Guard Your AI: Essentials for Picking a Trustworthy Data Labeling Partner
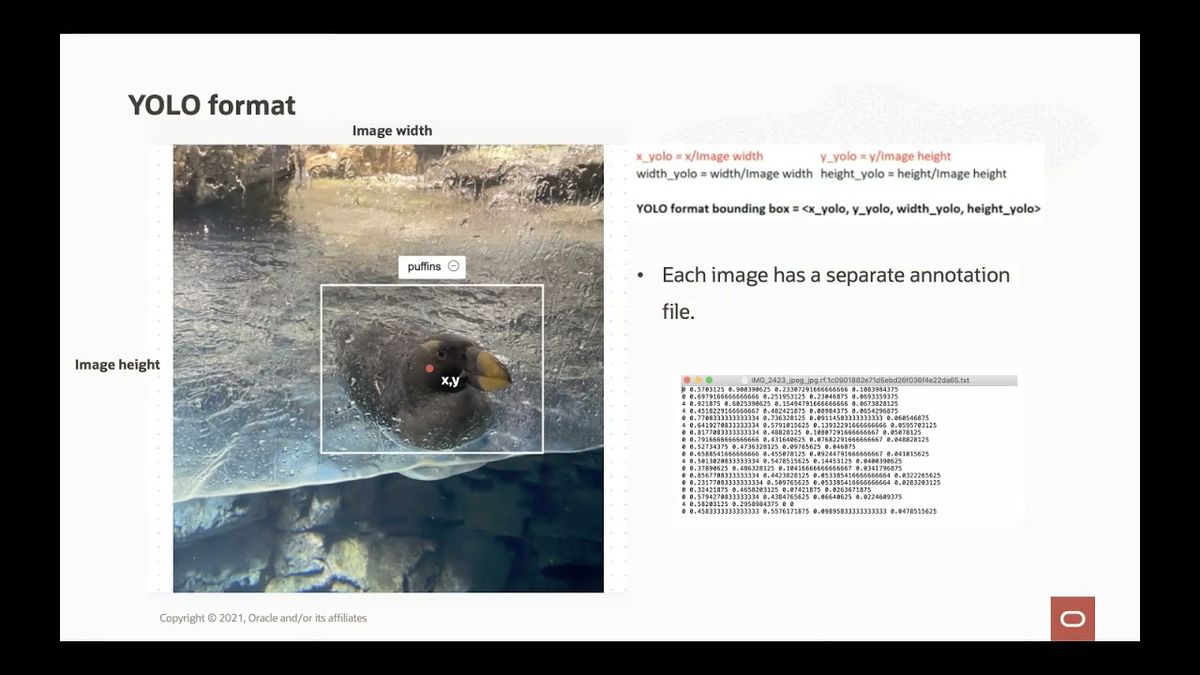
In today's digital age, data is the backbone of every business. It drives decision-making, improves customer experiences, and helps companies stay ahead of the competition. However, to leverage the power of data, it needs to be accurately labeled and annotated. Data labeling is a crucial process that involves assigning relevant tags and metadata to raw data to make it understandable and usable.
But, data labeling is not a simple task. It requires specialized skills, tools, and expertise to ensure accuracy, consistency, and quality. That's why many companies outsource their data labeling needs to third-party providers. However, choosing a trustworthy data labeling partner is not an easy feat. With so many options available, it can be overwhelming to make the right choice.
In this article, we'll discuss the essentials of picking a reliable data labeling partner. We'll cover factors such as quality control measures, security protocols, pricing models, and more. We'll also explore case studies and customer reviews to provide a comprehensive overview of what to expect from a data labeling partner. Lastly, we'll look at future trends and innovations in data labeling to help businesses stay ahead of the curve.
The Importance Of Data Labeling
Choosing a reliable data labeling partner is essential in ensuring accurate and efficient machine learning outcomes. The labeling process involves tagging collected data to aid in the training of machine learning algorithms. It is imperative to choose a partner with experience and expertise in your industry to achieve better results.
Quality assurance measures are crucial when selecting a data labeling partner. These measures help maintain accuracy by identifying and correcting errors, ultimately saving time and resources. It's also essential to ensure that the labeling partner adheres to high levels of data security, confidentiality, and compliance protocols.
There are different ways one can approach data labeling that vary depending on your needs. In-house labeling works best when dealing with sensitive or proprietary information, while crowdsourcing allows for quick scaling. Outsourcing may be an ideal solution when requiring project-specific expertise.
When selecting a data labeling partner, consider their track record and reviews from other clients as this will give an insight into their capabilities. Partnering with someone who has the right credentials ensures that you receive quality work within stipulated timelines while maintaining efficiency during data processing.
Having a trustworthy labeler saves time on training models again due to imprecise labels or inconsistent standards between multiple labelers' work therefore picking them correctly is key in obtaining effective machine learning outcomes from accurately labeled datasets .
Factors To Consider When Choosing A Data Labeling Partner
When choosing a data labeling partner, several factors should be taken into consideration to ensure the success of your project. One important factor is the level of expertise that the partner possesses, especially when it comes to handling complex data labeling projects. Look for a partner that has a team of experts with necessary skills and experience.
Another crucial factor is the ability of the partner to scale according to your needs and successfully manage program management practices. Consider whether they have established recruiting and onboarding processes in place, as well as being able to manage large volumes of data within predetermined timelines.
Data security capabilities cannot be overlooked either. The partner should clarify confidentiality measures in their processes, personal data processing in accordance with instructions, compliance with applicable laws and regulations regarding data privacy protection, and policies on deletion or return of data after the end of processing period.
It's also important to consider cost when choosing a partner. While cost shouldn't be your only deciding factor, make sure you choose a provider that offers good value for money not just upfront, but throughout each project phase.
Trustworthiness hinges on trust! Trust and communication are vital factors when entrusting confidential business data to an external party - choose wisely who you will work with based on their proven track record in delivering quality output consistently-satisfying customer expectations.
Choosing an unreliable or insufficiently experienced service provider can lead to project delays, broken algorithms due dirty/dubious input semantic annotations being used for training datasets validation stages; surprise costs related tasks like future consulting fees from third-party experts (e.g., legal counsel) or worse yet breaches in sensitive information leading legal action typically instigated by stakeholders/customers themselves because something went wrong somewhere along the way without proper documentation steps taken by both parties involved beforehand regarding any expected outcomes or solutions sought out together cohesively between all parties working together concertedly synergistically aligned towards shared common mission-vision targets aspirations jointly -so always stay cautious/vigilant!
Quality Control Measures And Training Programs
Quality control measures and training programs are essential for data labeling service providers to ensure they deliver high-quality labeled data. Supervised learning algorithms require labeled instances, making the quality assurance and consistency in data labeling crucial. AI and ML models rely on high-quality training datasets, which can only be achieved through strict quality control measures.
Implementing a robust quality assurance process starts with defining the right quality metrics to evaluate the accuracy of the labeled data. Quality control measures should be enforced at every stage of the procurement process – from acquiring raw data to delivering labeled datasets. This is necessary to maintain consistency and avoid errors that could affect machine learning models' performance.
Training programs are also important for maintaining high standards in data labeling. These programs equip labelers with essential skills to perform their tasks accurately, consistently, and efficiently. Adequate training ensures that labelers understand how to detect anomalies, categorize entities correctly, avoid bias or ambiguity when interpreting instructions or labeling criteria.
To meet legal obligations such as GDPR (General Data Protection Regulation), all stakeholders must ensure social accountability by complying with privacy regulations when handling sensitive or personal information belonging to individuals or organizations.
In conclusion, choosing a reliable partner requires selecting a company that prioritizes stringent quality control measures accompanied by proper training programs for their workforce responsible for providing labeled datasets.
Security And Confidentiality Protocols
When choosing a data labeling partner, security and confidentiality protocols are extremely important. To ensure the safety of your sensitive data, companies should have strict physical access controls and clean desk protocols in place. It's crucial to inquire about a company's security protocols and handling of sensitive data before partnering with them.
Properly trained staff members should be responsible for labeling data, undergoing background checks and signing confidentiality agreements. Data labeling partners must have expertise and technology to meet all data requirements while maintaining confidentiality. The GDPR requires organizations to provide timely information regarding data breaches and perform DPIAs.
Privacy, security, and confidentiality may have different meanings but they are all equally important in protecting personal data. Information Transfer Security labels are used to control access and handling restrictions of data.The result of choosing an unreliable or incompetent partner can lead to significant harm such as loss of customers' trust or financial losses due to legal implications.
Data privacy is essential in today's increasingly digital world, so make sure that you select a trusted partner who is transparent about their security measures for keeping your confidential information safe from prying eyes.
Flexibility And Scalability Of Services
Flexibility and scalability are critical when choosing a trustworthy data labeling partner. Outsourcing data labeling operations can significantly reduce costs and leverage the latest technology without building an in-house infrastructure. A reliable offshore group for data labeling services should have the ability to support multiple sensors and various types of data while ensuring scalability.
When outsourcing data labeling, it’s essential to consider whether or not a provider can scale production quickly while maintaining high quality. The scalability of services depends heavily on flexibility, especially regarding meeting deadlines without compromising work quality and accuracy. With flexible scheduling arrangements, providers can meet project needs promptly without impacting essential business operations.
As data storage needs continue to grow, providers must ensure that their teams consist of labelers who understand the nuances of projects and make subjective decisions accurately. Providers should also implement robust hiring and vetting processes that evaluate potential labelers’ proficiency before they join their teams.
Transparency is crucial for scaling teams successfully since it ensures that all stakeholders are up-to-date with project timelines and understanding shift priorities when changes arise. Additionally, transparent workflows make it easier to change machine learning lifecycles as projects evolve over time.
In summary, selecting a trustworthy partner for data labeling requires comprehensive consideration around flexibility and scalability of services provided throughout the project lifecycle. With flexible scheduling arrangements fueled by transparency in workflows, offshore groups gained through outsourcing present immense advantages financially and quality-wise for businesses aiming to improve their ML efforts while also freeing up company resources for other key areas such as development efforts or improving existing strategies ultimately propelling growth objectives forward effectively within budgets constraints often seen within startup environments across industries today.
Case Studies And Customer Reviews
Choosing a trustworthy data labeling partner is essential for the success of any Machine Learning (ML) project. Accurate data labeling, or annotation, is crucial in preparing datasets for AI models to learn and work effectively. However, not all data labeling partners are created equal. It's crucial to ensure that the partner has enough experience within your specific industry and can be expected to maintain quality and consistency throughout the process.
In addition to checking an annotation company's reputation through customer reviews, it's also important to consider their partnerships with reputable companies within your industry. For example, Recycleye worked with Keymakr Data Annotation service company to label and edit data for a client’s AI model in waste management projects.
An important consideration when working with external parties is data privacy. Tough questions must be asked regarding how personal information will be handled during the labeling process to keep sensitive data private and safe from exposure. By evaluating potential partners carefully based on these vetting points while ensuring quality services are provided across boarders can help make informed decisions on selecting a reliable partner who can provide effective pre-labeled datasets needed in ML tasks of diverse applications.
Future Trends And Innovations In Data Labeling
As more businesses rely on machine learning models, data annotation services have become crucial for obtaining the labeled data needed to train these algorithms. The global data annotation services market is expected to reach $5.3 billion by 2030 due to an increasing demand for labeled data that surpasses traditional manual labeling supply. Three key processes in machine learning models include initial model training, inference, and output.
Data labeling companies continuously grow at a rate of 28.4% and are projected to generate $3.5 billion in revenue by 2026. One trend among these companies is automation, which leads to greater efficiency and accuracy in the labeling process. Healthcare also relies heavily on data labeling as it applies machine learning in areas such as drug development and medical imaging analysis.
Predictive annotation is another up-and-coming trend where algorithms make predictions based on incomplete information before completing annotations based on those predictions. However, quality control procedures must be implemented to ensure the highest level of accuracy possible.
Lastly, encoding variable data has become a prime trend with an emphasis on quicker and more accurate product serialization and extended attributes labeling for narrow AI applications.
For businesses looking towards long-term strategies with digital trust at their core, two driving forces are predicting future trends and leveraging cybersecurity measures that enhance overall trust in the way we utilize big data today.
Overall, eight of the top trends within D&A leaders regarding using top D&A trends for 2022 include activating dynamism & diversity imperatives along with augmented people/decisions while institutionalizing trust when picking trustworthy Data Labeling Partners within Business Intelligence requirements have emerged from these findings thanks to companies pushing toward standardization through improved protocols designed specifically around specific verticals like healthcare applications or enterprise commerce solutions alike."
Comments ()