Introduction To AI In Finance
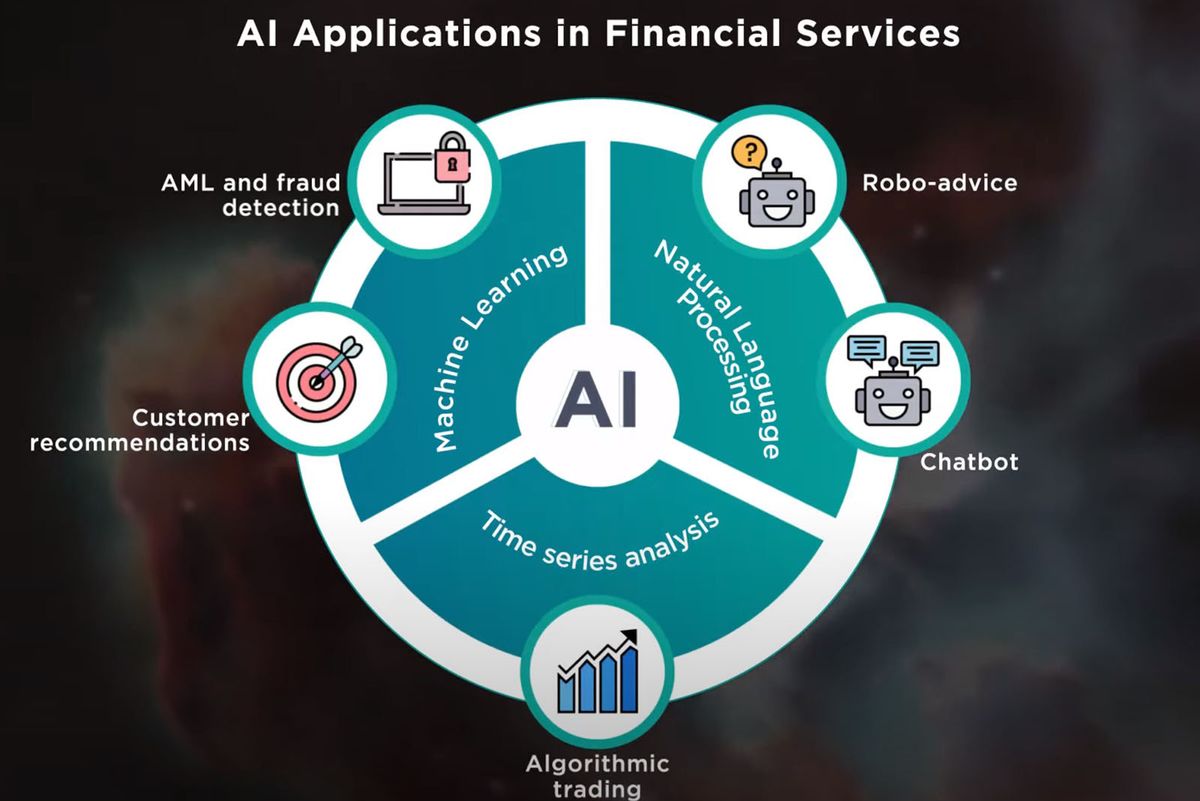
Artificial intelligence (AI) has been making waves in the finance industry, transforming the way financial institutions operate and serve their customers. From fraud detection to investment management, AI is being used to streamline processes, reduce costs, and improve customer experiences. But what exactly is AI, and how is it being used in finance?
In this article, we’ll explore 10 use cases of AI in finance and their potential effects. We’ll start with an introduction to AI in finance and its benefits, followed by specific examples of how AI is being used in fraud detection and prevention, credit scoring and risk assessment, trading and investment management, customer service and personalization, insurance claims processing, regulatory compliance, and more.
We’ll also discuss the potential effects of AI on employment in finance and ethical considerations that must be taken into account. Finally, we’ll look at the future of AI in finance and what it means for the industry as a whole. Whether you’re a finance professional or just curious about the impact of AI on the industry, this article is for you.
AI is quickly becoming an integral part of the financial sector, bringing about both challenges and opportunities. Financial institutions are investing heavily in AI technologies to streamline processes, improve customer experiences, and mitigate fraud and cyber attacks. By 2030, FinTechs anticipate that AI will have expanded their workforce by 19%.
One of the most significant business cases for AI in finance is its ability to prevent fraud and cyberattacks. Financial institutions are leveraging machine learning algorithms to detect fraudulent activity, thus reducing costs associated with security breaches. Moreover, banks with over $100 billion in assets are increasingly implementing AI technologies; 75% of them are already using them.
Financial forecasting and market performance comparison are other areas where AI is finding success in finance. By predicting market trends and making data-driven decisions on investments or trading strategies, financial institutions can stay ahead of the competition.
AI models used in quantitative finance must be easily understandable to regulators and clients alike; explainable AI (XAI) is gaining traction as a result. Additionally, a growing number of banking leaders are taking a comprehensive approach to deploying advanced AI solutions across multiple departments within their organizations.
Overall, it's clear that the impact of AI on financial institutions will continue to grow exponentially over time. As such, it's important for these organizations to stay informed about emerging technologies and develop strategies accordingly if they want to remain competitive well into the future.
AI In Fraud Detection And Prevention
Fraud is a growing concern for businesses, with 72% of business leaders citing it as a problem. This is where AI-powered fraud detection and prevention comes in. AI-based tools analyze data in real-time to identify and predict instances of fraud, making it more efficient compared to manual detection.
Advanced analytics can prevent, predict, detect, and remediate fraud by analyzing patterns within big data. The analysis of real-time threat detection can process data on a live basis to detect threats in real-time. With the use of natural language processing (NLP) algorithms and machine learning techniques like supervised/unsupervised learning, AI helps organizations with risk assessment.
AI helps financial institutions drive the future of finance by using predictive modeling to reduce potential losses by detecting fraudulent activity before it happens. Even artificial intelligence algorithms from banks that have already encountered cyber-crimes become better equipped at identifying anomalous customer behavior since they learn from case-specific evidence.
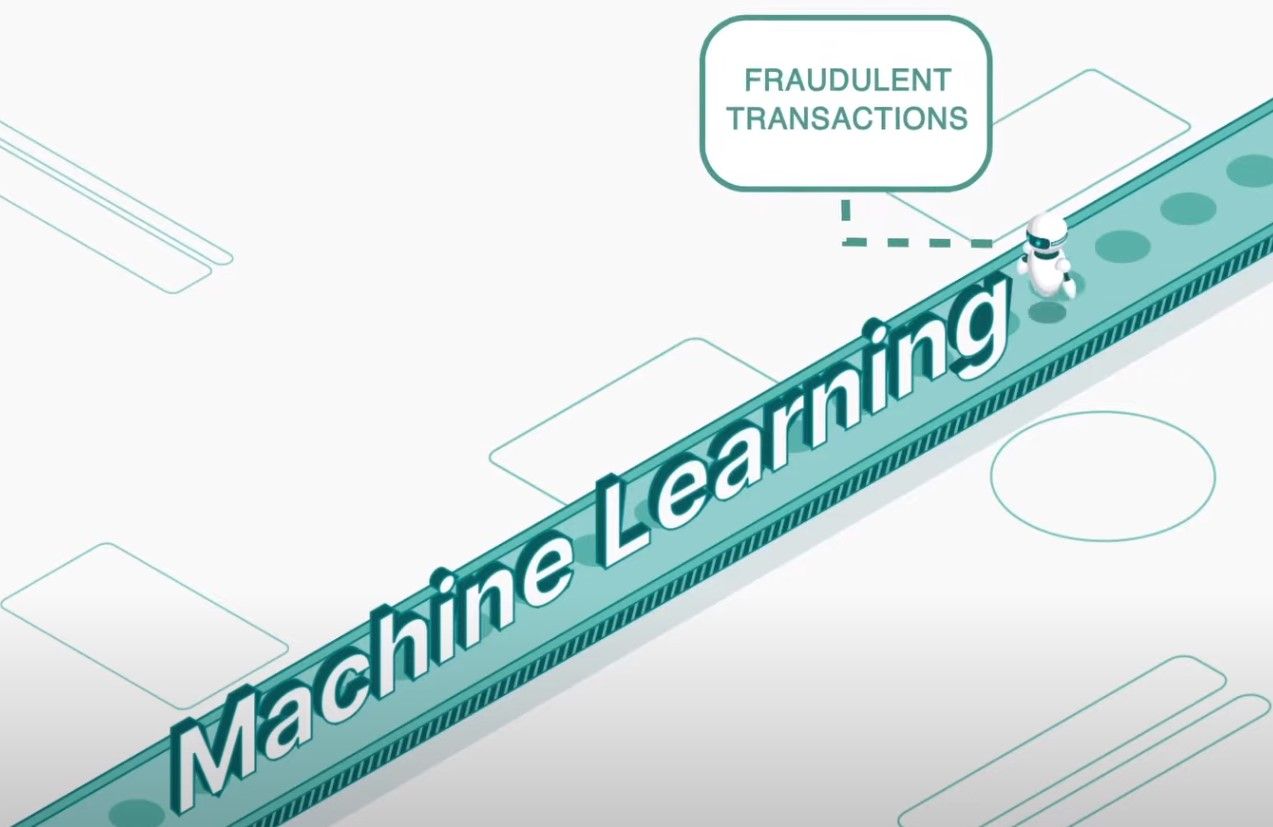
AI in fraud-detection helps financial service providers investigate risks through advanced analytics models that detect fraudulent attempts at different stages across various channels where machines are trained on vast amounts of past information containing identify theft incidents or other irregularities to improve analysis or augment human decision-making.
AI-based tools for fraud detection bring comprehensive insight into every aspect of an organization's security posture through instant alerts concerning abnormal transactions across multiple channels - alerting analysts when treading down certain paths if necessary - all while reducing errors resulting from traditional methods that previously relied solely on human surveillance capability during business hours only.
AI In Credit Scoring And Risk Assessment
AI is quickly improving the credit scoring and risk assessment process in the finance industry. With its ability to quickly analyze large quantities of data, AI has become an invaluable tool for discovering new risk segments or patterns. Through AI-driven assessments, lenders can better analyze borrowers' financial activities and reduce risks associated with loans and credit fraud.
Alternative data sources, such as social media and internet activity, are also being explored for creditworthiness assessments. By using unconventional data points in addition to traditional sources (e.g., credit history), lenders could more accurately determine a borrower's likelihood to default on a loan or make timely payments.
In terms of automating credit decisions with AI, studies have shown that it can outperform traditional scorecards. This not only improves efficiency and productivity but also reduces costs in risk management. Generative AI also has potential in conversational finance by producing more natural language responses during interactions between customers and virtual assistants.
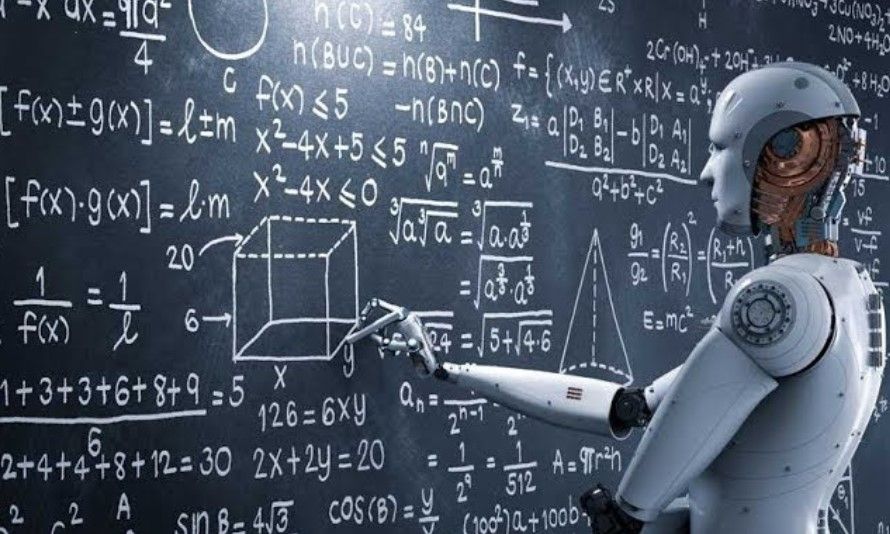
Overall, incorporating AI into the credit scoring and risk assessment process offers many benefits for both lenders and borrowers. It helps mitigate risks while enhancing accuracy, efficiency, and productivity in an industry where time is crucial.
AI In Trading And Investment Management
AI is revolutionizing the trading and investment management industry, providing analysts with the ability to research and extract data at lightning speed. Three types of AI applications are being used in this industry: natural language processing, computer vision, and voice recognition. These applications can analyze huge amounts of complex data faster than humans could ever accomplish. Investment firms are using AI to detect patterns in the market which allow them to predict future market values.
Moreover, AI technology has the potential to transform how credit and risk are allocated within financial systems resulting in a fairer and more inclusive system. By using machine learning, banks can predict cash flow events and adjust credit scores accordingly to reflect a more accurate assessment of risk factors. Additionally, automation through AI is possible leading to faster and more efficient tasks when it comes to financial applications.
Leveraging artificial intelligence has significant potential benefits for businesses operating in the finance sector when it comes to trading and investment management. By implementing machine learning technologies into their operations, asset managers can gain access not only to vast amounts of complex data but also quickly analyze them effectively on a global scale. As such early adopters hope that these investments will lead over time widespread use turning into more robust systems as operations become streamlined through automation upending legacy business models along the way while hopefully benefiting all participants including positive effects on society as a whole alike through increased efficiency reducing costs ultimately releasing higher returns over time creating a virtuous circle of economic growth from which many would potentially benefit both inside or even beyond finance sector likely resulting various side achievements such as improvements towards achievement for sustainable development goals set globally aimed at raising quality living standards worldwide bringing society forward into an altogether better future for all involved parties across international borders.
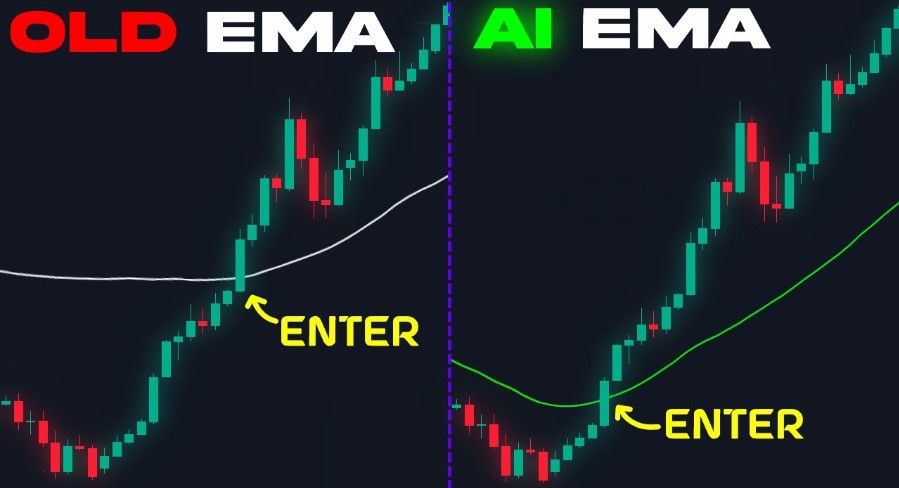
AI In Customer Service And Personalization
AI has revolutionized the way finance teams interact with their customers. One of the most significant advantages of AI-driven insights in customer service is hyper-personalization. With access to data such as customer churn prediction/prevention, CLV estimation, marketing optimization, customer segmentation and personalization - AI can be used for smarter interaction between businesses and customers. This use case can lead to increased engagement, improved customer loyalty, higher sales figures and better understanding of your clients.
Chatbots have also been instrumental in improving robo-advisory services for portfolio management. By providing 24/7 support through chatbot interactions, customers can access personalized help without waiting for a human advisor response. Additionally, finance teams are using AI-based tools for improving other forms of customer service in the industry – providing faster response times and even freeing up available time to work on more strategic tasks.
The benefits of using AI-driven insights continue into middle office functions such as risk management, fraud detection & compliance monitoring - as well as supporting operations like streamlining process flows and eliminating human error/false positives. Customers can expect enhanced experiences when it comes to banking or financial transactions thanks to intelligent optimizations brought forth by advanced systems powered by artificial intelligence technology.
AI In Insurance Claims Processing
Artificial Intelligence (AI) has been gaining traction over the years and its application in the insurance industry is no exception. AI has a wide range of applications, especially in insurance claims processing. According to CCC Information Services, 46% of AI vendors provide solutions for claims while 43% offer solutions for underwriting. This technology can increase efficiency, reduce cycle time and improve customer experience.
Insurance companies have suffered from unsatisfied customers leading to a risk of $170 billion in premium over the next five years. Therefore, integrating AI solutions with human intervention could help solve this challenge by improving customer experience through speed and accuracy. This process will also streamline the workflow which will lead to less time spent on administrative tasks.
AI can also improve fraud detection since bots are programmed to learn different patterns of risks such as fraudulent claims and thus can flag off suspicious activity. Fukoku Mutual Life Insurance has already implemented AI solutions in detecting fraudulent claims resulting in a cost-saving measure which mainly involved reducing manpower costs.
AI integration with humans remains critical for any insurer looking to benefit from increased efficiency in processing claims within an insurance company. Once an insurer decides to leverage these technologies there's plenty of room for improvements that will impact both internal processes and external stakeholders involved in the wider ecosystem such as customers or partners who work together with them towards achieving common goals.
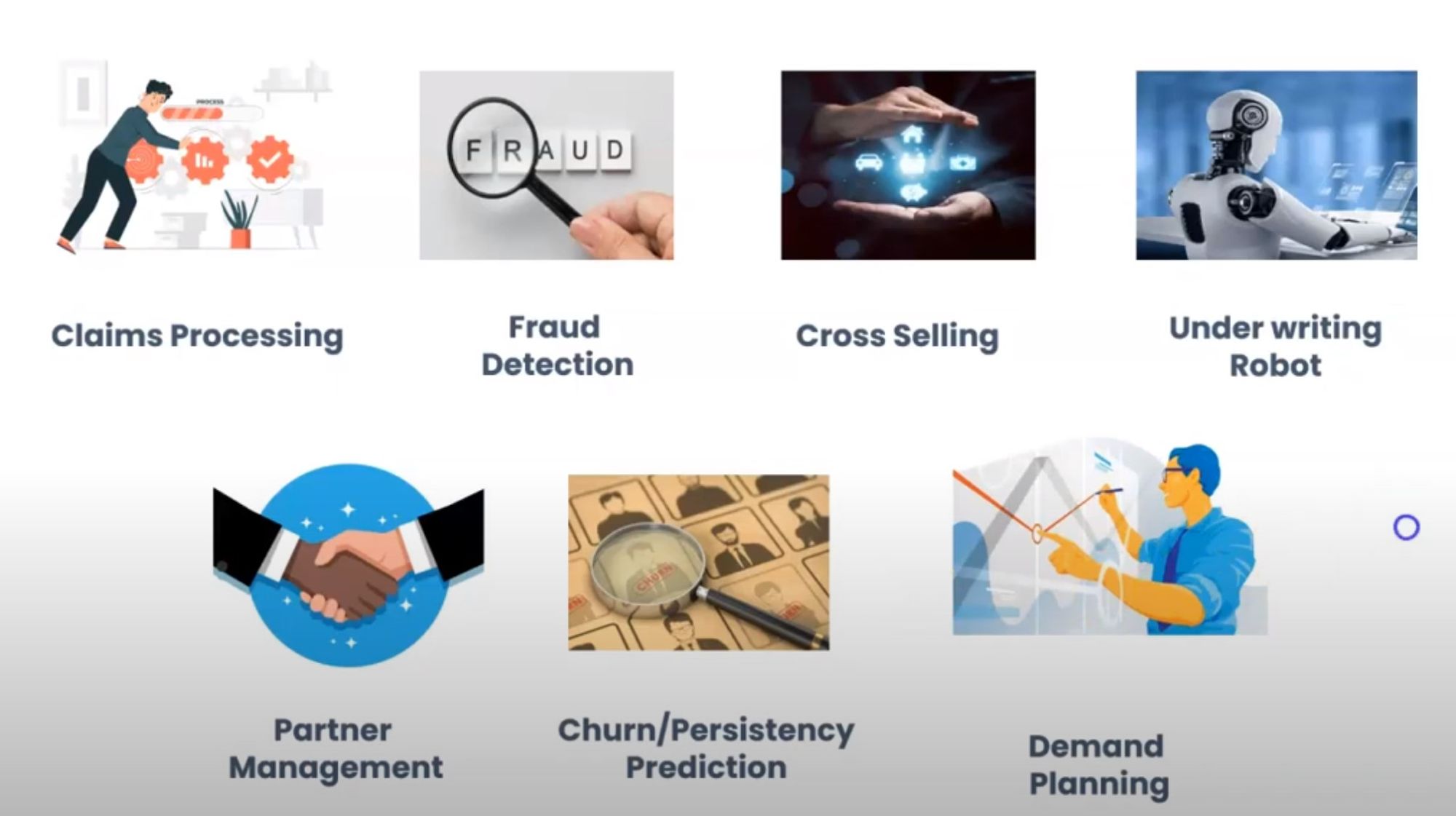
AI In Regulatory Compliance
AI is a game-changing technology that has the potential to transform regulatory compliance in finance. A key use case for AI is in anti-money laundering (AML) and sanctions screening, where it can help financial firms address challenges such as hefty fines for non-compliance. With its ability to process large volumes of data with speed and accuracy, AI can enhance the effectiveness of screening processes and ensure regulatory compliance.
Data governance is another vital AI and ML use case for corporate finance. By using intelligent algorithms to manage data sources, identify missing or incorrect information, reduce errors, and optimize decision-making processes, financial firms can improve their overall data quality. This can increase productivity while minimizing operational risk.
As AI continues to drive regulatory compliance efforts in finance, including initial regulations implemented in 2022 and more general initiatives expected in 2023 by the European Commission imposing fines up to 6% of company's annual revenues for noncompliance with AI regulation- it creates an opportunity for financial institutions to innovate across various aspects of their operations from risk management & alert management systems down to fraud prevention.
The potential benefits are significant: streamlined processes, reduced operational costs through increased efficiency & productivity as well as mitigated risks due breaches and noncompliance; all leading towards a more rigorous system of checks-and-balances benefiting consumers. As with any transformative innovation - there will be challenges along the way but it’s clear that AI-driven advancements are already delivering an improved experience within financial services which makes these exciting times indeed.
Potential Effects Of AI On Employment In Finance
AI is set to transform the finance industry, potentially increasing efficiency and reducing costs, but what will be the impact on employment? Some experts predict that it will be similar to the effect of spreadsheets on the industry. While AI may lead to job losses in certain areas, it also has the potential to create new roles and opportunities for workers with technology skills.
Studies have shown that exposure to AI is associated with lower growth in hours worked in occupations where computer use is low. From 2012-19, while overall employment increased across OECD countries, the average number of hours worked per worker declined. However, the impact of AI on the finance industry's labor market, including employment and wages, is still being studied.
Financial institutions are already investing heavily in AI technologies as they realize its potential to generate more than $250 billion in value by applying it to their financial processes. In fact, a survey found that 75% of respondents at banks with over $100 billion in assets are implementing AI technologies. This suggests that there could be a shift towards more highly skilled jobs such as data analysis and programming as firms seek employees with expertise in these areas.
It remains unclear how widespread job losses will be due to AI automation; however, many firms are beginning to realize that retraining employees for higher-skilled positions may be one solution. It's clear that change is coming quickly for finance workers who must stay competitive by developing essential technical skills if they want to remain relevant during this technological revolution.
Ethical Considerations Of AI In Finance
With the increasing adoption of AI in finance, there are growing concerns about its ethical implications. Privacy and surveillance, bias and discrimination, and the role of human judgment are three major areas of ethical concern raised by AI in finance. For example, AI algorithms can contribute to systemic risks in finance without reflecting on its ethical implications. Therefore operationalizing data and AI ethics requires identifying existing infrastructure and creating an ethical risk framework.
Legal personality for AI systems or robots is fundamentally inconsistent with human agency, accountability, and responsibility. Moreover, algorithmic bias poses a particular threat to financial institutions as it can create new types of risks for businesses such as amplifying biases or leading to opaque decisions. The use of AI in investment management must be addressed while advancing ethical practices.
To move forward with the inclusion of this technology, companies have to understand its risks and ethical considerations fully. Transparency is imperative when introducing any new technology that affects people's lives drastically. One critical way financial institutions can ensure they remain ethically conscious about their use of data analytics is by mandating ethic boards that help analyze their system for potential violation against one's privacy rights while shooting down any unwanted moves against human security concerns caused by malicious or hidden functionality within the software structure themselves during production stage itself at regular intervals.
Future Of AI In Finance And Conclusion
The AI market for fintech is projected to reach $27 billion in the next five years, and there are many potential use cases of AI in finance. However, it is important to note that implementing AI can also lead to an increase in wicked and legacy problems, such as discriminatory pricing and exclusionary filtering.
Some of the top AI use cases in finance include generative AI, natural language understanding/generation (NLU/NLG), and reducing repetitive work. These technologies can enable a more frictionless customer interaction, save money for financial institutions by automating processes like loan approvals or fraud detection systems.
AI can enhance customer experience by providing personalized recommendations based on their spending habits and investment behavior. It can detect fraudulent transactions and assess risks better than humans ever could. Furthermore, over 70% of financial firms are using machine learning algorithms to predict cash flow events and adjust credit scores.
Banking institutions stand to gain significant value through the integration of artificial intelligence technologies. In fact, banking firms alone could generate over $250 billion by applying these tools effectively. By prioritizing potential use cases at a business level, building cross-functional teams with technical experts, data analysts ,and business representatives streamlined decision making processes at scale.
While there are significant benefits from implementing AI solutions within the fintech industry such as client satisfaction improvements or fraud prevention strategies which contribute greatly towards driving revenue but they must be handled carefully so as not to create or exacerbate ethical issues where existing biases within datasets cause discriminatory trends emerge."
Comments ()