Manual Data Annotation vs. Augmented Annotation: Pros and Cons
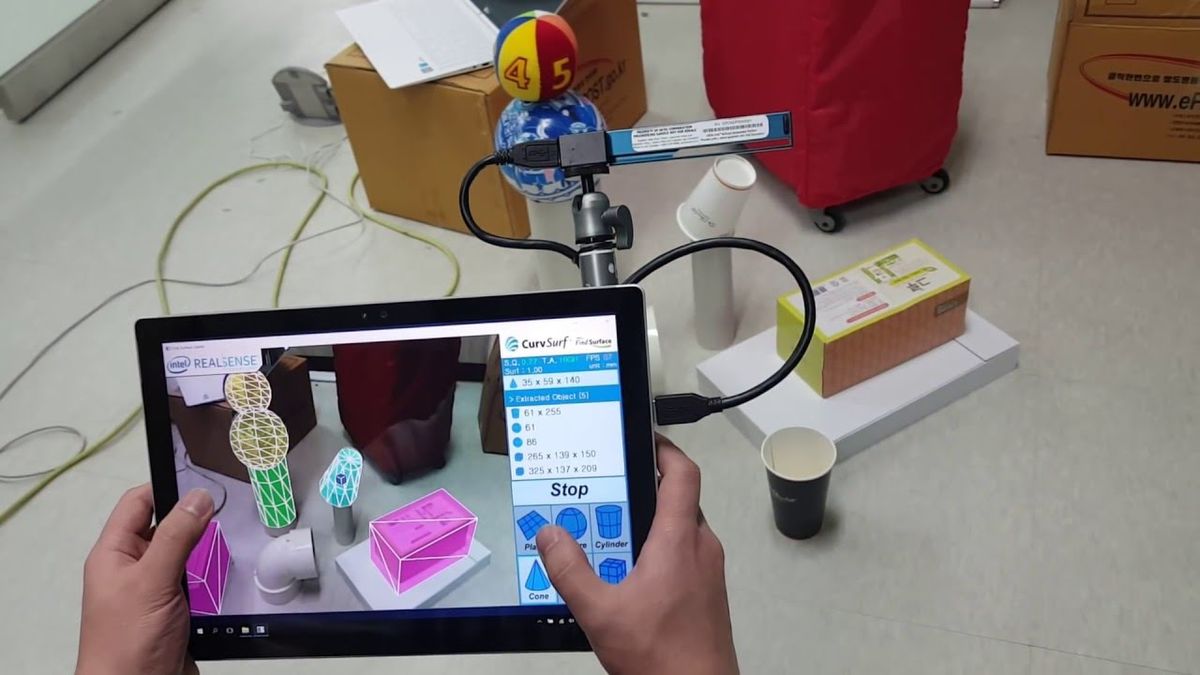
Data annotation is the process of labeling data to make it understandable for machines. It is a crucial step in machine learning, natural language processing, and computer vision. However, data annotation can be a time-consuming and labor-intensive process. To address this issue, two methods have emerged: manual data annotation and augmented annotation.
In this article, we will explore the pros and cons of each method, and compare them to help you choose the best approach for your data annotation needs. We will discuss the factors to consider when selecting between manual and augmented annotation, and provide best practices for effective data annotation. Additionally, we will examine future trends in data annotation techniques, so you can stay ahead of the curve.
Whether you are a data scientist, a machine learning engineer, or a business owner looking to automate your processes, this article will provide valuable insights into the world of data annotation. So, let's dive in and explore the world of manual data annotation vs. augmented annotation!
Understanding Manual Data Annotation
Manual data annotation is an important process used in NLP experiments that involves the manual labeling of data by human annotators. It is a time-consuming process that can be regarded as a bottleneck for NLP experiments. Despite the slow process, manual labeling is the only option for tasks that require direct training or high-level domain knowledge. This makes it essential to choose the right data annotation tool.
The criteria for choosing an effective manual data annotation tool includes factors such as efficiency, functionality, formatting, application and price. Furthermore, through exclusive annotation during manual labeling, rights to data and models are claimed with ease.
On the other hand, automated data labeling is faster and less expensive than manual annotation; however it can pose challenges with accuracy depending on how complex or specific the task requirements are. Outsourcing work related to manual annotations offers several benefits such as cost savings on labor costs , ensuring quality control and scalability including timely availability improving turnaround times when meeting peak demands of runs or higher volume studies.
Regarding Augmented Annotations - this type of ground truth dataset creation has been used in recent years due to its accuracy compared to traditional methodologies which tend to neglect region relationships found within images themselves and allows you not only greater precision but also improved performance may result from large datasets produced by these methologies alone as welll thus contributing one more technique researchers use when annotating their models with labeled datasets generated through augmented annotations workflows.
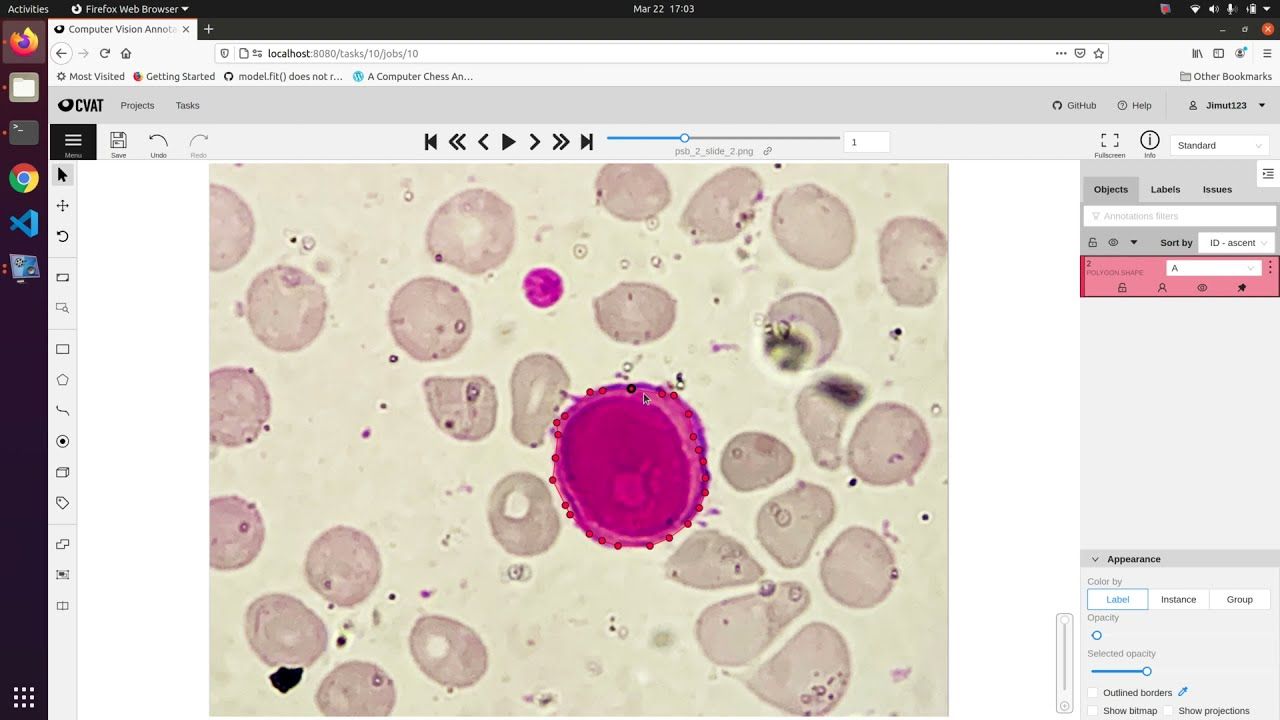
The Pros And Cons Of Manual Data Annotation
Manual data annotation is a time-consuming process that involves adding metadata to datasets by human annotators. This method allows for greater control and accuracy in data labeling but can also lead to poor quality due to human errors. However, with proper training and standardized guidelines, manual annotation can ensure high-quality, accurate, and consistent metadata.
One major advantage of manual annotation is the ability to capture complex contextual information that automated tools may miss. Human annotators can make connections between concepts, identify nuances in language use, and provide additional context that machines cannot easily pick up on. Additionally, by using multiple annotators and cross-checking annotations, manual data labeling can increase inter-annotator agreement and improve overall accuracy.
On the other hand, there are several drawbacks to using manual data annotation. The primary issue is the possibility of inconsistencies or errors due to variations in interpretation or mistakes made during the labeling process. These mistakes can lead to mislabeled data which can have significant implications in downstream applications such as machine learning models.
Overall, while there are pros and cons associated with manual data annotation, it remains an essential tool for generating high-quality training datasets for machine learning algorithms. By combining rigorous training programs with standardized protocols for labeling and quality control measures such as cross-checking annotations between multiple annotators before integrating them into a final dataset version can reduce inconsistencies essentially reducing errors better than automated methods alone could achieve in many cases.
Understanding Augmented Annotation
Augmented annotation is a procedure that can be utilized for training and testing machine learning models. This annotation system is not closely tied to any particular annotation tool, allowing for more flexibility in the process. Automated data annotation saves time and money compared to manual annotation, as it requires less human intervention.
The quality of the data labels has an impact on the machine learning model prediction accuracy. Consistency and quality of automated labels presents a challenge, requiring human review to maintain accuracy.
Outsourcing data annotation tasks is an option that provides cost savings, scalability opportunities, and increased data security since it can be done remotely with minimum involvement from employees. Hiring third-party companies gives businesses access to skilled professional annotators who work efficiently with multiple clients while meeting strict deadlines.
Augmented labeling offers faster solutions than manual approaches which allows businesses across different industries to save time and resources while reducing human error; nevertheless consistent labeling remains key in order to maintain high-quality datasets for models. Additionally outsourcing offers businesses flexibility while also accessing professional help in getting their datasets labeled according to their specific needs at affordable costs.
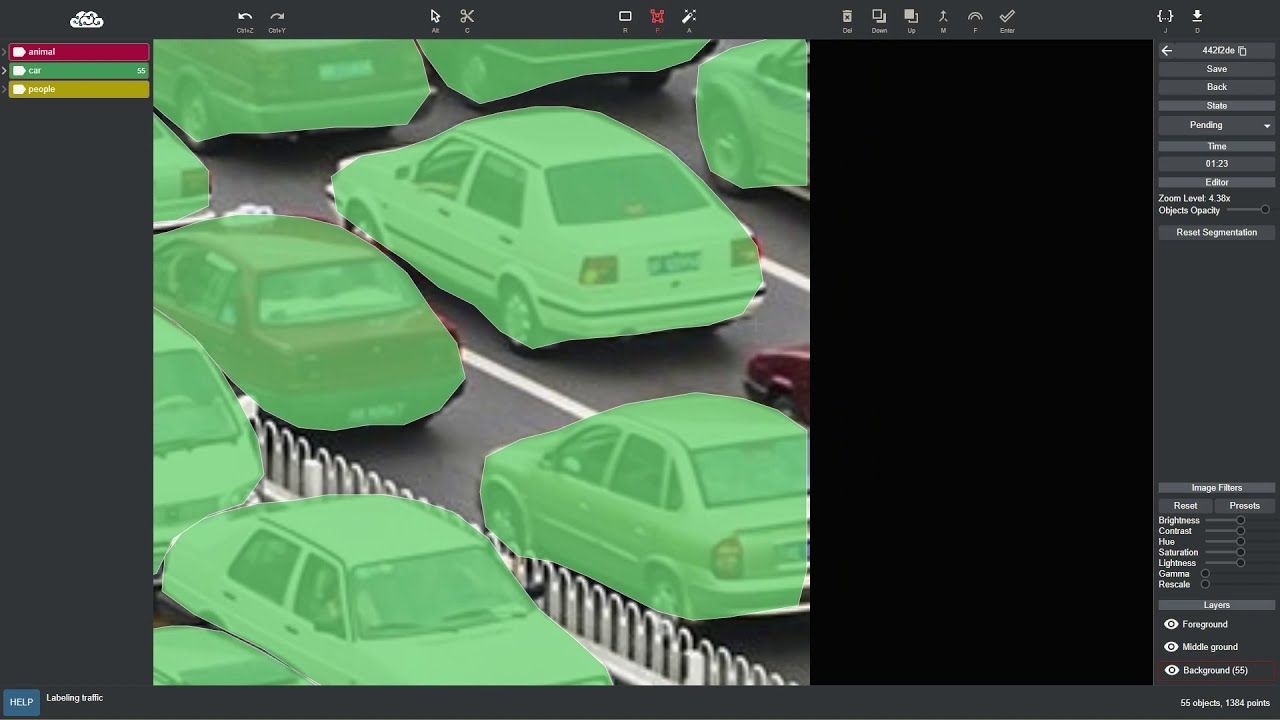
The Pros And Cons Of Augmented Annotation
Augmented annotation, or automated data labeling, is an alternative to manual data annotation that offers several benefits. One of its main advantages is increased speed and cost-effectiveness. Automated tools can label large amounts of data quickly and more affordably than manual methods. This makes it a better option for businesses with tight budgets or those dealing with vast quantities of data.
Automated methods can also increase precision by minimizing human intervention. Algorithms can detect patterns in the data and generate labels based on these patterns, which can help improve learning and model accuracy. Additionally, augmented annotation is effective in cases where high-level domain knowledge is not required because the algorithms can process images in bulk without being influenced by subjective factors.
However, like any technology, augmented annotation has its drawbacks as well. Lack of human oversight increases the risk of errors since software programs solely rely on rules-based algorithms designed by experts in a specific field who may miss essential features outside their expertise or fail to recognize some complex structures captured during image acquisition such as textures and microstructures that humans easily identify with their eyes.
Moreover, augmented annotation may not be suitable for more complex tasks that require deep understanding and analysis from specialists' viewpoint; hence they would need manual labor input to fulfill quality through diversity requirements such as inconsistencies checks, ensuring semantics tags are accurate among other details.
Therefore before committing to either method business stakeholders should evaluate their goals about what they hope to accomplish when provided with additional human labor input (manual annotations) versus rapid throughput AI-driven operations techniques (augmented annotations).
Comparing Manual Data Annotation And Augmented Annotation
Manual annotation of data is a time-consuming and monotonous task, which can become a bottleneck in the process of NLP experiments. However, human annotators provide more accurate labeling as compared to algorithms. Crowdsourcing platforms may prioritize quantity over quality when it comes to data annotation. Outsourcing the task to a reliable data annotation service provider can save costs while ensuring high-quality work.
Manual data annotation requires significant effort and maintenance of data quality, which can be challenging. Using an augmented annotations tool like automation and cognitive skills could improve the efficiency of manual annotations, saving time and improving accuracy. Labeled datasets are essential in supervised machine learning for input pattern comprehension.
Augmented annotations help in empowering humans with automation tools that streamline time-consuming tasks using artificial intelligence technology. Automation follows complex pre-defined rules that use natural language processing technology to recognize patterns within text boxes and select relevant features with high accuracy levels that match predefined criteria.
Manual data annotation is still valuable for high-quality training datasets required for supervised machine learning models where precision is critical. Augmented Annotations are essential when you want to quickly scale your training dataset or are comfortable sacrificing some precision for faster results at scale without compromising on quality. By leveraging both methods, organizations can strike a balance between cost-saving measures and ensuring accurate training datasets for their NLP model's best performance possible across various applications from sentiment analysis, chatbots/voice assistants interpretation & recognition so enterprises or business users can achieve better decision-making outcomes faster than before!
Factors To Consider When Choosing Between Manual And Augmented Annotation
When choosing between manual data annotation and augmented annotation, there are several factors to consider. Efficiency, functionality, formatting, application, and price are the key criteria for choosing the right tool. Manual labeling is meticulous and done by experts with years of experience. This process ensures high accuracy rates and customized results. However, it can be time-consuming and poses privacy and security issues as sensitive data needs to be shared with annotators.
Augmented annotation can provide labeled ground-truth data for training machine learning models quickly. It is preferred when large datasets need to be labeled in a short amount of time. While augmented annotation is an automated process that saves time, it may not always generate accurate results required for some complex tasks such as medical imaging or facial recognition.
The choice between manual annotations or augmented annotation also depends on the task at hand; some tasks like image classification require precise labeling from experts while others like sentiment analysis consume vast amounts of user-generated content which can be gathered through crowdsourcing platforms like Amazon Mechanical Turk. To leverage both efficiency and expertise in labeling data two popular methods “human-in-the-loop” or “active-learning" combine human effort (manual) with intelligent algorithms (augmented) resulting in more accurate annotated datasets useful for training AI models. While manual testing discovers vulnerabilities through targeted efforts evaluating specific functions, automated testing assures rapid detection using algorithms that facilitate smart scanning leveraging scripts developed by trusted hacking communities around the world.
No matter what method you choose ultimately depends on cost considerations relative to available resources from your company’s budget allocated towards labor vs technology adoption balance which continue to change over long periods necessitating regular re-evaluation of these choices.
Best Practices For Effective Data Annotation
Data annotation is an essential part of creating a machine learning model, and here are some best practices to follow for effective annotation. Firstly, defining an annotation schema and writing comprehensive guidelines is critical. Guidelines provide clarity for annotators on how to approach the task and ensure accuracy and consistency across the dataset.
Pre-processing documents before annotation is another best practice that can save time. This involves tasks like pre-formatting data or identifying specific sections within documents where annotations are required. Additionally, choosing the best method for collecting annotations is also crucial; options include crowdsourcing, expert annotators, or outsourcing to professional services.
It's important to train annotators to perform a task accurately rather than just providing instructions; this training may involve several rounds of revisions on annotated data before building consensus between annotators. One challenge with manual data annotation is cost versus accuracy; balancing these factors depends largely on whether cost savings outweighs a slight decrease in accuracy.
Finally, automated data labeling workflows and synthetic data are increasingly popular methods of reducing manual labor costs while maintaining high-quality datasets. It's essential to implement appropriate retention policies alongside compliance regulation requirements when handling sensitive information like personal health records (PHR), financial records (FR) or other confidential business datasets.
Overall effective annotation workflows involve setting clear guidelines that promote consistency between experts, leveraging automated processes when possible without sacrificing accuracy or incurring additional risks due to errors introduced during automation processing steps such as prediction errors caused by Machine Learning models trained using less accurate labeled samples than human experts would have produced individually done by humans themselves.
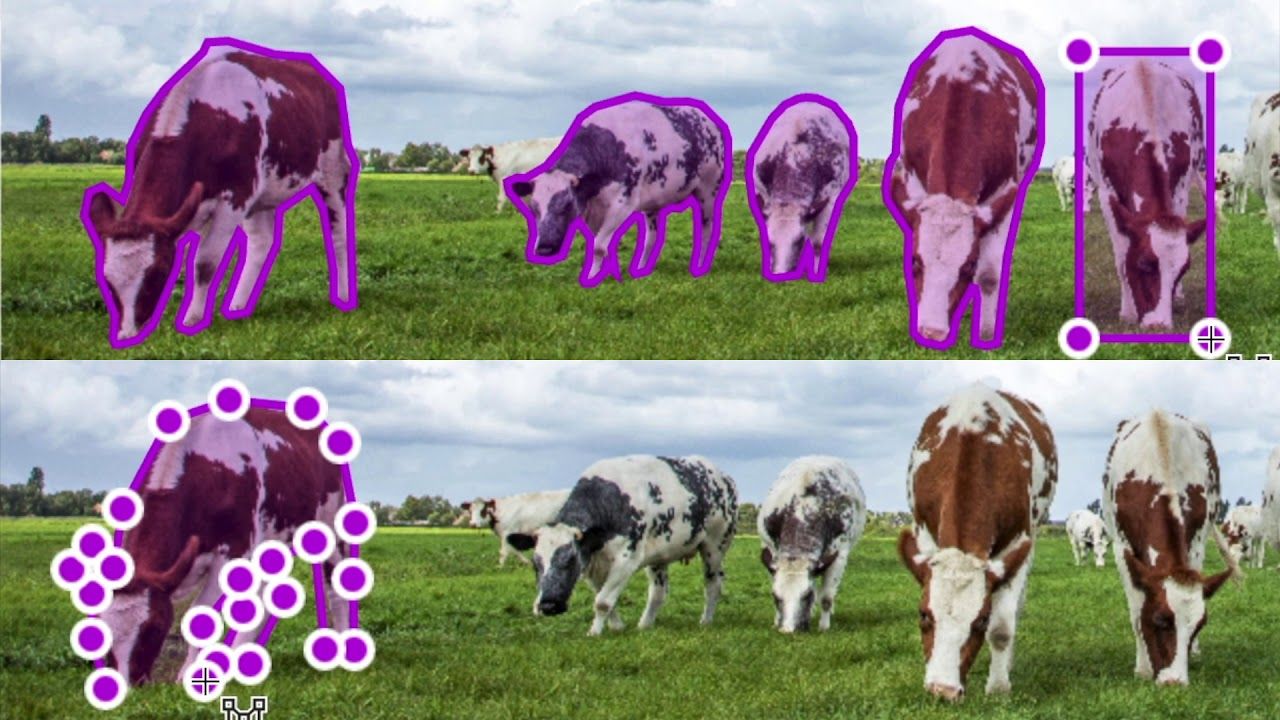
Future Trends In Data Annotation Techniques
Automated data labeling is gaining popularity due to its efficiency and accuracy. The market size of data labeling tools is expected to grow at a rate of over 30% annually between 2021 and 2027. This rising demand for high-quality and accurate data labeling is for computer vision (CV) and natural language processing (NLP) tasks.
One emerging trend in data annotation techniques is image and video annotation, which trains CV systems. Manual annotation, however, is becoming an unpopular choice because it is time-consuming and expensive. Instead, there are many image annotation techniques that seek to find correlations between words and image features such as color, shape, texture to provide an automatically correct annotation.
Furthermore, augmenting training sets with synthetically generated data can help overcome the lack of training data. Augmented Annotation has become increasingly popular as it offers several benefits compared to manual annotation methods or using un-augmented datasets alone. It can improve accuracy by providing additional overlaps on the same object or changing the lighting in synthetic images while adding diversity to the dataset - producing higher quality annotations.
Overall, technological advances mean that automated data labeling will continue to define future trends in Data Annotation Techniques- more specifically around Augmented Annotation combined with Image/Video Annotation AI capabilities offering safe cost reductions through time saved annotating accurately by achieving better quality results avoiding errors caused by human fatigue .
Conclusion
In conclusion, data annotation is an essential component of machine learning and artificial intelligence, and both manual and augmented annotation methods have their pros and cons. While manual annotation offers greater accuracy and control, it can be time-consuming and expensive. Augmented annotation, on the other hand, offers greater speed and scalability, but may not always be as accurate.
When choosing between manual and augmented annotation, it is important to consider factors such as the complexity of the data, the size of the dataset, and the available resources. By following best practices for effective data annotation and staying up-to-date with future trends in annotation techniques, businesses and organizations can make informed decisions about which method to use for their specific needs.
Comments ()