High-Accuracy Video Annotation for Security AI Applications
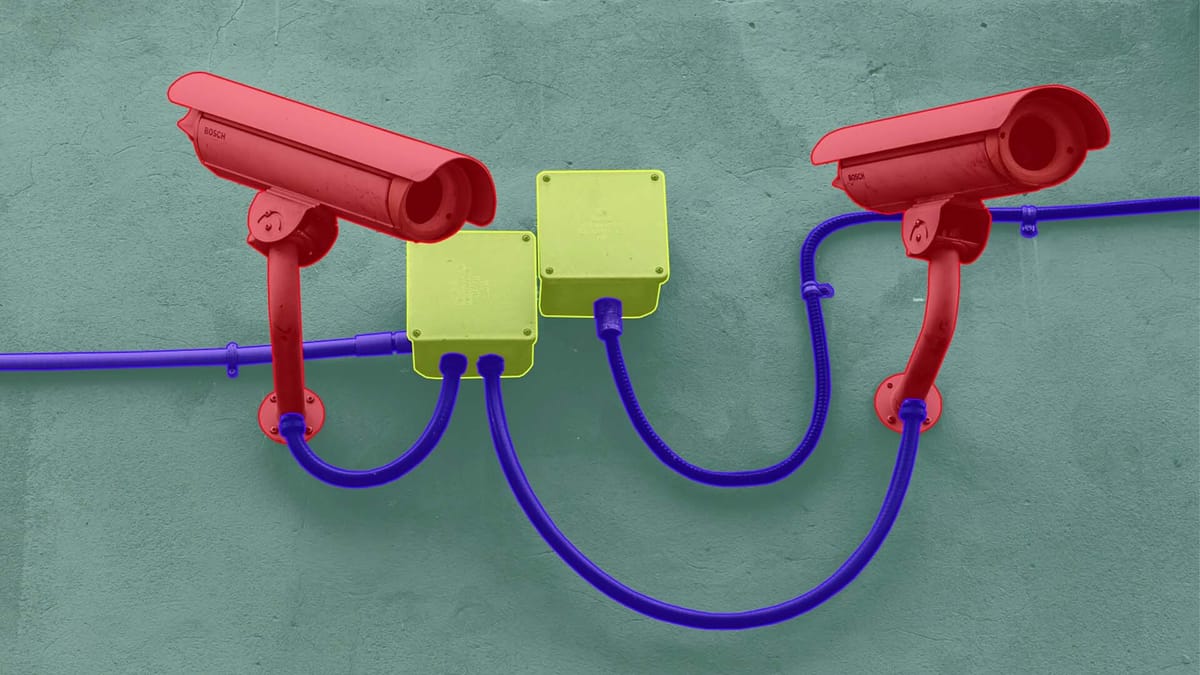
The fusion of high-accuracy annotation with video surveillance systems turns raw footage into detailed, actionable insights. This precision video tagging process is vital for boosting AI model capabilities. It enables them to spot patterns, objects, and human behaviors with high precision.
Dedication to quality ensures these annotations meet the highest standards. This is critical for developing strong security measures and advanced surveillance methods. By using high-accuracy annotation, we enhance our AI's performance. This, in turn, boosts the reliability of our security systems, making them more adept at detecting threats.
Delving into the intricacies of security video annotation reveals its vast benefits and applications. It plays a central role in refining AI model training and surveillance systems. High-accuracy annotation is a cornerstone in our quest for superior artificial intelligence security solutions.
Key Takeaways
- High-accuracy video annotation transforms raw surveillance data into actionable insights.
- Precision video tagging significantly enhances AI model performance.
- Efficient annotation methods contribute to more effective security systems.
- Applications span security surveillance, traffic management, and behavior monitoring.
- Choosing the right tools and quality control measures is critical for accurate annotation.
Understanding Security Video Annotation
Annotating videos is key for teaching machines to "see" by providing examples that help them understand the visual world. Using video annotation tools, these annotations boost AI accuracy. They are vital for recognizing objects and patterns, essential in self-driving cars, security cameras, and sports analysis.
What is Video Annotation?
Video annotation labels video data, making it understandable to AI systems. It involves tagging raw footage with labels that identify and categorize objects, actions, or events. This labeled data is critical for training computer vision models. High-quality annotations make video content searches efficient, allowing easy access to specific scenes. They are useful in studying animal behavior and analyzing disease progression.
Importance in Security Systems
Accurate video annotations greatly enhance the reliability of security systems. They enable AI-powered security solutions to detect objects, recognize behavior, and analyze threats precisely. This capability is vital for ensuring safety in public infrastructure and retail. Proper data labeling in video annotation tools ensures these systems can accurately interpret real-time security footage. This expands surveillance capabilities.
Types of Video Annotation Methods
Understanding the various video annotation methods is key to achieving high video labeling accuracy. By combining manual, automated, and semi-automated annotations, businesses and tech professionals can improve their AI models. These improvements are vital for applications like security, healthcare, and autonomous driving.
Manual Annotation
Manual annotation involves human annotators labeling video data frame by frame. This method ensures high precision, making it ideal for complex tasks that require detailed attention. While it offers unparalleled accuracy, it is also time-consuming.
Automated Annotation
Automated annotation uses algorithms and machine learning to label video data quickly. Though automated methods are faster and more scalable, they may not match the accuracy of manual annotation. They are best for straightforward scenarios with clear, predictable patterns.
Semi-Automated Annotation
Semi-automated annotation blends the strengths of manual and automated approaches. It uses automated tools for straightforward tasks and human annotators for complex ones. This hybrid method offers a balance of speed and accuracy, making it ideal for scalable and precise security applications.
Annotation Method | Precision | Speed | Best Suited For |
---|---|---|---|
Manual Annotation | High | Low | Complex Tasks |
Automated Annotation | Moderate | High | Straightforward Scenarios |
Semi-Automated Annotation | High | Moderate | Scalable, Precise Tasks |
Choosing the right video annotation method is essential for optimal results, whether in security or healthcare. Consider outsourcing these tasks to reliable service providers. This can save time and resources while ensuring high-quality results.
Key Features of Effective Annotation Tools
Modern security applications require robust annotation platforms. These tools must meet the detailed needs of video annotation. The following features ensure these tools are efficient and reliable for security surveillance tasks.
User-Friendly Interface
Effective annotation tools need a user-friendly interface for ease of use. A well-designed interface makes navigation and task performance effortless. This reduces training time and boosts productivity. Such software supports various annotation types, including bounding boxes and semantic segmentation, for accurate data annotation.
High Customization Options
Customizing annotation tools is key for adapting to specific project needs. High customization options allow for unique features like facial recognition and 3D information. This ensures accurate identification of behaviors and objects, even the most nuanced ones.
Real-Time Collaboration
Real-time collaboration is vital for large-scale projects. Collaborative annotation tools enable team members to annotate simultaneously. This ensures consistency and speeds up the process. Such platforms also use AI-assisted labeling and automated object tracking to boost productivity and accuracy.
Feature | Importance | Examples |
---|---|---|
Advanced Video Handling | Enables effective processing of long and complex videos. | Facial Recognition, Gait Analysis |
Dynamic Classifications | Allows categorizing events and frames for detailed data. | Crime Detection, Behavior Analysis |
AI-Assisted Labeling | Improves annotation efficiency with automated processes. | Object Tracking, Interpolation |
Benefits of High-Accuracy Video Annotation
High-accuracy video annotation is vital for modern AI applications, most noticeably in security. It enables systems to train more precisely and efficiently. This significantly enhances various industry-specific applications.
Improved AI Model Training
High-accuracy video annotations offer detailed, structured data critical for training advanced AI models. This data enables AI systems to learn more effectively. They improve in tasks like object detection, tracking, and recognition. As companies increasingly rely on AI/ML models, high-quality annotated data for enhanced AI training is essential.
Enhanced Surveillance Systems
Accurate video data annotation leads to accurate surveillance systems. These systems can better identify objects, individuals, and behaviors. This is critical for analyzing threats and suspicious activities. Enhanced surveillance is vital for ensuring safety in public and private sectors, forming the backbone of modern security infrastructures.
Faster Threat Detection
High-accuracy video annotation enhances rapid threat detection. It provides AI systems with precise data, reducing the time to recognize and respond to threats. This rapid detection is key to safeguarding environments, from corporate premises to metropolitan areas.
These advantages lead to the development of efficient security solutions capable of handling real-world complexities. Video annotation ensures AI models remain robust and responsive. It opens the door to future innovations in security and beyond.
Aspect | Benefits | Applications |
---|---|---|
Improved AI Model Training | Higher accuracy and performance of AI models | AI/ML models, autonomous driving, medical imaging |
Enhanced Surveillance Systems | More effective threat analysis and detection | Public security, private surveillance, crime prevention |
Faster Threat Detection | Rapid recognition and response to threats | Corporate security, metropolitan safety, emergency response |
Challenges in Security Video Annotation
The field of security video annotation faces many hurdles that can affect the precision and utility of AI models. It's essential to grasp these challenges to adopt effective and secure annotation methods.
Data Privacy Concerns
Protecting data privacy during video annotation is a top priority. Annotated data must adhere to legal and ethical norms to safeguard individual privacy and foster trust. This is more critical with sensitive content, like surveillance footage. Secure annotation practices are key to preventing unauthorized access and data breaches, ensuring privacy.
Varying Quality of Training Data
The quality of training data in video annotation can differ greatly, leading to inaccuracies in AI model training. High-quality data is critical for developing AI systems that can accurately detect objects and recognize faces. To overcome these challenges, advanced tools and methods are needed to standardize and improve data quality, ensuring consistency across datasets.
- Organize data into manageable chunks.
- Combine auto-annotation with human verification.
- Implement continuous quality assurance checks.
By adhering to these strategies, the accuracy of annotated videos can be enhanced. This improvement enables better performance in real-world scenarios. Businesses can cut costs and expedite video annotation tasks by integrating AI with human annotators, boosting overall efficiency.
Challenges | Solutions |
---|---|
Data Privacy | Adopt secure annotation practices and comply with legal standards. |
Quality of Training Data | Use sophisticated tools to standardize and enhance data quality. |
Real-World Applications of Video Annotation in Security
Video annotation technology is evolving rapidly, becoming essential in various security applications. It plays a critical role in crime scene investigation, traffic monitoring, and intrusion detection. These applications highlight the importance of video annotation in modern security systems.
Crime Scene Investigation
In AI in crime investigation, video annotation is vital. It helps document and analyze crime scenes accurately. This is essential for effective investigations and evidence gathering. Annotated videos allow law enforcement to track and review events efficiently.
Advanced tools like automated video labeling speed up the annotation process. They can annotate videos up to 90% faster, reducing costs significantly.
Traffic Monitoring
Traffic surveillance AI enhances traffic monitoring systems. Annotated videos help AI systems detect irregular patterns, such as traffic congestion and accidents. This enables swift responses and corrective measures.
Automated video annotation tools improve this process. They provide high accuracy and efficiency in identifying objects in traffic. This ensures monitoring systems operate at their best.
Intrusion Detection
Robust intrusion detection systems are essential for securing sensitive areas. Video annotation plays a key role in these systems. It enables precise tracking and identification of suspicious activities.
Annotated videos in real-time surveillance trigger alerts and initiate security responses promptly. Advanced tools like multi-object tracking and auto object segmentation enhance system reliability. They ensure any intrusion is quickly detected and addressed.
Application | Benefits | Key Tools |
---|---|---|
Crime Scene Investigation | Detailed Analysis, Efficient Evidence Gathering | Keylabs, Automated Video Labeling |
Traffic Monitoring | Improved Traffic Management, Violation Detection | AI-Powered Annotation Tools |
Intrusion Detection | Real-Time Alerts, Accurate Tracking | Multi-Object Tracking, Auto Object Segmentation |
Best Practices for Video Annotation in Security
In the rapidly evolving security AI landscape, establishing reliable video tagging methods is critical. Adhering to best practices in video annotation ensures precision and consistency. This enhances the overall effectiveness of security systems. We explore key practices in defining annotation guidelines and maintaining continuous quality assurance, elements essential for successful video annotation projects.
Defining Clear Annotation Guidelines
One of the fundamental steps in video annotation involves defining clear annotation guidelines. This creates a uniform standard that annotators can follow. Here are essential practices:
- Task Specification: Break down videos into shorter chunks, considering frame rates for efficient annotation.
- Category Definitions: Clearly define objects and associated actions within security videos, ensuring uniformity across datasets.
- Annotation Examples: Provide annotators with examples that illustrate expected results for different annotation types.
By maintaining detailed and comprehensible annotation guidelines, we uphold a high level of accuracy. This is critical for training reliable AI models in security applications, minimizing room for error.
Continuous Quality Assurance
Continuous quality assurance is indispensible in video annotation. Regular checks and systematic updates contribute to maintaining high accuracy standards. Key strategies include:
- Regular Review Cycles: Schedule periodic evaluations to review annotated videos, ensuring that they meet the required quality.
- Active Learning: Integrate feedback loops where AI models learn and improve based on new data during deployment stages.
- Performance Metrics: Implement detailed performance insights, including Time per Task (TPT) and accuracy rates for each annotator.
Emphasizing quality assurance in annotation ensures the reliability of video tagging, a necessity for high-risk surveillance AI applications.
For more insights on quality assurance and defining annotation guidelines, you can refer to our detailed guide.
The global market for AI surveillance systems is anticipated to burgeon, highlighting the need for robust video annotation practices. Employing these best practices enhances our capacity to implement effective and dependable security AI solutions.
Emerging Technologies in Video Annotation
The field of video annotation is rapidly evolving, driven by new technologies. These advancements are transforming how we handle and use video data, with a significant impact on security applications.
Machine Learning Enhancements
Recent breakthroughs in machine learning are significantly boosting the speed and precision of video annotation. AI tools are reducing the need for manual tagging, leading to faster and more scalable processes. Now, object detection and behavior analysis are more advanced, allowing security AI systems to quickly spot and respond to threats. This improvement enhances the overall effectiveness of these systems.
Integration with IoT Devices
The merging of IoT devices with video annotation systems is creating a powerful network for real-time data collection. IoT integration enables the smooth combination of video feeds from various cameras, sensors, and devices. This integration broadens the reach of surveillance systems and enriches data annotation, boosting situational awareness and response capabilities.
Cloud-Based Solutions
Cloud-based annotation solutions bring significant advantages in terms of scalability, accessibility, and cost-effectiveness. By using cloud platforms, organizations can efficiently manage and process large volumes of annotated video data. This model supports real-time and 3D video annotation, essential for augmented reality and autonomous vehicles. It also allows for continuous updates and collaboration, keeping video annotation projects relevant and impactful.
"The integration of innovative security technologies and cloud-based solutions is transforming the future of video annotation, making it more efficient and resilient in managing unstructured data."
- Machine learning advancements are reducing manual tagging needs.
- Integration with IoT increases the effectiveness of surveillance.
- Cloud-based annotation solutions offer scalable and real-time processing options.
Future Trends in Security Video Annotation
The future of security video annotation is set to see major breakthroughs. Automated security systems are becoming more prevalent, thanks to AI-driven analysis. These systems aim to cut manual annotation time by up to 50%. AI handles both the annotation and analysis, boosting efficiency and accuracy in security tasks.
Shift Towards Fully Automated Systems
We expect a rise in fully automated systems that need little human input. These systems use machine learning to improve data annotation speed and quality.
Companies are increasingly turning to outsourced data annotation services. These services offer specialized annotations, vital for training high-quality models. This move is key for businesses looking to cut costs and reduce the burden of maintaining an in-house annotation team.
Increased Focus on Real-Time Analysis
There's also a growing emphasis on real-time video analytics. This technology offers immediate insights and quick responses, essential for today's security needs. By 2024, 80% of new data pipelines will handle unstructured data ingestion, processing, and storage.
FAQ
What is Video Annotation?
Video annotation is the process of labeling video data to make it discernible to AI systems. This involves tagging raw footage with labels to identify and categorize objects, actions, or events. It's essential for training computer vision models.
What is the importance of video annotation in security systems?
Accurate video annotations increase reliability in security systems. They enable precise object detection, behavior recognition, and threat analysis. This is vital for maintaining safety and security in various environments.
What is manual annotation?
Manual annotation involves annotators labeling video data frame by frame. It allows for high precision but is time-intensive.
What is automated annotation?
Automated annotation uses algorithms to automatically label video data. It's faster but may lack the accuracy of manual methods. It's suitable for clear-cut scenarios.
What is semi-automated annotation?
Semi-automated annotation combines automated and manual annotations. It optimizes both speed and accuracy, making it essential for scalable, precise security applications.
What are the key features of effective annotation tools?
Effective annotation tools should have a user-friendly interface and high customization options. They should also support real-time collaboration. This facilitates efficient and precise video annotation processes.
How do high-accuracy video annotations improve AI model training?
High-accuracy annotations provide detailed data for training sophisticated AI models. These models perform reliably, improving the effectiveness of AI-powered security solutions.
How do video annotations enhance surveillance systems?
Accurate annotations lead to improved surveillance capabilities. Systems can detect and analyze threats or unusual behavior more effectively.
What are the challenges in video annotation regarding data privacy?
Ensuring annotated data complies with legal and ethical standards is essential. Adequate measures must be in place to protect sensitive information.
Why does the quality of training data vary in video annotation?
The quality of input data can vary due to differences in video sources, recording conditions, and labeling practices. Sophisticated tools are needed to standardize this data for accurate model training.
How is video annotation used in crime scene investigation?
Video annotation helps in detailed documentation and analysis of crime scenes. It aids in more effective investigations and evidence gathering.
How can AI-driven traffic monitoring benefit from video annotation?
AI-driven traffic monitoring systems using annotated videos can manage traffic flow. They detect violations more efficiently and improve overall road safety.
What role does video annotation play in intrusion detection?
Advanced intrusion detection systems use video annotations to identify unauthorized entries automatically. They trigger alerts, improving security measures.
What are the best practices for video annotation in security?
Best practices include defining clear annotation guidelines and maintaining continuous quality assurance. This ensures consistency and precision in the annotated data.
What are the emerging technologies in video annotation?
Emerging technologies include enhancements in machine learning and integration with IoT devices. Cloud-based solutions offer innovative and scalable options for security applications.
What future trends can we expect in security video annotation?
Future trends include a shift towards fully automated systems and a focus on real-time analysis. This could significantly enhance the efficiency and responsiveness of security monitoring.
Comments ()