Jumpstart Your Freelance Career as a Data Annotator
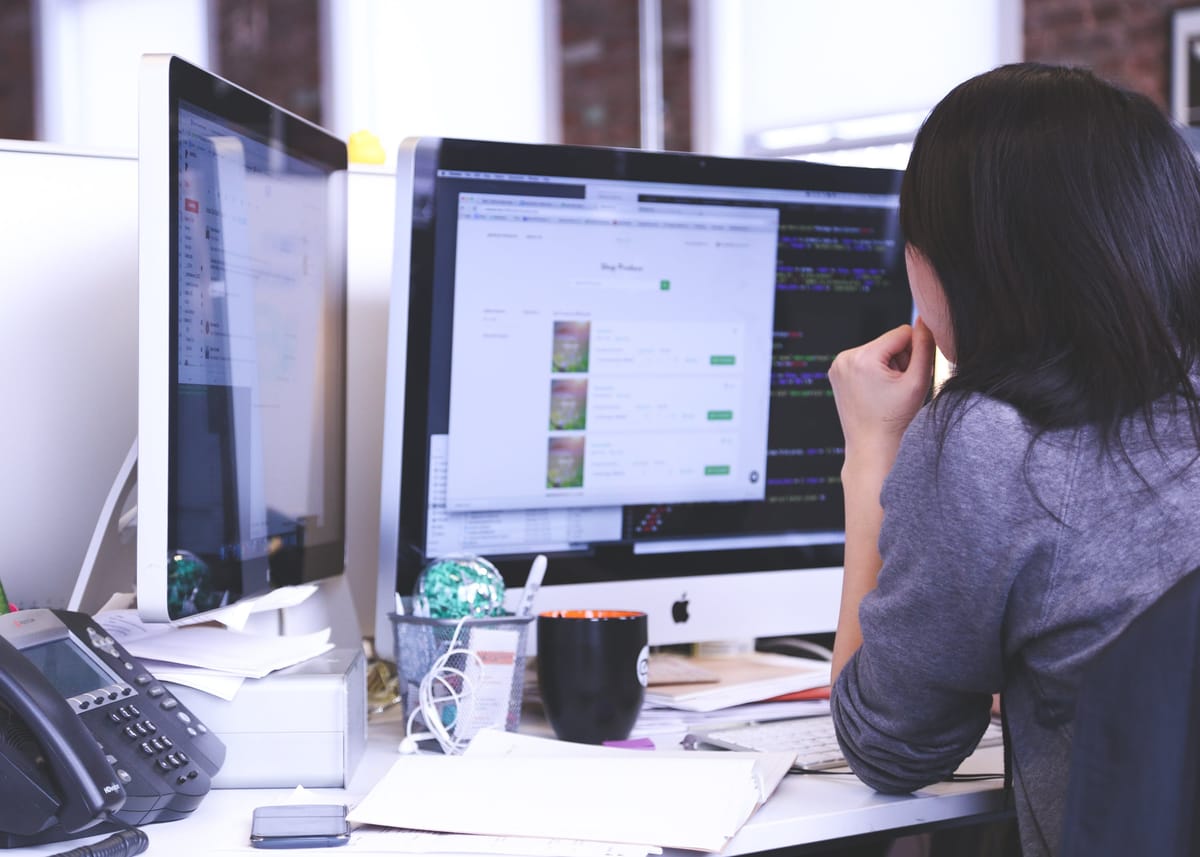
Data annotation is a crucial task in the field of AI and machine learning. As a data annotator, you have the opportunity to kickstart your freelance career in this fast-growing industry. In this article, we will explore the essentials to excel as a freelance data annotator and provide valuable insights to help you succeed in this field.
Create an abstract representation of data annotation, using colors and shapes to convey the concept of organizing and labeling large amounts of information. Focus on the idea of precision and accuracy, using geometric patterns to suggest order and structure. Avoid any literal or figurative interpretations of data, instead emphasizing the process of annotation itself. Use overlapping shapes and gradient colors to create depth and dimension in the image.
Key Takeaways
- Data annotation is a crucial task in AI and machine learning.
- Freelancing as a data annotator offers great career opportunities.
- Developing the right skills and using the right tools is essential for success.
- Networking and building an online presence can help you find freelance projects.
- Following best practices and continuously learning are key to excel in data annotation.
What is Data Annotation?
Data annotation is the process of labeling data to train AI and machine learning models. It involves tasks such as image annotation, where objects or features are marked in images, and text annotation, where entities or sentiments are tagged in text documents. Data annotation plays a crucial role in improving the accuracy and performance of AI systems. It requires attention to detail and a strong understanding of the data domain to ensure high-quality annotations.
"Data annotation is the foundation upon which AI models are built. It provides the necessary labeled data that enables machine learning algorithms to understand and process information accurately."
The Importance of Data Annotation in AI and Machine Learning
Data annotation plays a vital role in the development and enhancement of AI and machine learning models. By accurately labeling and annotating data, these models can effectively learn patterns and make accurate predictions and decisions. The process of data annotation involves tasks such as object detection, sentiment analysis, speech recognition, and more, which are essential for training AI systems. Without proper data annotation, AI models may struggle to understand and interpret input data correctly, leading to inaccurate and unreliable results. Therefore, data annotation is a critical step in the AI and machine learning pipeline, enabling the creation of reliable and high-performing models.
Skills and Qualifications for Data Annotation
To excel as a data annotator, certain skills and qualifications are required. These include:
- A strong attention to detail: Data annotation requires meticulous focus and accuracy to ensure high-quality annotations.
- Knowledge of different data annotation techniques and tools: Familiarity with a variety of annotation techniques, such as image and text annotation, and proficiency in using data annotation tools are essential.
- Familiarity with AI and machine learning concepts: Understanding the fundamentals of AI and machine learning helps in comprehending the significance of data annotation in training models.
- The ability to work with large datasets: Data annotators often deal with extensive datasets, so having the ability to manage and process large volumes of information is crucial.
- Good communication skills: Effective communication with clients, team members, and project stakeholders is important for clarifying requirements and ensuring accurate annotations.
- The ability to follow guidelines and instructions: Adhering to annotation guidelines provided by clients or projects ensures consistency and accuracy in labeling.
- Domain knowledge: Depending on the industry you are working in, having specific domain knowledge — such as medical terminology, e-commerce products, or automotive features — can be advantageous.
Various data labeling services, tools, and companies are available to support data annotators in their work. These resources provide training, guidelines, and platforms to assist annotators in effectively carrying out their tasks.
Finding Freelance Data Annotation Opportunities
As a freelance data annotator, there are several ways to find opportunities. One option is to join data annotation platforms or companies that connect annotators with clients. These platforms provide a steady stream of projects and handle the administrative aspects of freelancing, such as payments and client communication.
Another option is to network with AI and machine learning professionals and companies directly to find freelance projects. By reaching out to individuals and organizations in the industry, you can discover potential opportunities and showcase your skills as a data annotator. Building a strong online presence, such as through a portfolio or website, can also help attract potential clients.
Show an image of a person sitting in front of a computer, with various AI tools and software visible on the screen, as they meticulously label and categorize different types of data. The person should look focused and productive, surrounded by charts and graphs that visually represent the data they are working with. The scene should have a futuristic feel to it, with sleek lines, bold colors, and sophisticated technology visible throughout. The overall mood should be one of efficiency, productivity, and accuracy, capturing the essence of what it means to be a freelance data annotator working with cutting-edge AI tools.
Essential Tools for Data Annotation
Data annotation is a task that requires the use of various tools to efficiently label and annotate data. By leveraging these tools, data annotators can enhance their productivity and accuracy in the annotation process. Some popular data annotation tools that are widely used in the industry include:
- Labelbox
- Prodigy
- Amazon Mechanical Turk
These tools offer a range of features and functionalities that cater to different types of data annotation tasks. They provide capabilities for image annotation, text annotation, and collaboration among annotators. When used effectively, these tools can significantly streamline the data annotation workflow and improve the quality of the annotated data.
Best Practices for Data Annotation
As a data annotator, following best practices is essential to excel in data annotation. By adhering to these practices, you can ensure the quality and accuracy of your annotations, leading to successful AI and machine learning models.
1. Understand Annotation Guidelines
One of the first steps in data annotation is to thoroughly understand the annotation guidelines provided by the client or project. These guidelines outline specific requirements for labeling data, such as the labeling conventions and annotation criteria. By familiarizing yourself with these guidelines, you can ensure consistency and alignment with the project's objectives.
2. Ensure Consistent Labeling
Consistency in labeling is crucial to maintain data integrity and train accurate models. When labeling a dataset, make sure to apply consistent annotations across similar data points. This includes using the same labels for identical objects or entities, ensuring uniformity in naming conventions, and maintaining consistent formatting or tagging standards.
3. Maintain High Accuracy
Precision and accuracy are vital in data annotation. Aim to deliver annotations with a high level of accuracy, avoiding mistakes or misinterpretations. Double-check your annotations to identify any errors or inconsistencies and rectify them promptly. Regularly validating annotations with quality checks or peer reviews can also help maintain a high level of accuracy.
4. Regularly Review and Validate Annotations
It is crucial to review and validate annotations regularly throughout the annotation process. By frequently reviewing your annotations, you can identify potential errors, inconsistencies, or ambiguities and take corrective actions. Validation procedures such as cross-validation or inter-annotator agreement assessments can help ensure the quality and reliability of the annotated dataset.
5. Collaborate and Communicate
Effective collaboration and communication with clients, project managers, or team members are vital for successful data annotation. Regular communication ensures clarity and alignment with project expectations, addressing any questions or clarifications promptly. By actively engaging with the project stakeholders, you can foster a collaborative environment and deliver annotations that meet their requirements.
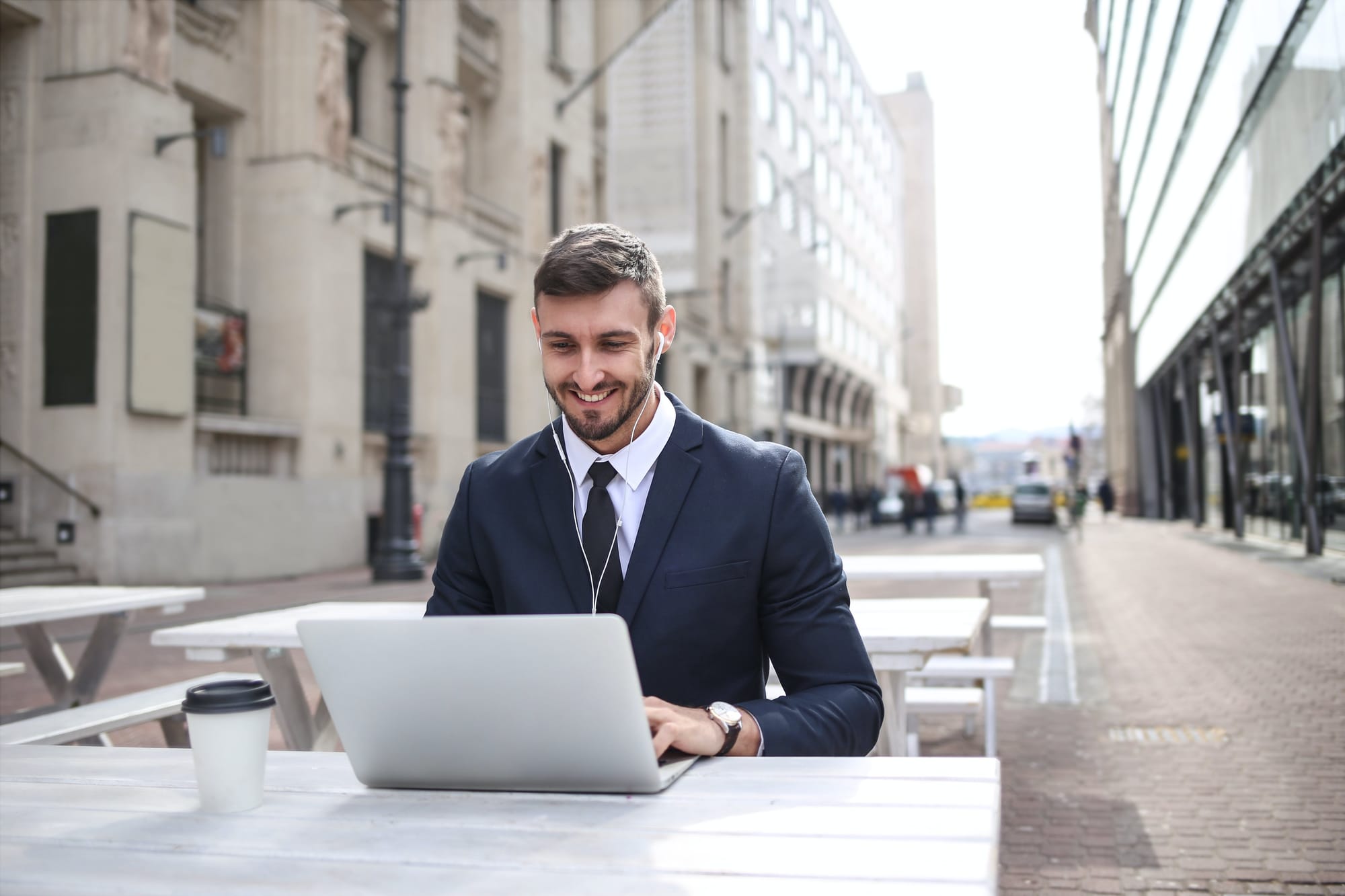
Create an image showing the process of data annotation in AI. Include elements such as a computer screen, a mouse, and various shapes and colors representing different types of data. Show the annotations being made with precision and accuracy. Use vibrant colors to emphasize the importance of this task in training artificial intelligence.
Challenges and Opportunities in Data Annotation
Data annotation, while presenting exciting opportunities for freelancers, also brings forth its fair share of challenges. The task of dealing with large and complex datasets can be daunting, requiring meticulous attention to detail and a deep understanding of the data domain. Additionally, managing tight deadlines and handling diverse annotation requirements can put considerable pressure on data annotators. However, these challenges can also be seen as opportunities for growth and development in the field of data annotation. By continuously learning and staying updated with new techniques and methodologies, data annotators can overcome these challenges and enhance their skills to excel in this dynamic industry.
Challenges in Data Annotation
Data annotation often involves working with large and complex datasets, which require meticulous attention and expertise. Ensuring accurate and consistent labeling across the dataset can be a significant challenge, especially when dealing with intricate details and ambiguous data. Moreover, managing tight deadlines while maintaining high-quality annotations requires a careful balance of efficiency and accuracy. It is crucial for data annotators to adapt to diverse annotation requirements, as different projects may demand specific labeling techniques or guidelines.
Opportunities for Growth
Despite the challenges, data annotation presents opportunities for freelancers to refine their skills and expand their expertise. Continuous learning and staying updated with new techniques and methodologies can elevate the quality of annotations and improve the overall performance of AI and machine learning models. By embracing challenges, data annotators can gain valuable experience and establish themselves as trusted professionals in the field. As the demand for AI data annotation continues to grow, there are ample opportunities for freelancers to contribute to groundbreaking projects and make a significant impact in the industry.
Conclusion
Data annotation is a dynamic and thriving field that offers great opportunities for freelancers. By developing the necessary skills, leveraging the right tools, and following best practices, you can jumpstart your freelance career as a data annotator. With the increasing demand for AI and machine learning solutions, the need for high-quality annotated data will continue to grow. So, take the leap, embrace the challenges, and embark on your journey as a freelance data annotator in this exciting industry.
FAQ
What is data annotation?
Data annotation is the process of labeling data, such as images or text, to train AI and machine learning models.
Why is data annotation important in AI and machine learning?
Data annotation is crucial in AI and machine learning as it improves the accuracy and performance of models by providing accurately labeled data for training.
What skills and qualifications are required for data annotation?
Data annotation requires attention to detail, familiarity with AI and machine learning concepts, and the ability to work with large datasets. Good communication and following guidelines are also essential.
How can I find freelance data annotation opportunities?
You can join data annotation platforms or companies, network with professionals, or showcase your skills through a portfolio or website to attract clients.
What are some popular data annotation tools?
Popular data annotation tools include Labelbox, Prodigy, and Amazon Mechanical Turk.
What are some best practices for data annotation?
Best practices include understanding the annotation guidelines, maintaining consistency, and regularly reviewing and validating annotations.
What are the challenges and opportunities in data annotation?
Challenges include dealing with large datasets and tight deadlines, but these challenges can also be opportunities for growth and skill development.
How can I jumpstart my freelance career as a data annotator?
By developing the necessary skills, leveraging the right tools, and following best practices, you can kickstart your freelance career as a data annotator in this thriving industry.
Comments ()