High-Precision Image Annotation for Accurate AI Model Training
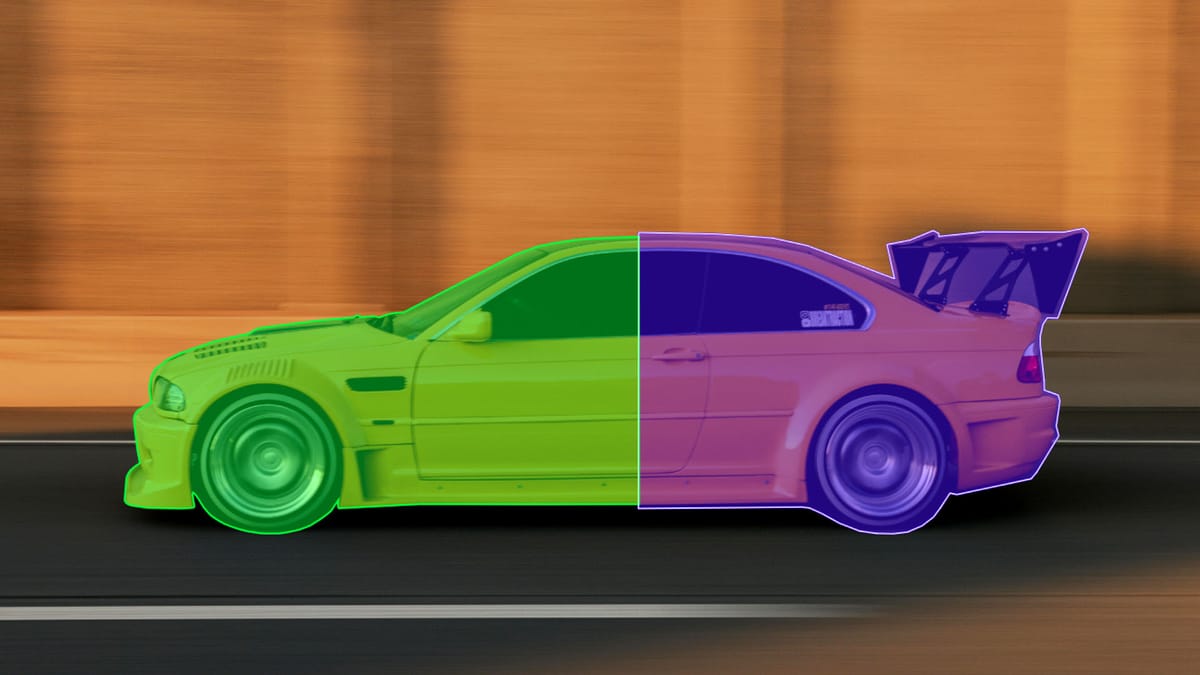
High-precision image annotation is key to advancing artificial intelligence. It boosts AI model accuracy through detailed data labeling. This process is vital for computer vision, enabling algorithms to grasp and decode digital images. By merging advanced technology with human expertise, businesses strive to offer datasets of unmatched precision and dependability.
Key Takeaways
- Appen collaborates with global leaders to drive high-quality data labeling for accurate AI model training.
- Labelbox's automation features enhance pre-built models and workflows for effective image annotation.
- Keylabs provides integrated and automated image annotation solutions.
What are Image Annotation Services?
Image annotation services involve the meticulous task of labeling features within images. This fundamental process enables machine learning models to learn and recognize patterns independently. It provides the essential groundwork needed for robust AI systems. Accurate annotations delineate the parameters for tasks such as object detection and classification. This significantly contributes to the development of advanced AI applications across various industries.
Definition of Image Annotation
At its core, image annotation translates into tagging and labeling images with metadata to train machine learning algorithms. Given the diverse range of tasks, from image tagging services to semantic segmentation, these annotations can take multiple forms. For example, bounding box annotation offers a straightforward way to outline objects within an image, which is computationally efficient. On the other hand, more complex tasks may necessitate semantic segmentation, where each pixel is labeled to provide a more detailed image understanding.
Importance in AI and Machine Learning
High-quality image annotation is critical for the accuracy of AI models. It serves as the foundation for informed learning and complex pattern recognition in machine learning ecosystems. To effectively train these algorithms, hundreds to thousands of annotated images are required. This significantly enhances the predictions made by AI systems.
Industries rely on advanced techniques like object detection using algorithms such as CNN, R-CNN, and YOLO to execute tasks effectively. Leveraging semantic segmentation within AI applications allows for precise categorization of various image fragments. This is indispensable in fields like autonomous vehicles, agriculture, and surveillance.
Types of Image Annotation Services
It's essential to grasp the various image annotation services for your AI and machine learning endeavors. Each approach brings its own set of benefits, tailored to specific data labeling and image recognition needs.
Bounding Box Annotation
Bounding box annotation is a prevalent method in computer vision. It entails drawing rectangular boxes around objects to outline their location and dimensions. This technique is widely applied in sectors like autonomous vehicles, retail, healthcare, and surveillance. For example, bounding boxes in autonomous vehicles are key to identifying and tracking objects like pedestrians, ensuring safe movement.
Polygon Annotation
Polygon annotation is used for objects with irregular shapes, requiring more detailed boundary definitions. Utilizing polygonal annotations, all edges of an object can be accurately outlined. This method is perfect for complex objects. It's vital in fields like satellite and drone imagery analysis, where precise object borders are necessary. The iMerit team's expertise in these annotations ensures detailed data labeling.
Semantic Segmentation
Semantic segmentation labels each pixel of an image for a detailed, contextual understanding. This technique is invaluable in scenarios requiring a thorough scene analysis, such as urban planning or autonomous vehicle navigation. The iMerit team excels in segmenting images into component parts, ensuring no detail is overlooked in the image recognition process.
Applications of Image Annotation
Expert image annotation is vital in various sectors, boosting AI model performance. We'll dive into its importance in healthcare imaging, autonomous driving, and retail and e-commerce.
Healthcare and Imaging
In healthcare, image annotation is key for AI model training. It enhances diagnostic accuracy. Through healthcare image annotation, doctors can use AI to spot diseases like cancer more accurately. Detailed annotations on medical images allow AI to learn from large datasets, boosting its ability to detect anomalies precisely.
This annotated data is vital for tools like YOLO, enabling real-time object detection and segmentation in medical images.
Autonomous Vehicles
Autonomous driving technology heavily relies on precise image annotation for safety and navigation. It creates essential datasets for training self-driving cars. Annotated data is needed for tasks like in-cabin behavior monitoring and steering response.
Tools like Keylabs and Labelbox, with features like automated queuing and pre-labeling, can increase efficiency by up to 50%. Annotation is critical in autonomous driving, used in LiDAR sensing and movement tracking. These are key for advancing driver-assistance systems.
Retail and E-commerce
In retail, retail AI and e-commerce machine learning bring significant improvements. Accurate image annotation is vital for virtual inventory management and predicting shopping behavior. Semantic segmentation and item tagging help retailers optimize merchandising and offer personalized shopping experiences.
Benefits of Using Professional Annotation Services
Engaging professional annotation services offers significant advantages for businesses in AI and machine learning. These benefits include enhanced data quality, quicker turnaround times, and the capacity to scale effectively. This meets the evolving demands of projects.
Improved Data Quality
Professional annotation services stand out for their meticulous verification processes. These ensure efficient data labeling, critical for creating precise and dependable AI/ML models. With strict quality control, businesses can achieve over 98% accuracy in their data, as Infosys BPM expertly demonstrates.
Faster Turnaround Times
These services employ streamlined workflows and advanced AI tools to annotate datasets swiftly. This efficiency leads to quicker turnaround times, enabling businesses to accelerate their AI model training and deployment. The rapid processing of large data volumes gives companies a competitive edge, helping them stay ahead in fast-paced markets.
Scalability
Scalability is a key benefit of professional annotation services. They offer scalable image annotation services, accommodating the growing needs of larger datasets and more complex models. The ability to adjust annotator resources as needed ensures ongoing efficiency and effectiveness. This makes it simpler for businesses to manage and scale their AI projects.
- Enhanced data accuracy through high-quality annotation.
- Efficient data labeling leading to reliable AI models.
- Quick processing and data annotation with advanced tools.
- Scalable services to manage large datasets effectively.
How to Choose the Right Image Annotation Service
Choosing the right image annotation service is critical for the success of AI and machine learning projects. It requires evaluating the provider's expertise, technology, and tools. Also, their track record through customer reviews and case studies is essential. Here, we outline the key factors to consider for an informed decision.
Assessing Expertise and Experience
Assessing the provider's annotation expertise is a key aspect. They should understand various annotation types, such as bounding box and semantic segmentation. Keymakr is known for its expertise in complex projects. It's also important to check for clear guidelines, quality control processes, and high inter-annotator agreement for consistency and accuracy.
Evaluation of Technology and Tools
A thorough technology assessment in annotation is essential. The tools and software should be advanced, with user-friendly interfaces and integration with machine learning frameworks. Secure cloud storage with robust encryption is also critical for data security. Ensure the provider uses advanced tools for various annotation techniques, like 3D cuboids, for high precision in projects like robotics or sports analysis.
Image Annotation Process Explained
The image annotation process is vital for creating precise AI models. It involves detailed steps to ensure top-notch accuracy. From data preparation to quality assurance, each phase is critical for success.
Data Preparation
Starting with data preparation for AI means selecting and organizing images carefully. This stage ensures the data is relevant and representative. High-quality images, free from noise, are essential for achieving 99% accuracy in annotations.
Annotation Techniques
Various annotation techniques are used depending on the project's needs. Techniques like bounding box, polygon annotation, and semantic segmentation are employed. For example, bounding boxes are great for object detection, while semantic segmentation excels in pixel-level analysis.
Quality Assurance
Quality assurance in data labeling is key to ensuring reliable datasets. Thorough quality checks are conducted to maintain accuracy and consistency. Multiple reviewers validate the annotations, correcting any errors. This rigorous QA process ensures high-quality data for AI model training in healthcare, autonomous vehicles, and agriculture.
Industry | Application |
---|---|
Healthcare | Training AI algorithms for medical imaging to detect anomalies |
Autonomous Vehicles | Optimizing image recognition for navigation and object detection |
Retail & E-commerce | Classifying objects and assisting customers in finding items easily |
Agriculture | Identifying crop conditions for better yield management |
Cost Factors in Image Annotation Services
The cost of image annotation is shaped by several key factors essential for training AI models accurately. Achieving a balance between affordability and data quality hinges on grasping these determinants. We explore the three main cost factors: project complexity, image volume, and turnaround time needs.
Complexity of the Project
Project complexity is a significant cost influencer in image annotation services. For example, using advanced techniques like instance segmentation or cubic bounding boxes requires specialized skills and more time, increasing costs. Annotating complex 3D LiDAR point clouds is more time-consuming than annotating simpler 2D images. The complexity also depends on the number of labels and classes, as projects with more complexity need more time and resources for quality assurance.
- Advanced techniques require expert annotators.
- Higher label/class counts increase project complexity.
- Detailed project planning can help manage complex annotation tasks.
Turnaround Time Requirements
Expedited services for quicker turnaround times come at a higher cost. For urgent projects, validating a partner's ability to meet deadlines is critical. Service providers may charge extra for tight schedules without compromising on annotation quality. Regular quality checks, like the Honeypot method, ensure high standards but increase time and cost.
- Expedited services incur additional fees.
- Quality checks ensure reliability.
- Long-term engagements may offer better rates.
In conclusion, understanding project complexity, image volume, and turnaround times is key to budgeting for cost-effective image annotation. Finding the right balance requires a nuanced approach, ensuring quality annotations without overspending.
Common Challenges in Image Annotation
Image annotation is vital for training AI models. Yet, it faces several challenges. Overcoming these challenges in image annotation is key to creating reliable datasets for AI use.
Variability in Image Quality
One major challenge in image annotation is image quality variability. Inconsistent image quality can greatly affect annotation accuracy. Such errors can harm model performance. To mitigate this, clear guidelines, skilled annotators, quality checks, and automation are essential. Expert human annotation services are vital for improving ML and AI.
Subjectivity in Labeling
Different annotators may have varying interpretations, causing inconsistencies. This subjectivity in labeling is a significant challenge in image annotation. To address this, clear guidelines, multiple annotators, and open communication are necessary. Inconsistencies in annotation can compromise data integrity and AI model accuracy.
Data Privacy and Security Concerns
Data privacy in AI is a pressing concern, given the reliance on outsourcing for data labeling. Safeguarding data privacy and security is essential. Many opt for on-premise labeling to avoid third-party access and ensure data protection. Ensuring data privacy in AI is vital for building trust and adhering to regulatory standards.
- Implement clear and detailed guidelines for annotators.
- Choose qualified and experienced annotators.
- Conduct rigorous quality assurance to ensure high consistency.
- Utilize multiple annotators for accuracy and consensus.
- Employ automated tools to assist and enhance the annotation process.
- Maintain continuous communication and provide feedback to annotators.
Future Trends in Image Annotation
The evolution of image annotation is rapidly reshaping how AI models are trained and deployed across various industries. As we look ahead, several key trends are emerging that promise to drive significant advancements in this field.
Automation and AI Integration
One of the most transformative trends is the integration of advanced AI technologies in the annotation process. Automation is increasingly replacing human annotators through machine learning techniques such as transfer learning and few-shot learning. Automatic Data Labeling (auto-labeling), for instance, leverages heuristic methods and AI models to efficiently identify and tag images. This trend is critical as it significantly reduces manual effort, cuts costs, and accelerates the data annotation pipeline.
Further, innovations like real-time annotation capabilities are becoming vital for applications that require immediate data processing and decision-making, such as autonomous driving and surveillance. The interplay of AI in annotation is set to foster enhanced accuracy and efficiency, making it a cornerstone of the future of image annotation.
Advancements in Annotation Tools
The continuous advancements in annotation tools are greatly improving user experience and output accuracy. Technologies like 3D annotation, using LiDAR (Light Detection and Ranging), are now prevalent in diverse sectors including automotive, medical imaging, and geospatial mapping. These tools provide detailed insights by capturing complex details where traditional 2D annotation falls short.
Also, data augmentation techniques are significantly impacting how training datasets are expanded and diversified. Methods such as geometric transformations, color space augmentation, and neural style transfer not only augment the dataset but also improve model generalizability and mitigate overfitting. These advancements in annotation tools are playing a key role in refining the process of creating high-quality datasets.
Evolving Industry Standards
Standards around data privacy and security, as well as ethical considerations to address biases in AI, are becoming more stringent. Industry-specific standards are being developed to tailor annotation processes to the unique needs of sectors like healthcare, retail, and automotive. This enhances the effectiveness and accuracy of AI applications in these domains.
These evolving standards are vital for promoting trust and reliability in AI systems. They drive the future of image annotation towards more standardized and robust practices.
FAQ
What is High-Precision Image Annotation?
High-precision image annotation is the meticulous process of labeling features in images. This enhances the accuracy of AI models through high-quality data labeling. It's essential for training computer vision systems to effectively recognize and interpret digital images.
What are Image Annotation Services?
Image annotation services involve tagging or labeling digital images. This is done so that machine learning models can be trained to recognize similar patterns. It's critical for applications in computer vision, allowing algorithms to learn from labeled data.
Why are Image Annotation Services important in AI and Machine Learning?
Image annotation services are essential in AI and machine learning. They provide the foundational data required for training models. High-quality annotations ensure the development of accurate AI models. This facilitates robust object detection, image recognition, and semantic segmentation.
What are the different types of Image Annotation Services?
We specialize in various types of image annotation services to meet diverse needs: - Bounding Box Annotation: Vital for projects requiring object localization within clear boundaries. - Polygon Annotation: Used for objects with irregular shapes needing precise boundary definitions. - Semantic Segmentation: Involves labeling each pixel of an image for detailed contextual understanding.
How are Image Annotation Services applied in different industries?
Expert image annotation services have broad applications: - Healthcare: Enhances diagnostic accuracy through imaging. - Autonomous Vehicles: Improves navigation and safety features. - Retail and E-commerce: Optimizes operations through advanced e-commerce analytics and AI-driven insights.
What are the benefits of using professional Image Annotation Services?
Professional image annotation services offer several benefits: - Improved Data Quality: Achieved through meticulous verification processes. - Faster Turnaround Times: Streamlined workflows and advanced tools expedite the process. - Scalability: Efficiently handle large datasets to keep AI projects on track.
How can I choose the right Image Annotation Service?
Selecting the right service involves evaluating the provider's expertise and experience. Also, consider the technology and tools utilized, and their reputation through customer reviews and case studies. This ensures the service meets the specific demands and quality standards of your AI projects.
What is the Image Annotation Process?
The process includes several critical steps: - Data Preparation: Selecting and organizing images. - Annotation Techniques: Applying methods such as bounding boxes or semantic segmentation. - Quality Assurance: Conducting rigorous checks to ensure accuracy and reliability.
What factors influence the cost of Image Annotation Services?
Several factors affect cost: - Complexity of the Project: More complex annotations require more effort. - Volume of Images: Larger datasets may have a higher cumulative cost. - Turnaround Time Requirements: Faster deliveries can incur additional charges.
What are the common challenges in Image Annotation?
Image annotation faces challenges like: - Variability in Image Quality: Affects annotation accuracy. - Subjectivity in Labeling: Can lead to inconsistencies. - Data Privacy and Security Concerns: Ensuring sensitive data is protected.
What future trends are shaping Image Annotation?
Trends include: - Automation and AI Integration: Enhances efficiency in annotation processes. - Advancements in Annotation Tools: Improve user experience and accuracy. - Evolving Industry Standards: Promote best practices and standardization.
Can you provide examples of successful implementations of Image Annotation?
Case studies illustrate the impact: - Healthcare AI Development: Revolutionizes diagnosis and treatment plans. - Improving Retail Checkout Systems: Enhances efficiency and accuracy. - Enhancing Automotive Safety Features: Promotes safer navigation and dynamic response using annotated datasets.
Comments ()