Optimize AI Systems with Specialized Logistics Data Annotation
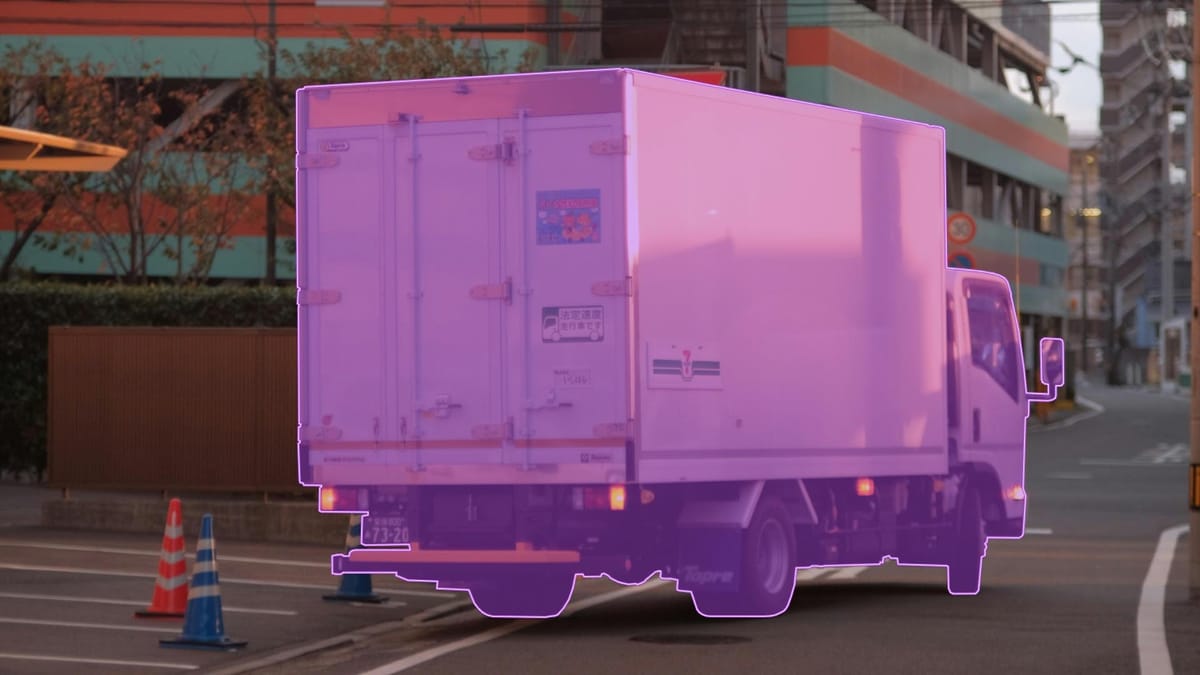
Specialized logistics data annotation plays a vital role in the industry. It transforms raw data into actionable AI insights, ensuring AI systems in logistics are trained with high-quality data. This process is tailored to the unique challenges of logistics, such as inventory management, shipment tracking, and route optimization.
By focusing on these areas, businesses can improve AI model reliability. This leads to better decision-making and optimized workflows. These are critical for achieving AI logistics optimization.
Key Takeaways
- Specialized logistics data annotation is essential for introducing AI and ML systems in logistics.
- Annotated data is critical for self-driving cars, drones, and warehouse robots in making decisions.
- Barcode and label detection aids in inventory management through computer vision systems.
- Predictive maintenance relies on sensor data annotation to reduce downtime.
- Traffic data annotation helps optimize routes by predicting traffic patterns and road conditions.
Understanding Logistics Data Annotation
Logistics data annotation is the systematic process of labeling logistics-related data to make it understandable for AI and ML models. This essential process involves annotating various types of data, such as images from warehouses, text from shipping documents, and videos from transit routes. It enhances AI’s ability to learn and predict outcomes accurately. By understanding the Definition and Importance of logistics data annotation, companies can tailor their AI and ML models more effectively.
Key components of logistics data include inventory levels, shipment details, and transport conditions. Accurate data annotation ensures AI systems can handle these components efficiently. This leads to more reliable logistics operations.
Challenges in data annotation in logistics include the high volume of data, the complexity of logistics scenarios, and maintaining data privacy and security. To illustrate, let's dive into the types of logistics data annotations:
- Image and Video Annotation
- Object Detection in Warehouses: Enhances accuracy in locating inventory, packages, and vehicles. AI models can track misplaced items and organize warehouse space efficiently.
- Fleet Monitoring: Tracking trucks, shipping containers, and assets in real-time elevates safety measures.
- Autonomous Vehicles: Labeling road signs, objects, and traffic lights for autonomous trucks improves traffic behavior and safety.
- Text Annotation
- Document Processing: Automating invoices, shipping orders, and contracts processing improves efficiency and compliance issues.
- Customer Support: Labeling inquiries and feedback enhances customer service through intelligent agents and chatbots.
- Sensor Data Annotation
- IoT Sensor Data: Analyzing data from sensors in vehicles, shipping containers, and warehouses enhances maintenance schedules and optimizes routes.
- Route Optimization: Adding GPS coordinates, weather conditions, and traffic flow data helps in determining the best routes for delivery trucks.
- Geospatial Data Annotation
- Mapping and Route Planning: Adding road types, distance, and speed limits on maps helps in determining optimal delivery routes.
- Delivery Zones and Heatmaps: Utilizing heat maps improves last-mile delivery efficiency and customer satisfaction.
Understanding Definition and Importance in the logistics context helps us tackle these challenges. It facilitates smooth annotation processes that ultimately bolster the AI’s performance in logistics operations. With the extensive data involved, a precise approach to logistics data annotation is key for achieving successful AI outcomes in the logistics industry.
Types of Logistics Data Annotations
Image Annotation in Logistics is vital for improving logistics efficiency and accuracy. It caters to specific needs within the logistics sector. Each type of data annotation serves a unique purpose.
Image Annotation: This involves labeling elements in images to aid automated systems in recognizing and categorizing items. For instance, annotating images of warehouse shelves enhances inventory management. Annotated images and datasets are essential for quality control and automation, where precision is critical.
Text Annotation: Used for shipping documents, text annotation classifies and extracts critical information. It includes shipment terms, item descriptions, and customer details, streamlining documentation processes. Proper text annotation ensures better compliance and quicker decision-making.
For more insights on how data annotation supports machine learning, refer to data annotation for machine learning models.
Video Annotation: Crucial for transport tracking, video annotation enhances the accuracy of monitoring and security. It involves complex techniques like Multi-Object Tracking (MOT) and Auto Object Segmentation to maintain quality while speeding up the process.
The global data annotation tools market size was $1.02 billion in 2023 and is expected to grow at a CAGR of 26.3% from 2024 to 2030 (Grand View Research). This growth highlights the increasing need for accurate and efficient data annotation across sectors, including logistics.
Here's a comparative overview of different logistics data annotation types:
Type of Annotation | Application | Tools & Techniques |
---|---|---|
Image Annotation | Inventory Management, Quality Control | Model-assisted labeling, HPE tracking |
Text Annotation | Shipping Documents, Compliance | Automated Text Classifiers, NLP |
Video Annotation | Transport Tracking, Security | MOT, Auto Object Segmentation |
Benefits of Logistics Data Annotation
Logistics data annotation brings numerous advantages. It boosts enhanced accuracy in AI models Streamlined operations come from better resource management and workflow automation. This ensures products move through the supply chain faster and with fewer mistakes.
- Enhanced product categorization improves search engine visibility and streamlines operations.
- Efficient supply chain operations enable on-time delivery, price optimization, and client satisfaction.
- Annotated data is essential for AI efficiency in image recognition and managing inventory.
- Real-time filtering of stock levels and locations leads to better forecasting accuracy.
- Optimized routing plans result in faster deliveries, reduced shipping costs, and increased customer satisfaction.
- Minimization of manual tasks and errors leads to financial savings and better operational performance.
- Data annotation is vital for training AI algorithms and building AI-powered applications.
Artificial intelligence can optimize routing plans by tracking annotated information, resulting in faster deliveries, reduced shipping costs, and increased customer satisfaction.
Data annotation in logistics provides valuable insights for making informed decisions. It helps anticipate disruptions and adapt to market changes. For example:
Aspect | Impact |
---|---|
Demand Forecasting | Enhanced accuracy in AI models ensures precise inventory predictions. |
Resource Management | Streamlined operations ensure efficient allocation and utilization of resources. |
Customer Satisfaction | Faster deliveries and reduced errors lead to improved customer experiences. |
Operational Costs | Automation reduces labor costs and human errors, saving financial resources. |
Data-Driven Decisions | AI-driven insights guide strategic decision-making to adapt to market changes. |
Companies like Walmart and Alibaba have seen significant improvements by using logistic data annotation. They've leveraged AI and machine learning to enhance their operations and forecasting. As the logistics sector evolves, the role of data annotation in achieving efficiency and accuracy will remain essential.
The Process of Logistics Data Annotation
The data annotation process in logistics is complex, starting with thorough data collection methods. This data comes directly from logistics operations, ensuring AI models learn from a wide range of inputs. Advanced annotation tools and technologies play a key role in efficiently processing and annotating this data.
Techniques like machine-assisted labeling and crowd-sourcing solutions speed up AI model development and deployment. For example, diverse image annotation methods, including bounding boxes and semantic segmentation, are essential for improving logistics operations. Companies like Amazon and Alibaba use these annotations to implement AI solutions. Amazon's Kiva robot system and Alibaba's Cainiao Network drone delivery services are examples.
Table summarizing key aspects:
Aspect | Details |
---|---|
Data Collection Methods | Collected directly from logistics operations for varied learning. |
Annotation Technologies | Advanced tools such as Docloop, Labelbox, and RectLabel. |
Quality Assurance | Techniques include machine-assisted labeling and human verification. |
Quality assurance is vital for maintaining the reliability and accuracy of annotated data. Validation processes ensure data meets high standards, critical for robust AI applications. For instance, BasicAI Cloud's polygon tools significantly improve warehouse management and other operations.
Data annotation is key across sectors like healthcare, retail, and finance. It enables AI/ML model development, saving time and money, improving precision, and reducing human intervention. This structured approach enhances logistics operations' efficiency and effectiveness, driving innovations like autonomous trucks and intelligent transportation systems.
Best Practices for Effective Annotation
Enhancing AI training models requires adopting best practices in data annotation. Ensuring consistency in data labeling is critical to avoid discrepancies that can mislead AI training. Collaboration with domain experts is invaluable for leveraging specialized knowledge. This ensures that annotations reflect the nuance and complexity of logistics tasks.
Our approach includes regular training and updates for the annotation team. This keeps them current with the latest tools and methodologies in data annotation. Through effective data annotation, we ensure AI training models remain relevant and accurate in a rapidly evolving market.
- Training and Continuous Learning: Regular sessions and updates keep the annotation team aligned with emerging technologies and best practices. This ensures consistent labeling of logistics data.
- Collaboration in Annotation: Engaging with domain experts allows for a deeper understanding of specific logistics tasks. This enhances the accuracy and relevance of the annotations.
- Tools and Techniques: Utilizing top-notch annotation tools is essential for speeding up the training of machine learning models. This improves the workflow, boosts consistency, and reliability of data labeling.
The market for specialized logistics data annotation is expected to grow at a rate of 26.5% annually until 2030. It is set to reach an estimated USD 5,331.0 million. This growth highlights the significance of maintaining high standards in annotation practices. It drives innovation and operational efficiency.
Key Statistic | Insight |
---|---|
26.5% annual growth rate until 2030 | Highlights the expanding market and increasing demand for high-quality annotated data. |
USD 5,331.0 million by 2030 | Signifies the substantial economic impact and importance of accurate logistics data annotation. |
Broad Sector Impact | Data annotation affects healthcare, retail, transportation, gaming, and personalized shopping, beyond machine learning alone. |
Enhanced AI Performance | Quality data annotation leads to improved decision-making, model accuracy, and competitive advantage. |
Enterprises that prioritize these best practices in data annotation are likely to outperform their competitors. They will achieve higher project success rates and model accuracy. By using advanced annotation tools and maintaining rigorous training and update schedules, we can achieve consistency in data labeling. This elevates the impact of AI systems in logistics and beyond.
Industry Applications of Logistics Data Annotation
Logistics data annotation is a game-changer for the logistics and supply chain sectors. It boosts the precision and accuracy of AI models when integrated with Warehouse Management Systems. This leads to better inventory tracking and automated storage optimization, making warehouse operations more efficient.
In last-mile delivery, annotated data is key to improving delivery accuracy and customer satisfaction. AI systems, powered by annotated datasets, can plan the best routes and provide real-time updates. This streamlines delivery processes and enhances the customer experience through timely and precise delivery schedules.
AI models, fueled by annotated data, significantly enhance freight and route optimization. They can accurately plan routes, manage load capacities, and reduce transportation costs. This optimization is vital for staying competitive in the logistics industry.
Data annotation's applications stretch beyond logistics, including healthcare. In healthcare, accurately labeled data is used in clinical diagnostics and procedures. Infosys BPM notes that healthcare enterprises have seen promising results from adopting data annotation tools, leading to better patient outcomes through enhanced AI systems (learn more here).
To summarize, logistics data annotation offers significant benefits:
- Enhanced inventory tracking and storage optimization in Warehouse Management Systems
- Improved accuracy and customer satisfaction in last-mile delivery solutions
- Efficient route planning and load management for freight and transportation
Below is a table highlighting the key advantages and industry applications of logistics data annotation:
Application | Benefits | Industry |
---|---|---|
Warehouse Management Systems | Enhanced inventory tracking, Storage optimization | Logistics & Supply Chain |
Last-Mile Delivery Solutions | Improved accuracy, Customer satisfaction | Logistics & Supply Chain |
Route Optimization | Efficient route planning, Cost reduction | Freight & Transportation |
Clinical Diagnostics | Improved patient outcomes | Healthcare |
Autonomous Vehicles | Precise navigation, Safety | Automotive |
Selecting the Right Annotation Service Provider
Choosing the right annotation service provider is key to optimizing logistics data annotation. It's essential to evaluate experience and expertise of prospective providers. This ensures they understand the unique challenges of logistics data.
When picking annotation providers, comparing their tools and technologies is vital. Companies like People for AI and BasicAI use managed workforces, keeping employees long-term to maintain expertise. In contrast, crowdsourced workforces might be cheaper but face challenges with consistency and complexity due to high turnover.
Scalability for future needs is also a must. Managed workforces generally improve data quality over time and handle large volumes efficiently. A good provider should scale their services to meet growing demands without compromising quality.
Criteria | Managed Workforces | Crowdsourced Workforces |
---|---|---|
Experience and Expertise | High, with long-term employees | Variable, inconsistent due to higher turnover |
Quality Assurance | Strong, with ongoing skill development | Inconsistent, may struggle with complex tasks |
Security & Privacy | Compliance with standards like SOC 2, HIPAA, GDPR | Generally less secure due to varied access |
Cost Efficiency | Balanced, long-term quality focus | Lower upfront, but quality trade-offs possible |
Companies must adhere to privacy and security standards, using tools that meet regulations like SOC 2, HIPAA, or GDPR. It's critical to ensure providers follow these standards. Quality assurance processes, including mandatory reviews and feedback, are essential for maintaining high standards.
It's important to balance speed, accuracy, and cost for successful partnerships. Outsourcing data annotation can significantly reduce project time and costs. External partners can complete tasks up to 50% quicker and at a 40% cost reduction. The goal is to find a provider that meets your specific needs, ensuring accurate, secure, and efficient handling of logistics data through thorough evaluating experience and expertise.
The Role of AI in Logistics Data Annotation
In the logistics sector, AI integration is transformative, significantly impacting data annotation. Through machine learning integration, companies can achieve more precise and efficient annotations. These are essential for optimizing logistics operations. Automation in data annotation allows for quick and accurate handling of large data volumes, reducing errors and boosting throughput.
United States Cold Storage's AI-based automated appointment scheduling system is a prime example. It shows how AI can enhance supply chain operations. AI-driven solutions, like UPS Bot, also improve customer service efficiency, demonstrating AI's versatility in logistics.
AI Applications | Benefits |
---|---|
Delivery Route Optimization | Reduces Fuel Consumption and Predicts Maintenance Needs |
Inventory Management | Forecasts Stock Shortages and Minimizes Holding Costs |
Warehouse Management | Dynamically Adjusts Item Placement Based on Demand Patterns |
Cargo Theft Prevention | Enhances Security Through Predictive Insights (e.g., RiskGPT) |
AI and image annotation services significantly enhance logistics. They improve planning, resource allocation, and supply chain management. This keeps logistics firms competitive in a fast-changing market.
AI automation streamlines processes, reducing manual tasks and boosting efficiency. It uses advanced analytics and unifies data sources for precise insights. This drives smarter logistical decisions. Machine learning models help logistics firms stay agile and responsive to market demands, underlining AI's vital role in logistics and supply chain management.
The Future of Logistics and Data Annotation
Looking ahead, logistics will evolve significantly due to advancements in data annotation. The role of continuous improvement in this area is vital, given that 80% of data is unstructured. Data annotation services make this data usable for machine learning, unlocking its full value for businesses.
Image annotation is transforming logistics, improving inventory management and warehouse operations. It ensures real-time visibility and quality control in complex supply chains. Future trends include the integration of computer vision, augmented reality, and IoT, promising to further enhance logistics.
FAQ
What is logistics data annotation?
Logistics data annotation involves labeling logistics-related data, like text, images, and videos. This makes the data understandable for AI and ML models. It helps these models predict outcomes based on logistics operations.
Why is data annotation critical in logistics?
Data annotation is key in logistics because it trains AI systems with high-quality, accurately labeled data. This boosts AI model reliability, leading to smarter decisions and optimized workflows. It's vital for tasks like shipment tracking, route optimization, and inventory management.
What types of data can be annotated in logistics?
In logistics, various data types can be annotated. This includes images from warehouses, text from shipping documents, and videos from transit routes. Each type of annotation—image, text, and video—improves AI capabilities.
What are the benefits of logistics data annotation?
Logistics data annotation enhances AI model accuracy, streamlines operations, and improves decision-making. Annotated data allows AI to predict demand, optimize inventory, and manage resources efficiently.
What are some challenges in logistics data annotation?
Challenges include handling large data volumes, complex logistics scenarios, and ensuring data privacy and security. Overcoming these is vital for training accurate and reliable AI models.
How do advanced annotation tools and technologies aid the process?
Advanced tools, like machine-assisted labeling and crowd-sourcing, efficiently process large, diverse data sets. These tools streamline the process, ensuring accuracy and speed while reducing human error.
Why is collaboration with domain experts important in data annotation?
Working with domain experts ensures annotations accurately reflect logistics tasks' nuances. Their specialized knowledge enhances annotation quality, making AI models more accurate and effective in real-world scenarios.
How does AI improve logistics data annotation?
AI enhances logistics data annotation by using machine learning for smarter, more accurate annotations over time. Automation quickly and precisely processes large data volumes, reducing errors and costs.
What future trends are expected in logistics data annotation?
Future trends include increased AI and machine learning use, real-time data processing, and advanced annotation tools. These advancements will further boost efficiency and effectiveness in logistics.
How important is scalability when selecting an annotation service provider?
Scalability is vital when choosing an annotation service provider. Data needs can grow, and a provider capable of expanding ensures your logistics operations can leverage high-quality data as demands evolve.
What quality assurance techniques are used in logistics data annotation?
Techniques include rigorous data validation, consistency checks, and expert reviews. These ensure annotated data's reliability and accuracy, essential for robust AI models.
How does logistics data annotation enhance last-mile delivery solutions?
It improves last-mile delivery by boosting accuracy and customer satisfaction. Annotated data enables optimal route calculations and real-time updates, ensuring timely and precise deliveries.
Comments ()