Machine learning hardware that can be trained with an optical chip
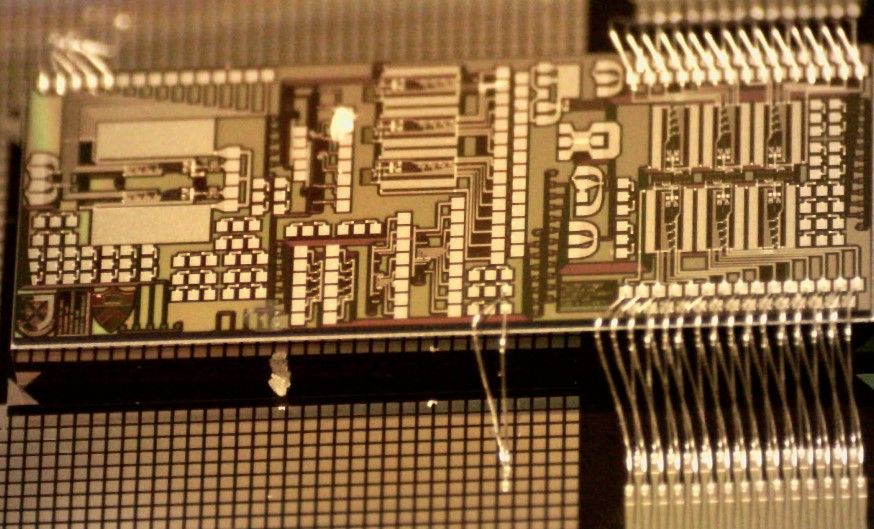
Using a chip developed by a multi-institutional research team, machine learning hardware can be trained. The research was published in Optica 23.11.2022.
A recent McKinsey report estimates that machine learning applications are worth $165 billion annually. However, before a machine is able to perform intelligence tasks such as recognizing details in an image, it must be trained. It costs several million dollars to train modern artificial intelligence (AI) systems such as Tesla's autopilot, which consumes a large amount of electricity and requires infrastructure comparable to that of a supercomputer.
There is a growing gap between computer hardware and the demand for artificial intelligence as a result of this surging AI "appetite.". A possible solution to increase computing performance has emerged through the use of optical integrated circuits, or optical chips. TTOPS/W, or the number of operations executed per second per watt consumed, is a measure of computing performance.
Despite the fact that photonic chips provide improved core functions in machine intelligence, they have yet to improve the actual training and learning process at the front end.
The process of machine learning involves two steps. In the first step, the AI system is trained using data, and in the second step, the system is tested using data.
Once a team member observed an error, a second training cycle was initiated, followed by additional training cycles until a satisfactory level of AI performance had been achieved (e.g., the system can correctly identify objects in a movie).
Up to this point, photonic chips have demonstrated only the capability of classifying and inferring information from data. Due to this, researchers have developed a method for accelerating the training process.
ASICs utilizing photonic chip manufacturing for machine learning and artificial intelligence are part of a larger project involving photonic tensor cores and other electronic-photonic application-specific integrated circuits (ASICs).
It represents a major advance in AI hardware acceleration by combining photonics and electronic chips in a way that will significantly speed up the training of machine learning systems.
The training of artificial intelligence systems generates a significant amount of energy and carbon footprint.
For example, one AI transformer consumes approximately five times more carbon dioxide than a gasoline car over its lifetime. It has been estimated that the overhead associated with training on photonic chips can be reduced, according to Bhavin Shastri, Assistant Professor of Physics Department Queens University.
Src: George Washington University
Comments ()