances trial efficiency and decision-making confidence.
"High-quality annotated data is fundamental to advancing both diagnostic imaging and clinical trial management, ultimately leading to superior healthcare delivery."
By using advanced medical annotation tools, researchers and healthcare professionals can ensure the quality and consistency of annotated data. This empowers AI applications to perform optimally, providing invaluable support in radiology imaging and clinical trials data management.
Selecting a Medical Data Annotation Service
In the rapidly changing world of medical data annotation, picking the right partner is key to achieving top results in AI model training. When choosing annotation partners, several factors must be considered. These include technological capabilities, experience in healthcare, and compliance with strict data protection regulations.
Factors to Consider for Choosing a Partner
Choosing the right annotation services for your project requires evaluating tools and service providers on various parameters:
- Compatibility: Make sure the tools support the required data formats. For instance, 3D Slicer and MITK are critical for handling DICOM files, which are widely used in radiology.
- Expertise: Choose partners who have deep experience in specific medical domains.
- Data Security: Strong data security compliance is essential. Partners must follow regulations like HIPAA to safeguard patient information.
- Scalability: The ability to scale operations to handle large volumes of complex data is vital. Technologies from companies like Keylabs stand out in this realm.
- Pricing Models: Clear and transparent pricing structures are important for accurate budgeting in long-term projects.
Comparing In-House vs. Outsourcing Options
The choice between in-house and outsourcing annotation is critical. In-house annotation offers direct control and consistent quality but requires significant investment in resources and training. Outsourcing to specialized firms like iMerit and Keymakr can be more cost-effective and scalable. It provides access to expert annotators and advanced annotation techniques.
- In-House Annotation: Suitable for organizations aiming to build long-term capabilities and maintain control over data processes.
- Outsourcing Annotation: Ideal for leveraging specialized expertise, reducing costs, and focusing on core competencies while ensuring scalability.
Choosing the right medical data annotation service requires balancing immediate needs with long-term strategic goals. By carefully evaluating the capabilities, experience, and scalability of annotation partners, organizations can make informed decisions. These decisions will enhance their AI model training processes.
The Future of Medical Data Annotation in Healthcare
The future of medical data annotation is set to transform with new technologies. Augmented reality (AR) and advanced AI algorithms are leading the way. These innovations aim to make annotations more precise and efficient. They will improve AI accuracy, which is key to better patient care by providing more reliable data.
These tools will also make healthcare workflows smoother. They will enhance the quality of services provided. This is a significant step forward for healthcare.
Emerging Technologies in Annotation
AR is a major leap in medical data annotation. It gives annotators immersive tools to work with complex data. This technology makes annotations more accurate by allowing real-time interaction with medical images.
AR, combined with AI, cuts down errors in data labeling. This is vital for training AI models. It leads to more accurate diagnoses and less human error in healthcare.
Predictions for the Next Decade in Medical AI
In the next decade, AI will automate annotation processes. AI systems will use large datasets to create accurate annotations with little human help. This change will make annotation faster and more effective.
AI will become essential in early disease detection and personalized treatments. It will also help in managing patient care proactively. This will revolutionize how we care for patients.
The future of medical data annotation is linked to technological progress. As AI and AR advance, annotation quality will improve. This will lead to better AI insights and healthcare outcomes.
FAQ
What is Medical Data Annotation?
Medical data annotation labels various medical data types—text, images, videos—to train AI systems. This process is key for developing algorithms that can predict, diagnose, and make decisions on their own.
Why Medical Data Annotation Matters?
It feeds accurate information into algorithms, driving advancements in diagnostics and treatment plans. This makes it vital for improving medical AI technologies.
How does Medical Data Annotation enhance Algorithm Accuracy?
Accurate data training leads to high algorithm accuracy. This is critical for early diagnosis and treatment recommendations.
How does Medical Data Annotation help in Reducing Bias in Machine Learning Models?
Diverse data sets reduce biases, ensuring fair healthcare delivery. This highlights the importance of data annotation in making healthcare models accurate and fair.
What is Image Annotation in Radiology?
Annotated images in radiology help AI distinguish between benign and malignant conditions. This enhances diagnostic accuracy.
What role does Text Annotation play in Clinical Records?
Text annotation makes parsing vast clinical records efficient. It aids in better disease tracking and patient management.
How is Video Annotation utilized in Surgical Procedures?
Annotated surgical videos train AI to assist or perform surgeries. This improves outcomes and precision in complex procedures.
What are the steps involved in the Annotation Process?
The process begins with collecting and preparing data. Then, the right tools are selected for annotation. Quality control and validation follow to maintain data integrity.
What are the Challenges in Medical Data Annotation?
Annotators face challenges like varying data quality and complex medical terminology. Balancing speed with accuracy is also a challenge.
What are the Best Practices for High-Quality Medical Data Annotation?
Best practices include training annotators in medical nuances and implementing review processes. This ensures consistency and accuracy in datasets.
How does AI impact Medical Data Annotation?
AI automates the annotation process, balancing human expertise with efficiency. Future advancements will further reduce human intervention, increasing speed and accuracy.
How have Radiology and Diagnostic Imaging benefitted from Medical Data Annotation?
AI trained on annotated images can detect nuances in X-rays and MRIs faster and more accurately. This advances diagnostic precision.
What is the role of Medical Data Annotation in Clinical Trials and Patient Records?
Annotated data in clinical trials tracks patient responses accurately. This increases trial reliability, essential for effective patient management and treatment evaluations.
What Factors should be considered when Selecting a Medical Data Annotation Service?
Consider technological capabilities, healthcare experience, and data protection compliance. Decide between in-house and outsourcing based on scalability, cost, and core competencies.
What is the Future of Medical Data Annotation in Healthcare?
Emerging technologies like augmented reality and advanced AI algorithms will shape the future. Predictions suggest a shift towards fully automated, highly accurate AI-driven annotation processes.
Comments ()