Getting Started: Data Annotation Jobs for Beginners
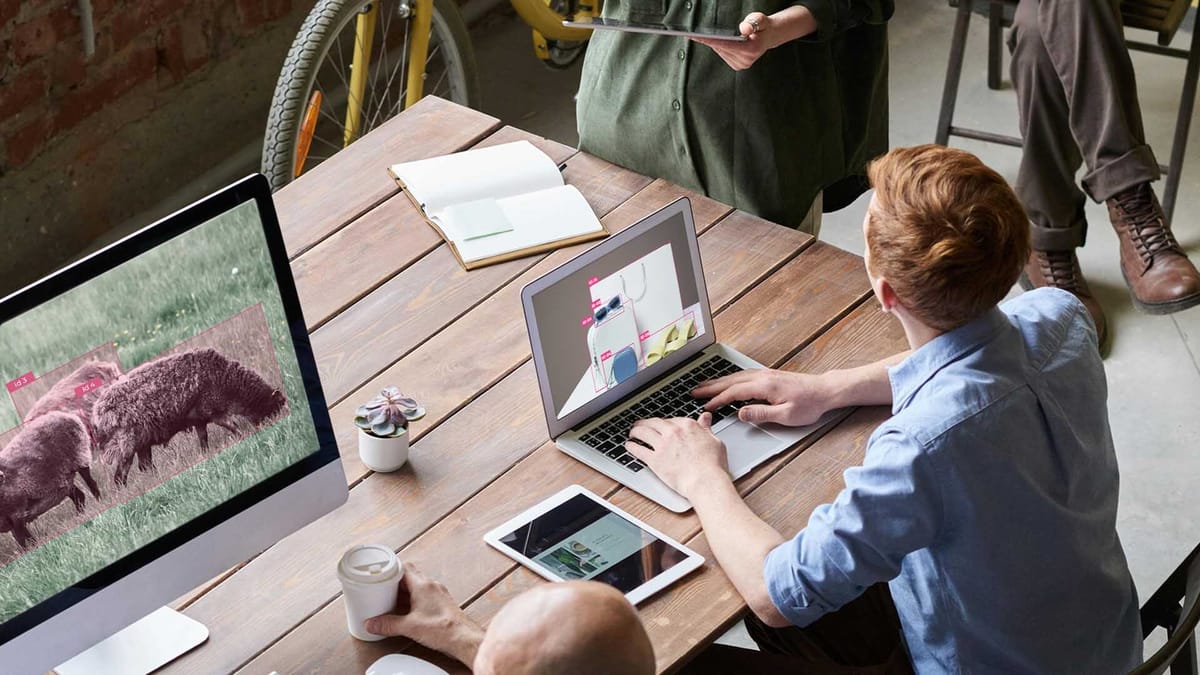
Over 11,070 entry-level data annotation jobs became available in the first 6 months of 2024. These jobs span various commitment levels, from full-time to volunteer work. The rise of AI and machine learning has made data annotation more accessible, even for those without prior experience.
Data annotation offers a pathway into the tech industry without needing a formal degree. Commonly referred to as entry-level data annotation tasks, these jobs provide foundational skills and critical experience. This prepares you for more advanced roles in the future.
Platforms like Lionbridge and Appen specialize in offering a wide range of data annotation jobs for beginners. From image labeling to text annotation, there's an array of tasks you can perform. These tasks help improve AI systems. Additionally, Amazon Mechanical Turk (MTurk) provides diverse earning opportunities by allowing you to choose from various annotation tasks based on your preference and skill level.
Pursuing entry-level data annotation tasks can lead to a reliable income. It can even open doors to specialized roles in the AI field. The industry values skills such as attention to detail, basic computer knowledge, and an understanding of AI and machine learning basics.
Key Takeaways
- Over 11,070 entry-level data annotation jobs are currently available.
- Entry-level data annotation tasks offer various commitment levels, from full-time to volunteer work.
- Platforms like Lionbridge, Appen, and Amazon Mechanical Turk provide diverse earning opportunities.
- Skills in attention to detail, basic computer knowledge, and understanding AI and machine learning basics are crucial.
- Data annotation jobs are a great way to break into the tech industry without needing a formal degree.
Introduction to Data Annotationms for Data Annotation Jobs
Data annotation is vital for artificial intelligence (AI) as it provides the foundation for machine learning models. These models learn from accurately labeled data, which is crucial for their ability to understand the world. Data annotators play a pivotal role in this process, ensuring AI systems can efficiently perform tasks such as object classification in images or transcribing audio.
The demand for precise and detailed data labeling for machine learning has led to significant financial rewards. Entry-level positions pay between $10 to $20 per hour, while specialized roles can reach $70+ per hour. In the United States, a Machine Learning Data Operations Lead can earn an annual salary between $117,000 to $350,000, highlighting the value of expertise. The higher end, of course, require running an entire department of labelers.
Freelancers in data annotation enjoy flexible schedules and the freedom to choose their projects. Compensation ranges from $5 per hour for beginners to $65 per hour for experts in specialized areas like niche medical imaging. This flexibility and the high demand for quality annotation work create substantial opportunities for skilled individuals.
Statistics show a promising outlook for those entering the field. Over 100,000 people worldwide have found success in data annotation, making it a viable career path. The growing recognition of the need for accurate data labeling for machine learning is reflected in better pay and benefits for those with advanced skills.
Data annotators handle a variety of tasks, including image labeling, video marking, and transcription. This diversity keeps the work engaging and supports the training of AI models for applications such as object detection in agriculture and facial recognition technology.
Attention to detail is crucial for data annotators. Precise labeling ensures AI and machine learning models receive reliable data, improving their performance. Roles such as an AI data trainer and those involved in AI evaluation are vital for developing robust AI systems capable of solving real-world problems.
The data annotation field is expanding, offering numerous opportunities for freelancers and employed professionals. As businesses increasingly rely on AI for innovation, the need for high-quality data annotation will grow. This opens new doors for both new and experienced data annotators.
Essential Skills for Data Annotation Tasks
To excel in data annotation tasks, various fundamental skills are required. These skills ensure that you can execute data preprocessing tasks, sentiment analysis labeling, and contribute significantly to machine learning projects.
Attention to Detail
Attention to detail is paramount for data annotators. Accuracy and consistency are crucial, especially when labeling data points for machine learning models. Whether you are involved in image labeling, text annotation, or video marking, small errors can accumulate, impacting the model's performance. Maintaining high precision ensures the annotations meet the required standards, contributing to the development of reliable AI technology.
Basic Computer Skills
Basic computer skills are indispensable for navigating various annotation tools and platforms effectively. These skills include proficiency in SQL, keyboarding skills, and the ability to use programming languages. Freelance data annotators, for example, depend on these capabilities to manage their workloads and maintain productivity. For those looking to start a career in data annotation, mastering these fundamental computer skills is essential.
Understanding AI and Machine Learning Basics
Understanding AI and machine learning basics is another critical skill for data annotators. This knowledge allows you to perform data preprocessing tasks and sentiment analysis labeling with insights into how the annotated data will be used in training AI models. By grasping these concepts, you can produce annotations that enhance the quality and effectiveness of the AI models being developed.
Popular Platforms for Data Annotation Jobs
In the dynamic world of AI, numerous data annotation platforms offer substantial opportunities for individuals involved in computer vision annotation projects. Platforms like Lionbridge, Appen, and Amazon Mechanical Turk (MTurk) stand out, each providing unique advantages tailored to diverse industry needs.
Lionbridge supports a community of 1 million remote workers, offering competitive starting rates for data annotation tasks worldwide. It is a favored option for many due to its extensive support network and ease of access. Appen connects individuals to a variety of crowdsourcing annotation tasks, enhancing skills in data labeling for computer vision, textual data, and beyond.
Platforms like Clarifai are crucial in computer vision annotation projects, offering essential tools for data scientists, developers, and enterprises. Their comprehensive solutions cover the AI lifecycle through annotation software, AI data management, project management, quality assurance, and task automation. For deeper insights, consider reviewing resources like LabelVisor, which provides detailed overviews of high-demand remote data annotation roles.
Platform | Key Statistics |
---|---|
Lionbridge | 1 million remote workers, competitive global rates |
Clickworker | Growth from 150,000 to 2 million workers |
Super.AI | AI and human collaboration in data labeling |
Cloudfactory | Focus on social impact, aiming to connect one million workers |
Clarifai | Leads in computer vision AI solutions for various users |
Whether you're a novice or an expert in data annotation, utilizing these platforms can significantly enhance your career in AI. It's essential to select the right data annotation platforms and participate in crowdsourcing annotation tasks to maximize your impact and earnings in this evolving field.
Types of Data Annotation Tasks
Data annotation tasks are crucial for training machine learning models. They cater to various skills and interests, offering image tagging opportunities, audio transcription jobs, text annotation services, and video annotation work. These tasks ensure accurate and reliable model training. Here are the primary types of data annotation tasks:
Image Labeling
Image labeling involves tasks like drawing bounding boxes, segmenting images, and labeling specific parts for context. These tasks are vital for distinguishing between objects, such as cats and dogs. Tools like Labelbox and CVAT are frequently used for image tagging opportunities.
Text Annotation
Text annotation services are crucial for applications like chatbots and natural language processing (NLP). Annotators label phrases, parts of speech, and other elements to train AI models to understand and generate human-like text. Both manual and automated annotation tools can effectively handle these tasks.
Audio Transcription
Audio transcription involves transcribing spoken words into text, which is essential for speech recognition systems. This task is a significant part of audio transcription jobs and requires a keen ear for accuracy. Platforms like Amazon SageMaker Ground Truth can assist with these tasks.
Video Annotation
Video annotation work includes labeling actions and events within a video frame. It's crucial for applications such as autonomous driving and surveillance. This task often employs tools that allow annotators to label each frame accurately, ensuring robust model training.
As you delve into data annotation, platforms like Upwork and TEKsystems offer numerous remote work opportunities. Whether you're interested in machine learning or AI, data annotation opens the door to various career paths.
Getting Started with Data Annotation Jobs for Beginners
Starting data annotation jobs for beginners is an exciting and rewarding journey. The demand for AI technology is growing, making skilled data annotators crucial for AI model accuracy and reliability. It's essential for newcomers to choose the right platforms and invest time in learning the tools and methods of accurate data labeling.
Building a strong foundation in entry-level data annotation tasks can significantly boost your career prospects. Key skills to focus on include drawing bounding boxes, segmenting images, and transcribing text accurately. Training materials and practice exercises provide valuable experience and help develop the skills needed for quality data annotation.
Here’s a comparison of how different industries apply data annotation:
Industry | Application of Data Annotation | Benefits |
---|---|---|
Healthcare | Cancer detection, medical diagnostics | Increases diagnostic precision, speeds up patient care |
Autonomous Driving | Object and obstacle detection | Enhances vehicle safety, enables reliable autonomous navigation |
Public Safety | Surveillance, behavior analysis | Improves security measures, aids in crime prevention |
By using the excellent features and community support of platforms like DataAnnotation.tech, you can confidently start your journey in data annotation jobs for beginners. This will contribute to the growth of AI technology.
Tools and Technologies for Data Annotation
The field of data annotation has seen significant advancements, offering a broad spectrum of tools and technologies for creating high-quality datasets for AI models. These tools fall into manual, automated, and semi-automated categories, each offering distinct advantages and challenges. We will explore these options, from manual annotation software to advanced tools, to help you find the best fit for your projects.
Manual Annotation Tools
Manual annotation tools are crucial for tasks requiring high precision, especially in fields like medical and healthcare. Tools such as LabelImg, VGG Image Annotator (VIA), and CVAT are popular choices. They excel in tasks like bounding box creation, semantic segmentation, keypoint annotation, and line annotation. Although manual annotation is time-consuming and costly, it provides data of unparalleled quality, essential for training precise AI models.
Automated Annotation Tools
Automated annotation tools utilize AI models and machine learning algorithms to speed up the labeling process. While they reduce time consumption, they might not match the accuracy and detail of manual methods. Platforms like Scale AI combine automated features with project management and quality control. These tools are expected to significantly contribute to the data annotation market, projected to reach USD 5,331.0 million by 2030.
Semi-Automated Tools
Semi-automated tools strike a balance by integrating manual oversight with automated processes, enhancing both quality and efficiency. They excel in addressing complex data annotation challenges like ambiguity, labeling imbalance, and annotator bias. These tools employ AI models to generate initial predictions, which human annotators then verify and refine. This approach ensures high-quality results while cutting costs and time. Keylabs, for example, offer customizable solutions tailored to your project needs, showcasing the potential of semi-automated tools in improving your data annotation workflow.
An overview of manual, automated, and semi-automated tools is provided in the table below:
Tool Type | Examples | Advantages | Challenges |
---|---|---|---|
Manual Annotation Tools | LabelImg, VIA, CVAT | High accuracy, detailed annotation | Labor-intensive, costly |
Automated Annotation Tools | Scale AI, Labelbox | Fast, efficient | May lack accuracy, less detailed |
Semi-Automated Tools | Keylabs | Balanced, efficient, customizable | Requires initial setup and human oversight |
The Importance of Quality in Data Annotation
Ensuring data annotation quality is crucial for AI models and their object recognition abilities. The accuracy of annotated data directly impacts AI model training performance. Quality control measures boost the effectiveness and consistency of machine learning projects.
Accuracy and Reliability
Data annotation precision is key to AI system effectiveness. Tools for quality control have shown significant improvements, boosting quality from under 80% to over 95%. Audio annotation teams maintain a 99% accuracy level, while video annotation of traffic achieves quality above 99%.
Quality Control Measures
Stringent quality control measures are vital for maintaining data annotation standards. Video object-tracking uses algorithms to improve annotation accuracy, even when annotating multiple objects. Quality teams review annotation quality daily, ensuring it meets project needs. This thorough quality control reduces costs and errors, with validation often finding 3-4 times more mistakes than internal checks. In text annotation, strict quality checks help service providers meet client standards 100%, underscoring the need for precise quality control in data annotation.
Maximizing Your Earnings in Data Annotation
To boost your earnings in data annotation, focus on an efficient workflow and increasing your productivity. As a data annotator, refining your skills, optimizing your processes, and using various platforms and tools can significantly raise your income potential.
"Data annotators play a critical role in enhancing the accuracy of AI models. Your efforts directly impact the quality of AI training datasets.
Working on multiple platforms such as Fiverr, Tooa, and Clickworker can help diversify your income. Efficiently managing multiple projects and meeting deadlines is crucial for freelancers. Leveraging training and support from platforms like FutureBeeAI can also enhance your skills, leading to better job opportunities and higher earnings.
The field of data annotation is constantly evolving, introducing new tools and techniques. Staying updated with these advancements ensures your workflow remains efficient and productive, thereby boosting your earning potential. By combining your refined skills with effective workflow management, you not only improve your productivity but also open up numerous profitable opportunities in data annotation.
- Enhance Navigation Skills: Master keyboard shortcuts to navigate through annotations swiftly.
- Segment Complex Projects: Break down intricate tasks for better manageability.
- Diversify Income Sources: Work across multiple platforms to maximize your income.
- Embrace New Tools: Stay updated with the latest annotation tools and techniques.
- Focus on Quality: Combine speed with quality to ensure high-paying opportunities.
Maximizing your earnings in data annotation requires a blend of speed, quality, and efficiency. By incorporating these practices into your workflow, you can significantly boost your productivity and, subsequently, your income potential.
Summary
Through various annotation platforms and tools, you can efficiently manage complex datasets essential for sophisticated machine learning models. As the future of artificial intelligence unfolds, data annotation emerges as a critical process. The market's forecasted growth to USD 8.22 billion by 2028 highlights the need for quality annotation and the rewards of investing in this field.
By tackling the challenges and seizing the opportunities in data annotation, you significantly contribute to the future of artificial intelligence. Enhancing your skills and using advanced generative AI for tasks like image segmentation and synthetic data creation positions you for a prosperous and rewarding career in data annotation.
Starting a career in data annotation opens up significant opportunities in the AI field. It's crucial for the growth of AI technologies, from self-driving cars to improving medical diagnostics. Those skilled in data annotation play a key role in technological progress.
Entry-level positions in this field typically require only a high school diploma, making it accessible to many. Beginners usually start as Data Annotators or Labelers, focusing on accurately tagging or labeling data. There are various types of annotation, including image, text, and audio, each needing distinct skills. Specializing in areas like linguistic annotation or medical image tagging can lead to better-paying roles and advanced positions.
By sharpening your skills and gaining practical experience, you can move up from entry-level data annotation roles. Networking and continuous learning are essential for career advancement in this dynamic field. If you're committed to quality and precision, data annotation is not just a job. It's a significant step towards becoming a vital part of the innovative AI industry.
FAQ
How do I get started with data annotation jobs for beginners?
To begin with data annotation jobs for beginners, join platforms like Lionbridge, Appen, or Amazon Mechanical Turk (MTurk). These platforms offer entry-level tasks and require a registration process. This includes qualification tests or training modules.
What is data labeling for machine learning?
Data labeling for machine learning means providing accurate annotations to machine learning models. This helps them learn and predict better. It's crucial for training AI models to understand data like images, text, and audio accurately.
What skills are essential for data annotation tasks?
Key skills for data annotation include meticulous attention to detail and basic computer proficiency. Understanding AI and machine learning basics is also vital. These skills aid in tasks like image labeling, text annotation, audio transcription, and video annotation.
Which platforms are popular for data annotation jobs?
Popular platforms for data annotation jobs are Lionbridge, Appen, and Amazon Mechanical Turk (MTurk). They offer tasks such as computer vision annotation, crowdsourcing, and more.
What are the different types of data annotation tasks?
Data annotation tasks include image labeling, text annotation, audio transcription, and video annotation. Each task demands specific skills and tools. This variety offers different opportunities for data annotators.
What tools and technologies are used for data annotation?
Data annotation employs various tools, from manual tools like LabelImg and CVAT to automated and semi-automated tools like Scale AI and Keylabs.ai. These tools enhance annotation efficiency and quality.
Why is quality important in data annotation?
Quality in data annotation is vital for AI model effectiveness. Ensuring annotations are consistent and precise impacts AI system performance in real-world scenarios.
How can I maximize my earnings in data annotation?
To boost your earnings, focus on increasing your annotation speed while maintaining quality. Utilize keyboard shortcuts and divide complex tasks into smaller parts to enhance efficiency and earnings.
How can data annotation lead to a career in AI?
Data annotation opens doors to the AI industry by providing essential skills and experience. By refining your annotation skills, you can contribute to advanced AI projects. This can lead to broader AI career opportunities, making data annotation a stepping stone to innovation and transformation.
Comments ()