Future Trends and Developments in YOLO
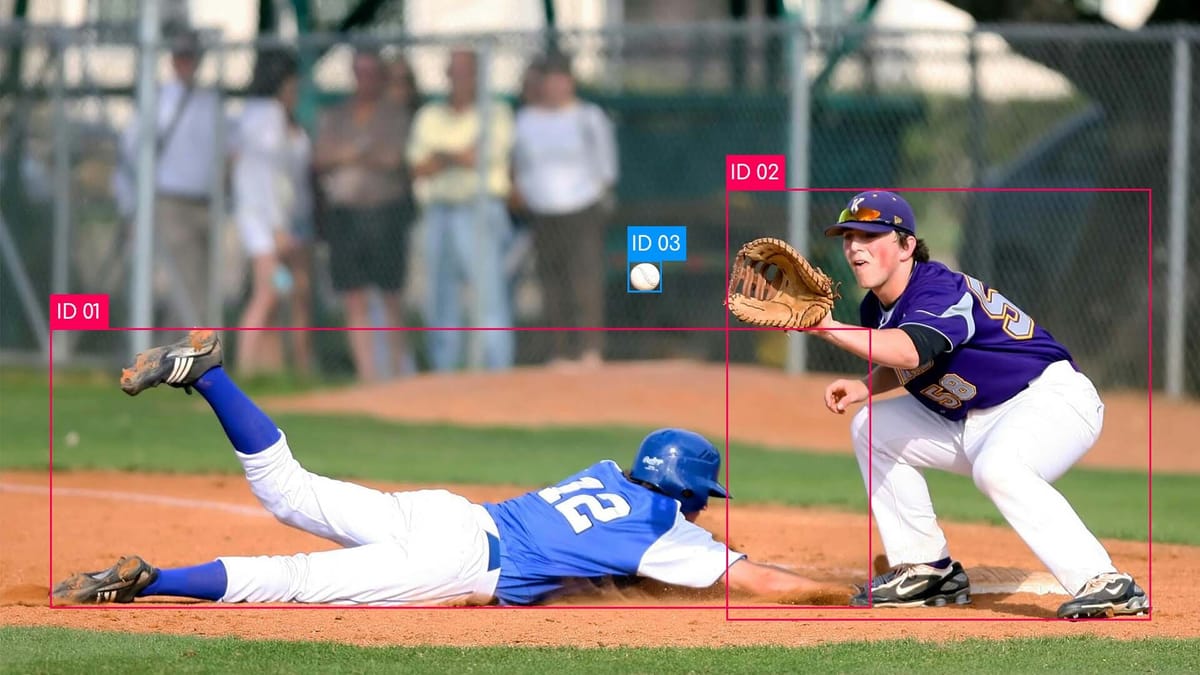
As we look ahead, the future of YOLO promises even more impressive advancements in real-time object detection and computer vision applications.
YOLO Vision 2024, an upcoming hybrid event scheduled for September, 2024, at Google for Startups Campus in Madrid, will showcase the latest YOLO features and object detection future. This gathering will bring together industry leaders and AI experts to discuss cutting-edge research, emerging trends, and real-world applications of Vision AI.
Key Takeaways
- YOLOv8 achieves 50.2 mAP at 1.83 milliseconds on COCO dataset
- YOLO Vision 2024 will showcase future trends in object detection
- Limited in-person attendance emphasizes high demand for YOLO insights
- Industry experts to discuss upcoming YOLO features and applications
- Event focuses on advancements in Vision AI and real-world implementations
The Evolution of YOLO: A Brief History
Since its debut in 2015, YOLO has undergone a remarkable transformation. Each version has significantly enhanced real-time object detection, reshaping the landscape of computer vision. Let's delve into the pivotal moments that have defined YOLO's journey.
From YOLOv1 to YOLOv10: Key Milestones
YOLOv1 marked the beginning with a 63.4 mAP at 45 FPS on Pascal VOC 2007. YOLOv2 introduced anchor boxes, elevating performance to 76.8 mAP at 67 FPS. YOLOv3 brought the Darknet-53 backbone into play. YOLOv4 then implemented Bag of Freebies and Bag of Specials, achieving 43.5 mAP at 65 FPS on MS COCO.
Improvements in Speed and Accuracy Over Time
YOLO's advancements in both speed and accuracy have been noteworthy. YOLOv5 introduced the CSP Connection block and YAML files. YOLOv6 featured anchor-free detection and a decoupled head. YOLOv7 employed the E-ELAN backbone and compound scaling. The latest YOLOv8 boasts enhanced accuracy and speed, thanks to its Python package and CLI-based implementation.
YOLO Version | Year | Key Features | Performance |
---|---|---|---|
YOLOv1 | 2015 | Single-stage detection | 63.4 mAP at 45 FPS |
YOLOv4 | 2020 | Bag of Freebies, Bag of Specials | 43.5 mAP at 65 FPS |
YOLOv8 | 2022 | Python package, CLI implementation | Improved accuracy and speed |
The Impact of YOLO on Real-time Object Detection
YOLO has revolutionized real-time object detection across various sectors. Its single-stage approach makes it ideal for edge device deployment. In manufacturing, YOLO facilitates efficient quality inspection, overcoming human limitations. The YOLOv7 paper's GitHub repository quickly gained over 4,300 stars, highlighting its significant impact on the field.
YOLO's rapid evolution has set new benchmarks in real-time object detection, paving the way for innovative applications in computer vision and beyond.
Current State of YOLO Technology
YOLO architecture has become a cornerstone in computer vision technology. The latest iteration, YOLOv8, offers cutting-edge performance for image and video analytics. It comes in five sizes, from nano to extra large, catering to diverse needs in classification, object detection, and segmentation tasks.
YOLOv8 employs advanced techniques like mosaic data augmentation and anchor-free detection. Its unique approach divides images into grids, predicting bounding boxes and class probabilities in a single forward pass. This enables real-time object detection, a crucial feature for many applications.
Let's look at some impressive statistics showcasing YOLO's capabilities:
Model | Performance Metric | Value |
---|---|---|
YOLOv3 | Mean Average Precision (COCO dataset) | 57.9% |
YOLO | Processing Time | 22 ms |
YOLO in Traffic Management | Top-1 Accuracy (Vehicle Detection) | 76.5% |
YOLO in Traffic Management | Top-5 Accuracy (Vehicle Detection) | 93.3% |
EfficientDet-D7 | AP (COCO test-dev dataset) | 55.1 |
These figures underscore YOLO's efficiency in various scenarios, from general object detection to specific applications like traffic management. The combination of speed and accuracy makes YOLO a preferred choice in many object detection algorithms.
Future Trends and Developments in YOLO
The YOLO algorithm is constantly evolving, with each new version introducing significant advancements. From YOLOv1 to YOLOv10, we've witnessed remarkable growth in design and training methods. These enhancements have greatly improved object detection, solidifying YOLO's role in modern computer vision
Future YOLO developments will focus on AI integration. Researchers are working to merge YOLO with natural language processing and reinforcement learning. This combination could result in systems that can understand and interact with their environment in real-time.
Advancements in Training Techniques
Training techniques are driving YOLO's evolution. New loss functions, optimization algorithms, and transfer learning methods are being developed. These innovations are vital for overcoming challenges like detecting small objects and handling occlusions.
The future of YOLO is bright, with ongoing research aimed at enhancing object detection performance. As AI integration and training techniques advance, YOLO will play an increasingly vital role in various fields. This includes autonomous vehicles and precision agriculture.
Emerging Applications of YOLO in Various Industries
YOLO applications are transforming numerous industries with their real-time object detection capabilities. From agriculture to wildlife conservation, YOLO's versatility is evident in various industry use cases. Let's delve into how YOLO is reshaping different sectors.
In agriculture, UAVs with YOLO algorithms are making a significant impact. These aerial wonders use YOLO for crop monitoring, disease detection, and weed management. Farmers now have a powerful tool for sustainable farming, thanks to YOLO's keen eye in the sky.
Forest management also benefits from YOLO's quick thinking. YOLO models excel at real-time fire localization, proving invaluable in forest fire detection and analysis. This rapid response capability is crucial for protecting our green spaces.
Wildlife conservation gets a boost from YOLO-powered UAVs. These systems enhance monitoring efforts, allowing researchers to track animal populations and movements with unprecedented accuracy. Marine scientists also leverage YOLO for underwater surveys and resource management.
The transportation sector isn't left behind. YOLO's object detection implementation aids in traffic monitoring and autonomous vehicle navigation. Its ability to process complex imagery in real-time makes it a game-changer for smart city initiatives.
- Sports analytics: YOLO tracks player movements and ball trajectories
- Retail: Inventory management and customer behavior analysis
- Security: Enhanced surveillance and threat detection
As YOLO continues to evolve, its applications across industries grow more sophisticated. From YOLOv3 to the lightweight YOLOv8, each iteration brings new possibilities. The open-source nature of YOLO fuels innovation, inspiring both academic research and industrial applications to push the boundaries of visual recognition technologies.
YOLO and Edge Computing: A Powerful Combination
The integration of YOLO with edge computing is transforming real-time object detection. This synergy brings object recognition closer to the data source. It offers substantial benefits for IoT devices and smart systems.
Benefits of Running YOLO on Edge Devices
Edge computing allows YOLO deployment on local devices, reducing latency and enhancing privacy. Real-time processing becomes a reality, with decreased bandwidth usage and offline capabilities. In smart cities, edge-based YOLO can identify traffic violations instantly, improving safety and efficiency.
Challenges and Solutions for Edge Deployment
Edge deployment faces hurdles like limited computational resources and power constraints. YOLOv5 addresses these challenges with various model sizes, from nano to extra-large, catering to different needs. It achieves impressive performance, with 37.4 mAP on COCO dataset and 6.4ms inference time on V100 GPU.
Future Possibilities in IoT and Smart Devices
The future of YOLO in IoT devices is promising. YOLOv8's anchor-free detection enhances small object recognition, crucial for IoT applications. Edge computing with YOLO opens doors for advanced object recognition in smart homes, wearables, and industrial settings.
Feature | Benefit |
---|---|
Local Processing | Reduced Latency |
Offline Capabilities | Improved Reliability |
Bandwidth Optimization | Cost Reduction |
Privacy Enhancement | Data Security |
Advancements in YOLO's Object Detection Capabilities
YOLO's object detection improvements have transformed computer vision. The latest version, YOLOv8, marks significant strides in both YOLO accuracy and detection speed. It scores a 50.2 mAP at just 1.83 milliseconds on the COCO dataset. This achievement sets new standards in real-time object detection.
One major advancement is the introduction of anchor-free detection. This feature boosts the model's versatility across various scenarios. It also reduces the number of bounding box predictions. This results in enhanced efficiency without sacrificing accuracy.
The C2f module in YOLOv8's backbone is another significant improvement. It combines outputs from all bottleneck modules, improving feature extraction. This leads to better performance in object detection tasks, especially for identifying smaller objects.
These advancements make YOLO a leader in real-time object detection. Its ability to process visual data quickly is crucial for applications needing instant decisions. Examples include autonomous vehicles and security systems.
"YOLO's evolution has simplified object detection by formulating localization as a regression problem using deep neural networks."
The effects of these improvements go beyond just speed and accuracy. YOLO's enhanced capabilities are driving innovation across various industries, from retail to healthcare. As object detection technology continues to evolve, we can look forward to even more exciting applications in the future.
YOLO in Computer Vision: Beyond Object Detection
YOLO's influence on computer vision goes beyond object detection. It's expanding into image segmentation, multi-task learning, and video analysis. These developments are transforming how we process and understand visual data in various sectors.
Expansion into Image Segmentation
Image segmentation moves beyond object detection by identifying objects at a pixel level. YOLOv8 now includes segmentation, allowing for more detailed object identification. This breakthrough is opening new avenues in medical imaging, autonomous driving, and robotics.
Potential for Multi-task Learning
YOLO's design is well-suited for multi-task learning. It can perform detection, segmentation, and classification all at once. This efficiency is key for complex computer vision tasks requiring real-time analysis.
YOLO's Role in Video Analysis
YOLO excels in video analysis. Its fast processing allows for real-time object tracking and event detection. This capability is revolutionizing surveillance, sports analytics, and traffic monitoring.
Application | Image Segmentation | Multi-task Learning | Video Analysis |
---|---|---|---|
Medical Imaging | Tumor detection | Diagnosis & prognosis | Surgical assistance |
Autonomous Driving | Road segmentation | Object detection & classification | Real-time navigation |
Retail | Product recognition | Inventory management | Customer behavior analysis |
As YOLO evolves, its applications in image segmentation, multi-task learning, and video analysis will transform industries. It will redefine the limits of computer vision.
Ethical Considerations and Privacy Concerns
As YOLO technology advances, the AI ethics landscape becomes increasingly complex. The rapid progress in object detection capabilities brings forth critical privacy concerns that demand attention. A prime example is the use of YOLO in the Architecture, Engineering, and Construction, where real-time monitoring raises questions about worker privacy.
Joseph Redmon, YOLO's creator, highlighted these issues when he stopped his computer vision research. He cited military applications and privacy concerns as key reasons, sparking a debate on responsible AI development.
The AI community is now grappling with the ethical implications of their work. NeurIPS 2020 required authors to address societal consequences in their papers, signaling a shift towards more responsible AI practices.
"I felt like I was enabling the construction of a surveillance state," Redmon stated, emphasizing the need for ethical considerations in AI research.
To address these concerns, researchers are exploring new philosophies:
- YDLO (You Don't Live Once): Promotes thoughtful decision-making in AI, considering long-term impacts.
- YXLO: Aims to balance immediate efficiency with long-term societal welfare in AI systems.
These approaches strive to steer AI development towards ethical, sustainable, and socially responsible practices. As YOLO continues to evolve, integrating these ethical frameworks will be crucial for maintaining privacy in object detection while advancing the field responsibly.
The Role of YOLO in Autonomous Systems
YOLO's rapid object detection has transformed autonomous systems. It can process up to 45 frames per second, outpacing older methods like R-CNN. This speed is essential for autonomous vehicles, enabling them to identify pedestrians, other vehicles, and obstacles in real-time.
In robotics, YOLO boosts machine vision for tasks like object manipulation and navigation. It has evolved to overcome initial challenges, such as detecting small or unusual objects. YOLO V2 enhanced accuracy by increasing input image size and dividing images into a 13x13 grid. YOLO V3 introduced logistic regression for more precise bounding box prediction.
The latest version, YOLO NAS, excels in real-time object detection. Its high accuracy-speed performance is perfect for surveillance and healthcare. As computer vision in automation advances, YOLO's importance in enhancing safety and efficiency in autonomous systems becomes more critical.
FAQ
What are some upcoming YOLO future trends and features?
YOLO's future may include addressing small object detection and occlusion handling. New network architectures, training methods, and data augmentation are being explored. Integration with AI technologies like natural language processing and reinforcement learning could enhance its capabilities.
How has YOLO evolved over time in terms of speed and accuracy?
YOLO has significantly improved in speed and accuracy. YOLOv1 achieved 63.4 mAP at 45 FPS on Pascal VOC 2007. YOLOv2 reached 76.8 mAP at 67 FPS. YOLOv4 achieved 43.5 mAP at 65 FPS on MS COCO. YOLOv8(medium) now scores 50.2 mAP at 1.83 milliseconds on COCO.
What are the current state-of-the-art YOLO architectures and algorithms?
YOLOv8 is the latest model, offering top results for image and video analytics. It has five variants, using mosaic data augmentation and a C2f module. This results in improved accuracy and speed.
What are some potential advancements in YOLO's training techniques?
Future advancements may include better loss functions, optimization algorithms, and transfer learning. These could boost performance and generalization.
In which industries is YOLO being applied, and what are some use cases?
YOLO is used in autonomous vehicles, traffic monitoring, surveillance, sports analytics, and wildlife monitoring. Its real-time detection makes it ideal for fast processing needs.
What are the benefits and challenges of running YOLO on edge devices?
Running YOLO on edge devices offers real-time processing and reduced bandwidth usage. However, it faces challenges like limited resources and power constraints. Solutions like model compression and hardware acceleration are needed.
How has YOLO's object detection capabilities improved in recent versions?
Recent YOLO versions have seen better accuracy and speed. YOLOv8(medium) scores 50.2 mAP at 1.83 milliseconds on COCO. It uses anchor-free detection and the C2f module for enhanced performance.
How is YOLO expanding beyond object detection?
YOLO is expanding into image segmentation, enabling pixel-level object identification. YOLOv8 offers segmentation capabilities with the -seg suffix. It also has potential for multi-task learning and video analysis, enabling real-time tracking and event detection.
What are some ethical considerations and privacy concerns surrounding YOLO technology?
Concerns include potential misuse, bias in training data, and the need for transparent AI. Developing ethical guidelines and ensuring data privacy are crucial. Promoting responsible AI practices is also essential.
How is YOLO being utilized in autonomous systems like self-driving vehicles and robotics?
YOLO is vital in autonomous systems for quick object detection. In robotics, it enhances machine vision for tasks like object manipulation and navigation. This improves safety and efficiency in automation.
Comments ()