Future Trends in Classification Models: AutoML and XAI
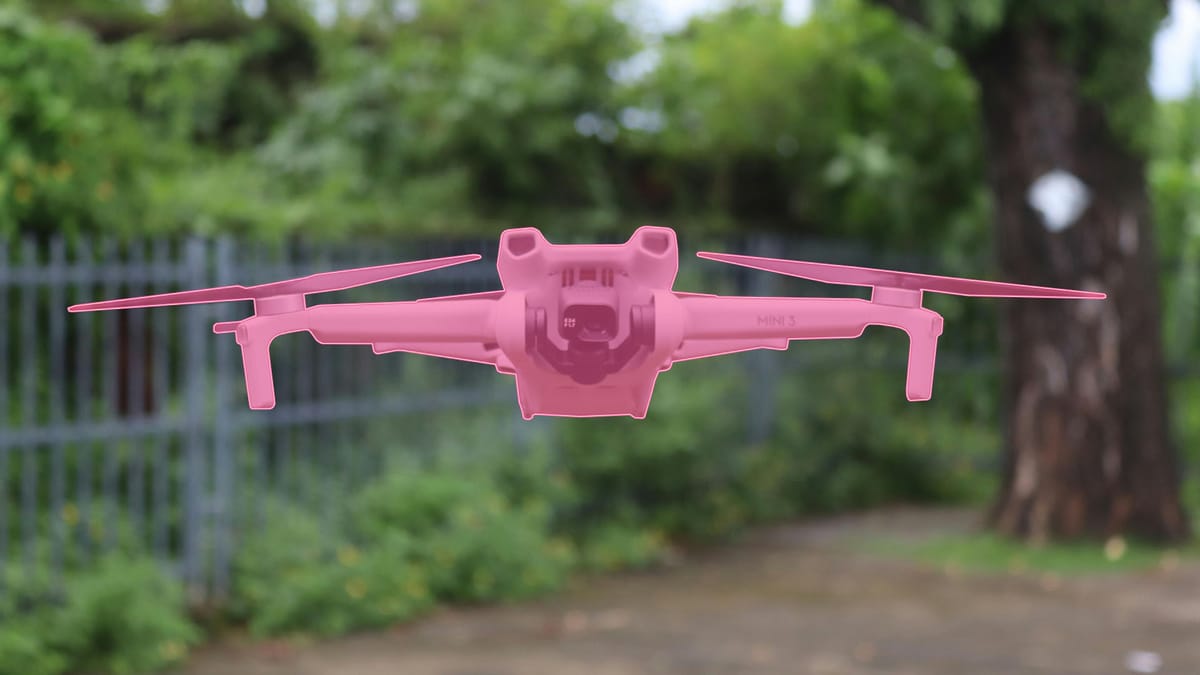
Predictive analytics is more than just a buzzword. It's a key part of many business operations, helping with forecasting sales, monitoring customer churn, and assessing risks. The pace of machine learning algorithms is incredible, with the future of classification models being shaped by trends like automated machine learning (AutoML) and explainable AI (XAI).
The field of machine learning algorithms is undergoing a significant transformation. AutoML is making complex modeling more accessible, while XAI ensures AI's decisions are transparent and understandable. These advancements are vital across various sectors, meeting the need for systems that are both intelligent and trustworthy.
Key Takeaways
- AutoML unlocks machine learning for non-experts, boosting efficiency and scalability.
- Explainable AI is key for making AI's decisions understandable and trustworthy.
- Embracing new ML trends can greatly enhance industry efficiency and compliance.
- AI and ML integration into data analytics marks a significant shift in data science.
- Certifications in AI could be a major step to keep up with the future of classification models.
The Increasing Importance of Automated Machine Learning (AutoML)
In the rapidly evolving tech world, Automated Machine Learning (AutoML) stands out as a key player. It not only drives future trends in machine learning but also boosts industry efficiency and promotes the democratization of AI. This tech simplifies complex tasks, enabling organizations to tap into AI's power without needing extensive data science knowledge.
What is AutoML and its Role in Future Trends
AutoML automates the process of choosing, training, and deploying machine learning models. It streamlines data tasks, making AI more accessible and scalable across different industries. The field has seen a significant surge in research and practical applications, showing its growing importance and ability to reshape the market.
How AutoML Democratizes Data Science for Businesses
AutoML democratizes AI, making data science accessible to more businesses. Companies of all sizes can use AutoML to improve operations and make informed decisions quickly. This shift empowers existing staff to perform complex analytics, reducing dependence on specialized data scientists.
Case Studies: AutoML's Impact on Industry Efficiency
PayPal's use of AutoML is a clear example of its ability to enhance industry efficiency. Their fraud detection models saw a notable improvement. Marketing and sales teams also see significant gains, with about 50% of senior professionals already using AI and another 29% planning to adopt it. These examples highlight AutoML's role in driving innovation and efficiency across various sectors.
As AutoML continues to evolve, its role in democratizing AI and improving industry efficiency is undeniable. Its integration into different fields not only streamlines processes but also fosters a more inclusive adoption of advanced technologies.
Explainable AI (XAI): Bridging the Gap Between Human and Machine Interpretation
Explainable AI (XAI) is vital in sectors where AI's decisions have major impacts. It ensures these decisions are clear and fair.
The Necessity of Transparency in AI Decision-Making
Transparency in AI means its workings and decisions can be checked and must follow ethical guidelines. This is key in fields like healthcare, finance, and law. XAI boosts trust and compliance, lowering risks tied to AI choices.
Implementing XAI in Regulated Industries for Compliance and Trust
In regulated fields, XAI is essential for compliance and trust. It uses methods to make complex AI models easier to understand. Techniques like feature importance graphs and model visualization help explain AI decisions.
XAI showcases the clear logic behind AI models like decision trees and linear regression. It proves AI's value through surrogate models and decision rules. This is critical in healthcare and finance, where AI's decisions affect people's lives and must meet strict rules.
Evolution of Real-Time Data Processing in Classification Models
The field of machine learning has revolutionized how industries manage vast data sets. Real-time data processing is now essential for making quick, effective decisions. Through data streaming, classification models can now predict outcomes instantly. This enables businesses to respond more swiftly to changing market conditions.
In finance, for example, immediate data analysis is vital for high-stakes trading and fraud detection. Every millisecond counts, as it can lead to significant gains or losses. In e-commerce, real-time models adjust prices and offers based on current demand and consumer behavior. This improves customer satisfaction and boosts business performance.
Technique | Number of Articles | Application Area |
---|---|---|
Time Series Methods | 77 | Biomedical Applications |
Statistical Modeling | 24 | Various Domains |
Machine learning's integration into real-time data processing brings both speed and precision. It leverages structured and unstructured data to provide detailed insights. The ability of classification models to adapt to diverse data streams is key to their success.
The Internet's rapid growth, driven by IoT devices and digital interactions, demands advanced data streaming techniques. These must capture and analyze data in real-time, adjusting to new information continuously. This environment requires robust, evolving classification models in machine learning to meet industry demands.
A five-year review of scholarly articles highlights a shift towards using time series methods in biomedical applications. This evolution emphasizes the need for proficient data handling and continually improving classification models. They must effectively foresee and respond to real-time scenarios.
The evolution of real-time data processing through machine learning and classification models is transformative. It offers a pathway for industries to leverage immediate data insights for better decision-making and strategic advantage.
Future Trends in Classification Models
The evolution of classification models in data analytics is driven by significant technological advancements. Multimodal learning and federated learning are key, improving model performance and protecting privacy. The integration of AI ethics ensures these models are developed and deployed ethically. This is essential for building trust and fairness. The need for industry-specific AI applications across various industries highlights the importance of tailored solutions.
Multimodal learning transforms sectors like healthcare and automated driving by integrating text, video, and audio data. This is critical for accurate decision-making. Federated learning offers a solution for data privacy and security, training algorithms collaboratively without sharing data. This is invaluable for organizations handling sensitive information, ensuring compliance with global data protection regulations.
Addressing AI ethics is vital as organizations aim to eliminate bias and ensure fairness in automated decisions. This ethical focus is essential for maintaining public trust and legal compliance, critical in regulated industries like finance and healthcare. The rise of industry-specific AI applications indicates a trend towards customized solutions, boosting operational efficiency and innovation.
Businesses must adopt advanced strategies and tools to remain competitive. Below is a table outlining current classification model techniques and their relevance in different industries:
Model/Technique | Industry Application | Importance |
---|---|---|
Multimodal Learning | Healthcare, Automotive | Increases accuracy of diagnostics and decision-making |
Federated Learning | Banking, Healthcare | Enhances privacy and compliance with data protection laws |
AI Ethics | Human Resources, Loan Approvals | Builds trust and fairness in automated systems |
Industry-specific Applications | Retail, Manufacturing | Tailors AI solutions to specific operational needs |
As these trends evolve, keeping up with the latest techniques in model building and data analysis is critical. This ensures your organization maximizes AI's benefits, addressing operational, ethical, and competitive challenges effectively.
Challenges and Ethical Considerations in Advancing AutoML and XAI
The integration of AutoML and XAI in healthcare faces unique challenges and ethical dilemmas. Ensuring ethical AI practices and maintaining data quality are essential for robust machine learning outcomes. This is critical in medical diagnostics, where AI's decisions can greatly impact patient care.
Overcoming Data Quality Issues for Robust Machine Learning
Ensuring data quality is a major AutoML challenge. High-quality data is vital for training effective and reliable algorithms. In healthcare, where patient care is at stake, data integrity and accuracy are non-negotiable. Understanding variables that influence critical conditions is key. Enhancing data quality is necessary to meet healthcare's rigorous standards.
Ensuring Ethical Use of Advanced Classification Models
Deploying AI systems ethically requires ongoing oversight and rigorous testing to avoid biases. Regulations like the GDPR emphasize the need for AI systems that comply with privacy laws and provide transparent explanations. This is essential in healthcare, where understanding AI-generated diagnoses or treatment plans is vital.
Creating systems that support explainability from the start is critical for ethical AI in healthcare. Embedding interpretability into AI's core ensures transparent, understandable, and trustworthy applications. For instance, visualizations and logical frameworks help demystify AI decisions for medical professionals, ensuring they align with clinical needs and patient safety.
Here's a detailed table showing the growth and importance of XAI in medicine:
Year | Focus Area | Article Count | Regulation Compliance |
---|---|---|---|
Early 2000s | Initial Publications | Minor | N/A |
2017-Present | Exponential Growth | 48,060 | GDPR, EGDPR Compliance |
Continuous | Medical AI | Varies by study | Medical domain-specific regulations |
Integration of AI and ML in Data Analytics: The Road Ahead
The digital age is witnessing a significant shift with the merging of artificial intelligence (AI) and machine learning (ML) with data analytics. This fusion is transforming business and technology landscapes. It's empowering automated decision systems and boosting predictive analytics, setting new standards for operational excellence.
Predictive analytics stands at the forefront of this evolution, leveraging ML algorithms to analyze vast data sets. These systems are dynamic, evolving based on data, algorithms, and predictions. They help businesses forecast trends, manage risks, and personalize customer experiences.
The impact of AI and ML on these capabilities is immense. With data generation soaring to 2.5 quintillion bytes daily, AI-driven insights are limitless. The data analytics market is expected to reach USD 307.52 billion, highlighting the significant role of AI and ML integration.
Yet, challenges persist. Data privacy, the intricacy of ML models, and the need for transparent algorithms require innovative solutions. The integration of AI in predictive analytics promises better decision-making but also demands ethical standards and ongoing learning.
Component | Description | Impact |
---|---|---|
Data Quality | Ensuring clean, relevant data for analysis. | Improves accuracy of predictions. |
ML Algorithms | Advanced algorithms including Neural Networks, Decision Trees. | Enables handling complex data sets effectively. |
Predictive Models | Forecasting trends, classifying data points. | Assists in proactive decision-making and strategy formulation. |
Ethical AI | Emphasis on transparency and privacy in AI operations. | Builds trust and ensures compliance with regulations. |
The integration of AI and ML in data analytics is more than an enhancement; it's a transformation of business processes and decision-making frameworks. As this integration deepens, predictive analytics will become even more precise, marking the beginning of an era of unparalleled precision in automated decision systems.
Edge Computing and ML at the Edge: Redefining Data Accessibility
In today's digitally driven landscape, the convergence of edge computing and machine learning at the edge is transforming data use for real-time insights. Edge computing decentralizes data processing, bringing it closer to data sources. This significantly enhances data accessibility and reduces latency, creating a robust platform for classification at the edge.
Industries like healthcare, automotive, and urban planning benefit from edge computing's rapid data analysis. This is critical for operational efficiency. Applications needing immediate response, such as medical devices and autonomous vehicles, rely on edge computing for timely and accurate results.
Edge Computing's Role in the Future of Classification Models
The integration of machine learning at the edge enables nuanced and dynamic classification models. These models adapt and respond quickly, a key advantage in environments where timing and precision are critical.
This shift boosts data accessibility and enhances device capabilities for complex classifications without central servers. Such advancements herald a new era of intelligence distributed across the edge, reducing centralized system burdens and minimizing data transit delays.
Use Cases: ML Applications Thriving on the Edge
Real-world applications of machine learning at the edge are abundant and growing. From predictive maintenance in manufacturing to real-time traffic management in smart cities, the impact is profound and wide-ranging. These advancements enable devices to learn and act without incessant reliance on distant cloud infrastructures, optimizing performance and functionality.
Examining edge computing's evolution and use across sectors reveals its transformative effects on data accessibility and machine learning applications. For a deeper dive into optimizing machine learning models at the edge, refer to this detailed paper on methodologies and future trends in edge learning.
Technology | Impact on Data Accessibility | Reduction in Latency (%) |
---|---|---|
Edge Computing in Predictive Maintenance | Enables timely data processing near source, increasing operational uptime | 45 |
Real-Time Traffic Management | Improves traffic flow and safety by immediate data assessment | 30 |
Healthcare Monitoring Devices | Allows for immediate patient data analysis, critical for urgent care | 75 |
As edge computing evolves, it is set to redefine industry landscapes by making data more actionable and machine learning more integral to local systems. The innovation possibilities are endless, as edge computing enables smarter, more responsive technology solutions. These are underscored by enhanced data accessibility and efficient decision-making at the edge.
Federated Learning: A Step Towards Secure and Collaborative AI
The surge in data breaches, nearly tripling from 2013 to today, has highlighted the need for secure AI. Federated learning emerges as a key solution, fostering collaborative machine learning while safeguarding data privacy. It allows for the creation of machine learning models on decentralized devices, reducing risks associated with centralized data storage. This approach is vital in sectors like healthcare and finance, where data sensitivity is critical.
The market for federated learning is expected to grow significantly, from $128.3 million in 2023 to $260.5 million by 2030. It's not just a trend; it's becoming essential for cybersecurity strategies in various industries. From smart retail to healthcare and finance, federated learning is gaining traction. The cybersecurity community is also working to establish standards, ensuring consistency in federated learning across different setups. This will encourage more widespread adoption of collaborative learning from IoT sources.
The importance of secure AI through federated learning is underscored by major breaches like those at Equifax, Marriott, and eBay, affecting nearly 800 million customers. Federated learning addresses these security issues head-on, prioritizing data privacy and distributed data processing. The growth in federated learning research, as shown by CiteSpace and Web of Science, highlights its role in the AI landscape. It promotes transparency, consent, and data minimization, making it a strategic asset for secure and collaborative AI networks.
FAQ
What are the future trends in classification models?
The future of classification models will see the integration of AutoML and XAI. We'll also see more multimodal and federated learning. There will be a focus on ethical AI and the development of AI for specific industries. Advances in quantum computing and edge computing will make models faster and more accessible, ensuring privacy and reducing latency.
How is AutoML changing the landscape of machine learning?
AutoML is transforming machine learning by automating the entire process. It empowers non-experts and enhances the work of professionals. It simplifies tasks like data preprocessing, model selection, and hyperparameter tuning. This democratizes data science, making it accessible to more people and speeding up innovation across industries.
What role does XAI play in regulated industries?
XAI is essential in regulated industries for its role in AI transparency. It ensures AI decisions are understandable and trustworthy. This is critical for compliance and maintaining public trust in sectors like healthcare, law, and finance.
How does real-time data processing improve classification models?
Real-time data processing boosts classification models by enabling instant analysis and decision-making. It's vital for applications needing immediate responses, like financial trading and fraud detection. Processing streaming data in real-time enhances AI systems' responsiveness and effectiveness.
What impact could quantum computing have on machine learning?
Quantum computing could revolutionize machine learning, speeding up data processing. Quantum algorithms are faster, potentially accelerating machine learning model training. This could solve complex problems currently challenging for classical computers.
Why are ethical considerations important in the advancement of AutoML and XAI?
Ethical considerations are key to address AI's risks of bias and accountability. Ensuring AutoML and XAI's ethical use involves careful design, extensive testing, and ongoing scrutiny. This prevents abuses and ensures fairness in AI applications.
What is the significance of integrating AI and ML into data analytics?
Integrating AI and ML into data analytics transforms data interpretation and use. It leads to automated decision systems powered by predictive analytics. These systems make accurate forecasts and guide strategic decisions, improving business performance and customer experience.
What is edge computing and how does it affect machine learning?
Edge computing brings computation closer to data sources, reducing latency and improving privacy. In machine learning, it enables real-time data processing on edge devices. This is critical for applications needing immediate action, like in healthcare and smart cities.
How does federated learning contribute to a more secure AI?
Federated learning trains machine learning models on decentralized devices, not a central server. This approach maintains data privacy and reduces breach risks. It fosters collaborative AI development while keeping sensitive data secure and localized.
Comments ()