ML Data Annotation Enhancing E-commerce Industry
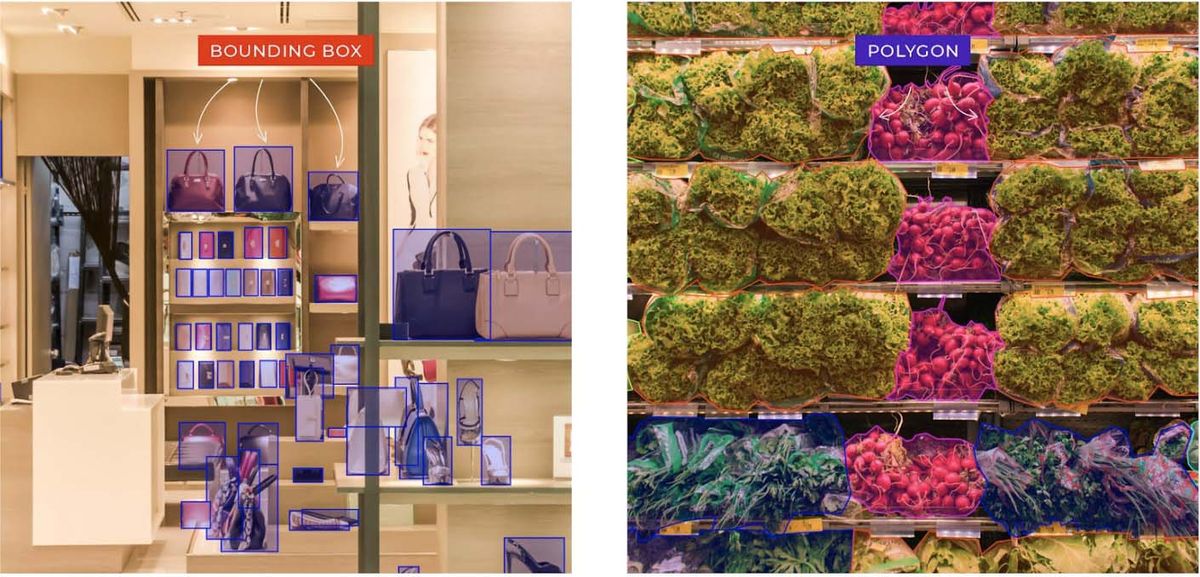
Machine learning (ML) has become an integral part of the e-commerce industry. It enables businesses to make more informed decisions and improve operations. At the heart of this technology is data annotation. The process labels and categorizes data to make it more useful for ML algorithms.
Accurate ML data annotation is crucial for the effectiveness of ML algorithms. It helps these algorithms understand and analyze data. In e-commerce, this can translate into better-personalized product recommendations. It can also help you with targeted marketing campaigns and more efficient operations.
But, data annotation in the e-commerce industry comes with its challenges. Businesses must navigate obstacles, from handling large datasets to maintaining consistent labeling standards.
This article explores the importance of accurate ML data annotation in e-commerce. You'll also learn about some of the challenges and best practices. Finally, you'll discover use cases that drive growth and improve operations.
The importance of accurate ML data annotation
Accurate data annotation is crucial for the effectiveness of ML algorithms. Without labeled and categorized data, algorithms cannot analyze and understand the information. So, you may receive unsatisfactory results and make poor decisions.
Accurate data annotation in the e-commerce industry can lead to many benefits. For example, well-annotated data can help improve personalized product recommendations. In addition, it can enable you to predict more about what products a customer will buy.
Accurate data annotation can enable businesses to create more targeted marketing campaigns. It allows you to understand and segment your customer base. As a result, marketing efforts are more effective and have higher conversion rates.
Challenges and best practices for ML data annotation in e-commerce
Data annotation in the e-commerce industry is not without its challenges. One common issue is the sheer volume of data that you must annotate. E-commerce businesses often deal with large datasets. Manually annotating all this information can be a time-consuming and tedious task.
Ensuring that all annotators are following the same guidelines can be challenging. Another difficulty is maintaining consistent labeling standards. You need to label and categorize data uniformly. This step is crucial to ensure that ML algorithms can understand and analyze it.
E-commerce businesses can turn to automated annotation tools to overcome these challenges. These tools help speed up the annotation process. They also ensure that data is consistent. But not relying only on automation is essential. Only using automated tools can sometimes produce inaccurate results.
Establishing clear guidelines for annotators ensures that data is accurate and consistent. An example includes outlining specific labeling standards. In addition, you could provide training and resources to annotators. Finally, remember to review and update guidelines regularly.
The key to annotation in e-commerce is combining automated tools with human oversight.
Use cases for successful data annotation in e-commerce
There are many ways e-commerce businesses can implement ML data annotation.
You can use data annotation to improve your personalized product recommendations. One example is in retail fashion. First, you could label and categorize customer purchases and browsing history data. Then, you could predict what products each customer will buy. This process could lead to increased sales and customer satisfaction.
Another example is an online marketplace. You can use data annotation to create targeted marketing campaigns. First, you need to label data on customer demographics and purchasing habits. Then, you could segment your customer base and create more effective marketing campaigns. You could start to see higher conversion rates and business growth.
These use cases illustrate the power of accurate data annotation for ML in e-commerce. By labeling and categorizing data, businesses can harness the full potential of ML.
Successful data annotation in e-commerce requires ongoing effort and attention to detail. But, implementing data annotation is not a one-time task. First, businesses must review and update annotated data. This way, you ensure that it is accurate and up-to-date. Also, you should review your annotation guidelines in line with industry changes.
Conclusion and future outlook
ML data annotation tools will continue to advance. The e-commerce industry continues to grow and change. Businesses that leverage data annotation are well-positioned to capitalize on machine learning.
In conclusion, data annotation is a crucial part of the machine-learning process. Labeling and categorizing data can improve the effectiveness of ML algorithms. The results are personal product recommendations, targeted marketing campaigns, and more efficient operations.
Data annotation in the e-commerce industry comes with challenges. Some include handling large datasets and maintaining consistent labeling standards. However, businesses can overcome these challenges. Turn to automated annotation tools and establish clear guidelines for annotators.
Accurate data annotation is essential if you're an e-commerce business. It improves your machine-learning algorithms and drives growth. Keylabs' state-of-the-art data annotation tool lets you quickly label and categorize your data.
Comments ()