Optimizing Maritime Video Annotation for AI Navigation Systems
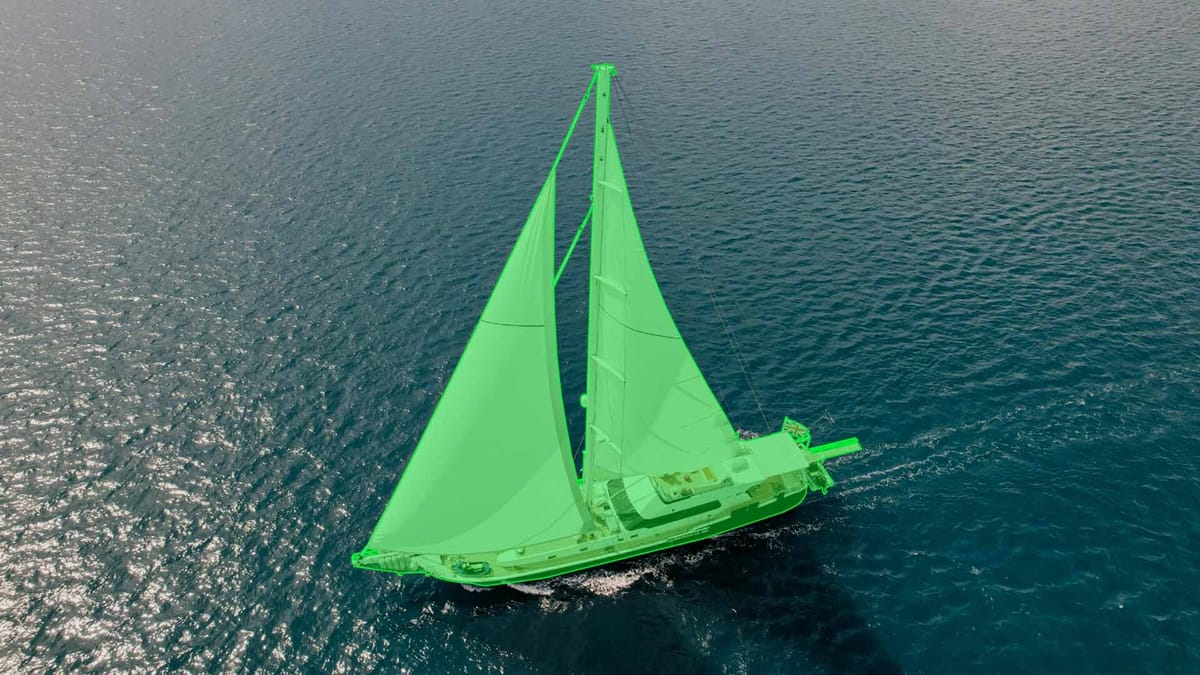
Maritime video annotation is key to boosting AI navigation systems. These systems are vital for maritime safety and efficiency. They rely on high-quality labeled data to accurately detect and monitor maritime environments. This leads to better decision-making and predictive analytics.
Key Takeaways
- High-quality maritime video annotation is essential for effective AI navigation systems.
- Improved detection and monitoring enhance safety and operational efficiency.
- AI training data must be meticulously labeled for accurate results.
- Trusted annotation services can prevent disruptions and support reliable maritime operations.
- Optimized annotation processes are critical for advancing autonomous maritime systems.
Maritime AI training data is a cornerstone for many breakthroughs. It supports sophisticated object detection and advanced predictive analytics. Accurate maritime video annotation is critical. It ensures AI navigation systems function optimally, addressing challenges like environmental variability and data privacy concerns.
As we move forward, optimizing these annotations will make maritime operations more reliable and autonomous. This highlights the tech's role in today's maritime activities. Using trusted outsource data annotation services can prevent supply chain disruptions. It ensures AI projects in shipping and logistics succeed.
Understanding Maritime Video Annotation
Maritime video annotation is a specialized process integral to the maritime industry. It involves the meticulous labeling of video footage specific to maritime environments to train AI models effectively. This process is critical for developing advanced systems capable of maritime object detection, activity recognition, and maritime scene understanding.
Definition and Importance
Maritime video annotation refers to the precise labeling of maritime video content. This practice is vital for creating data sets, which AI systems use to learn and improve their understanding of maritime environments. By annotating video footage, we enable AI to distinguish among different maritime objects. This enhances the automation of maritime surveillance and improves safety protocols.
Understanding scenes in a maritime context ensures AI can make accurate and reliable decisions. This leads to safer and more efficient maritime operations.
Key Applications in Maritime Industry
The applications of maritime video annotation are diverse and impactful:
- Maritime Surveillance: Enhancing security protocols by detecting unauthorized ships and potentially harmful activities in real-time.
- Vessel Tracking: Improving the monitoring and management of vessel movements within ports and harbors.
- Automated Port Logistics: Streamlining functions such as container handling, cargo inspection, and port entrance/exit monitoring to boost operational efficiency.
By leveraging maritime object detection and scene understanding, the maritime industry can achieve higher operational standards and safety measures.
Differences from Other Video Annotation Types
Maritime video annotation possesses unique challenges that set it apart from other types of video annotation. Unlike traditional annotation, which might deal with predictable environments, maritime annotations must account for variable lighting, weather conditions, and dynamic elements such as moving ships and buoys.
Operating under water and varying visibility conditions further complicates the process. These factors necessitate specialized approaches and technologies tailored for maritime scene understanding.
Aspect | Maritime Video Annotation | Other Video Annotation |
---|---|---|
Environment | Variable conditions (weather, lighting) | More controlled settings |
Elements | Moving objects like ships and buoys | Mostly stationary objects |
Complexity | More dynamic, requiring specialized approaches | Generally simpler and more predictable |
Applications | Maritime surveillance, vessel tracking, port logistics | General surveillance, retail analytics, autonomous driving |
Overall, maritime video annotation stands as a cornerstone for implementing advanced AI solutions in the maritime industry. It drives forward innovations and improvements in maritime object detection and scene understanding.
The Role of AI in Maritime Navigation
Artificial Intelligence (AI) is revolutionizing the maritime industry, boosting both safety and efficiency. By implementing maritime AI solutions, companies can lower operational risks and refine decision-making. This leads to a significant increase in navigation safety.
Enhancing Safety and Efficiency
AI's role in the maritime sector is critical, mainly in optimizing shipping routes. It analyzes GPS, weather forecasts, and maritime traffic data. This enables AI to reroute vessels, cutting down fuel consumption and CO2 emissions.
For example, autonomous ships equipped with AI can adjust their speed and course based on real-time sensor data. This effectively prevents collisions, ensuring a safer journey.
Real-Time Data Processing
Real-time maritime data processing is essential for managing complex maritime environments. Systems like Orca AI's SeaPod module, which you can learn more about here, use AI for navigation. They process live video feeds, providing immediate insights for navigating through congested waters and low-visibility conditions.
This innovation is vital for minimizing risks and improving vessel safety.
Predictive Analytics
Maritime predictive analytics are key for predictive maintenance and risk management. These are critical for maintaining operational continuity and reducing costs. By analyzing sensor data from ship engines, predictive analytics forecast maintenance needs, reducing downtime and expenses.
AI-based risk management systems also assess weather and traffic data to help vessels avoid hazardous conditions. AI analytics provide insights for optimal cargo loading, ensuring vessel stability and boosting operational productivity.
In summary, AI's role in maritime navigation is diverse, addressing critical areas from real-time data processing to predictive analytics. This technology integration drives the industry toward safer, more efficient operations. It also positions maritime companies at the forefront of innovation.
AI Applications in Maritime | Benefits |
---|---|
Fleet Management | Optimized scheduling and resource allocation |
Predictive Maintenance | Reduced downtime and maintenance costs |
Cargo Optimization | Improved loading efficiency and vessel stability |
Risk Management | Enhanced safety through weather and traffic data analysis |
Supply Chain Management | Increased operational efficiency from order management to logistics |
Techniques for Effective Video Annotation
In the maritime sector, video annotation relies on advanced techniques for precision and efficiency. These range from manual to automated methods. It's essential to blend these approaches for high accuracy and speed in labeling maritime video data.
Manual vs. Automated Annotation
Manual annotation requires human annotators to label objects in video frames, like ships or marine structures. This method ensures high accuracy but is time-consuming. On the other hand, automated maritime annotation uses AI algorithms to automatically detect and label objects. This speeds up the process and handles large datasets efficiently. Yet, automated methods need high-quality training data, which manual efforts can provide.
Quality Control Measures
Ensuring the reliability of video annotations is critical. This is achieved through rigorous annotation quality control measures. These include validation processes, consistency checks, and metrics from annotation platforms. Object interpolation is also used to track objects across frames, providing continuous and accurate maritime asset representation.
Tools and Software Used
Advanced video annotation tools and software are key for precise and efficient annotations. Platforms like Keymakr use technologies like RetinaNet and convolutional neural networks. These tools offer features for facial recognition, movement interpretation, and semantic segmentation. By leveraging these technologies, annotators can improve AI training data accuracy, leading to enhanced AI navigation systems in the maritime industry.
For instance, Keymakr services demonstrate the effectiveness of combining human expertise with advanced software. This approach accelerates maritime annotation, controls costs, and ensures precision. It provides a solid base for developing robust AI models for maritime applications.
Best Practices for Maritime Video Annotation
Following best practices in maritime video annotation is essential for the accuracy and reliability of annotated data. Establishing robust protocols significantly enhances the efficiency and quality of AI-driven autonomous navigation systems. Let's explore the fundamental principles that tech professionals should adhere to.
Standardizing Annotation Protocols
Standardizing maritime data annotation protocols is vital for maintaining uniformity across different tasks. Standard protocols ensure consistent annotation of each image or video frame, aiding in the seamless training of AI models. To achieve this:
- Develop detailed guidelines that cover all aspects of annotation, from labeling objects to categorizing events.
- Utilize standardized tools and software like NICAMS, VARS, and VIDLIB to ensure consistent data annotation practices.
- Regularly review and update protocols based on the latest research and technological advancements.
For more insights on effective maritime data annotation, click here.
Ensuring Data Consistency
Maintaining data consistency is a significant challenge in AI model training. Ensuring data consistency in AI training is critical for building accurate and reliable AI systems for maritime navigation. Here are some key measures:
- Implement automated quality control measures to catch any inconsistencies in the annotated data.
- Use cross-validation techniques to ensure the data set remains consistent across different training batches.
- Maintain a centralized database for storing annotated data, making it easier to track and manage the annotations.
Training Annotators for Quality Output
Thorough training of annotators is essential for producing high-quality data annotations. Annotators need to be skilled in handling complex maritime data and following standard protocols effectively. Essential training elements include:
- Comprehensive training programs that cover all aspects of maritime annotation, including sensor technologies and environmental variability.
- Regular workshops and refresher courses to keep annotators up-to-date with the latest tools and methodologies.
- Performance assessments and feedback mechanisms to ensure continuous improvement in annotation quality.
Annotation Type | Purpose | Tools Used |
---|---|---|
Bounding Box | Object tracking in videos | NICAMS, VIDLIB |
Polygon Annotation | Details on irregularly shaped objects | CATAMI, SQUIDLE |
Semantic Segmentation | Object class differentiation | Coral Point Count |
Instance Segmentation | Unique object identification | CoralNet, BIIGLE |
Challenges in Maritime Video Annotation
Maritime video annotation faces unique challenges due to the maritime environment's complexities. Annotated video data is vital for training AI systems. These systems aim to improve maritime operations' efficiency, safety, and reliability. Yet, several hurdles make this task extremely demanding.
Environmental Variability
The maritime environment's unpredictability significantly affects data quality. Lighting, weather, and sea state changes can drastically alter visual data from surveillance systems. A Keymakr's report highlights the need to mitigate the environmental impact on data quality for AI model robustness. Annotators must adapt to these variables, ensuring the data reflects real-world scenarios.
Equipment Limitations
Equipment limitations pose another significant challenge in maritime video annotation. UAVs, used for surveillance, face issues with data quality due to camera resolution and stability. Tools like BIIGLE 2.0 help overcome these limitations. It supports video annotations across multiple frames, adapting to object movement and shape changes.
Data Privacy and Security Concerns
Data privacy and security are critical in the maritime sector. The industry handles sensitive information, making it vulnerable to cyber threats. Implementing robust security measures and protocols is essential. Policies must govern data access and use, adhering to international standards.
Addressing maritime annotation challenges requires a holistic approach. It must consider environmental variability, equipment limitations, and data privacy. By using advanced tools and following industry best practices, the maritime sector can improve AI system capabilities.
Integrating Video Annotation with AI Models
Integrating video annotation with AI models in the maritime sector requires careful dataset preparation and management. This ensures the data used is relevant and of high quality. A robust maritime dataset management process is essential for developing precise and efficient AI solutions.
Dataset Preparation and Management
Effective maritime dataset management is key for integration. Properly organized datasets improve the quality and accuracy of training data. This ensures AI models learn from the best sources. The process involves:
- Curating diverse and representative data samples
- Implementing data annotation protocols
- Ensuring data consistency and quality
Training AI Algorithms
Training maritime AI models focuses on creating algorithms that accurately interpret and process annotated data. Techniques like object interpolation algorithms help streamline the process by tracking labeled objects across frames. Common methods include:
- Bounding box annotation for cost-effective labeling
- Instance segmentation for detailed object class identification
- Polygon annotation for precise object shape definition
Automation tools like V7, Dataloop, Keylabs, and Amazon SageMaker Ground Truth are essential for improving annotation efficiency and precision.
Continuous Learning and Improvement
The journey doesn't end with initial training; continuous AI improvement is necessary. This involves:
- Regularly updating datasets with new annotations
- Refining models based on performance metrics such as precision (P), recall (R), and F1 score
- Utilizing feedback loops for iterative enhancements
By keeping models updated with the latest data and advancements, we ensure they remain robust and accurate in real-world applications.
Annotation Type | Usage | Tools |
---|---|---|
Bounding Box | Cost-effective labeling | Dataloop, CVAT |
Polygon Annotation | Precise object shapes | Ruttl, V7 |
Instance Segmentation | Object class identification | Hasty.ai, Amazon SageMaker Ground Truth |
The integration of video annotation with AI models is a continuous cycle. It involves dataset preparation, training maritime AI models, and iterative improvement. This cycle ensures optimal performance in maritime environments.
Evaluating Annotation Quality
Ensuring the efficacy of maritime video annotation is critical for developing robust AI models. Quality assessment in video annotation relies on precise metrics, feedback mechanisms, and iterative improvement. These elements ensure annotations are accurate, consistent, and of high quality.
Metrics and Assessment Techniques
Several metrics are used to evaluate video annotation precision. Cohen's kappa and Fleiss' kappa measure agreement between annotators. Krippendorf's alpha is useful for datasets with incomplete data. The F1 score combines precision and recall for a detailed assessment of model performance.
Inter-annotator agreement (IAA) is key for maintaining consistency, even with large datasets. For example, analyzing 273 hours of video from 43 ROV dives requires consistent annotation.
Feedback Mechanisms
Effective feedback in maritime AI training is vital for maintaining and improving annotation quality. Regular feedback helps identify common mistakes and areas for improvement. This approach is reinforced by platforms like Labellerr, ensuring seamless feedback integration.
Automated and manual quality checks further enhance this process. They ensure high-quality deliverables are maintained.
Iterative Improvement Processes
Continuous improvement is essential for refining annotation quality. An iterative improvement process updates and refines annotation guidelines. This addresses previous mistakes and adapts to new AI technology challenges.
Establishing golden standards and aligning with best practices ensures data consistency and quality. Focusing on iterative refinement helps AI models achieve superior performance and more reliable predictions.
Future Trends in Maritime Video Annotation
The maritime industry is on the cusp of a technological revolution, with maritime video annotation at its forefront. Ongoing AI advancements are driving the growth of automated annotation, promising significant boosts in efficiency and accuracy. This evolution is set to transform maritime operations.
AI Advancements and Automation
AI is ushering in a new era of automation in maritime video annotation. Improved algorithms and machine learning are enabling the automatic identification and tagging of maritime elements. While current systems excel in post-processing stable platforms and images, the horizon for real-time annotation is vast. This will reduce human error and speed up analysis and decision-making, leading to safer and more efficient navigation.
Enhanced Sensor Technologies
The integration of advanced sensor technologies in shipping is set to enhance data quality and depth. By combining data from cameras, LiDAR, and radar, maritime video annotation can capture a more detailed picture of the maritime environment. This approach allows AI systems to better predict and respond to dynamic maritime scenarios, reducing accidents and operational errors.
Collaboration and Data Sharing Platforms
The development of collaborative platforms for maritime data sharing is an exciting trend. By sharing annotated datasets, industry stakeholders can drive innovation and standardize annotation protocols. This collective effort not only speeds up AI model development but also ensures consistency and reliability across the industry. It promises a future where maritime operations are safer, more efficient, and more intelligent.
These trends highlight the transformative power of video annotation in the maritime sector. Embracing AI advancements, enhanced sensor technologies, and collaborative data sharing sets a course for a safer and more efficient maritime future.
Case Studies in Maritime Video Annotation
Exploring various case studies in maritime annotation offers valuable insights into maritime operational efficiency. Real-world implementations show how detailed and accurate video annotation profoundly impacts maritime operations.
Successful Implementations
Skylight's innovative annotation tool is a prime example of success. It has significantly streamlined annotating Automatic Identification System (AIS) data. Over 2 million AIS positions were annotated in just a few months with a minimal number of annotators. This efficiency advances AI's understanding of vessel behavior and sets a new benchmark for the maritime industry. More details on this breakthrough can be found here.
The FathomNet Database, launched in 2021, has collected over 100,000 images and nearly 300,000 localizations from a community of over 600 individuals. This community effort has resulted in an accessible data pool that significantly aids in training and developing AI models for maritime applications.
Lessons Learned from Failures
Despite advanced tools and substantial datasets, the journey to effective maritime annotation has faced challenges. Varying environmental conditions and equipment limitations often introduce inaccuracies in annotations. Learning from these failures has been key to refining strategies and approaches in video annotation. A notable lesson is the importance of consistent quality control measures to ensure data reliability and usability.
The pilot course "Computer Vision across Oceanography" highlights educational gaps in this field. Designed to equip students with best practices in marine imaging techniques, this initiative emphasizes the need for continual learning and adaptation in the evolving landscape of maritime video annotation.
Impact on Operational Efficiency
The cumulative knowledge from successful cases and lessons learned from failures has profound implications for maritime operational efficiency. By leveraging detailed annotations and extensive datasets, maritime operators can significantly enhance vessel tracking, predictive maintenance, and security measures. The FathomNet Model Zoo, for instance, hosts a variety of machine learning models trained on this annotated data, enabling automated and precise monitoring of maritime activities.
Continuous refinement of annotation techniques and integration with AI systems facilitates improved operational efficiency. This sets a foundation for advanced maritime navigation and safety protocols.
FAQ
What is maritime video annotation and why is it important?
Maritime video annotation labels video footage specific to maritime environments. It trains AI models effectively. This is key for object detection, activity recognition, and scene understanding in maritime contexts. It boosts safety and operational efficiency in the maritime industry.
How does AI improve maritime navigation systems?
AI transforms maritime navigation by processing data in real-time, using predictive analytics, and making immediate decisions. It navigates complex routes, predicts hazards, and optimizes routes automatically. This enhances safety and efficiency.
What are the key applications of maritime video annotation?
Maritime video annotation is vital for surveillance, vessel tracking, and automated port logistics. It improves security and efficiency by providing high-quality data for AI systems.
How does maritime video annotation differ from other types of video annotation?
Maritime video annotation faces unique challenges like variable lighting, weather, and moving objects. It requires specialized techniques and tools for accurate labeling.
What techniques are used for effective maritime video annotation?
Effective annotation combines manual and automated methods. Manual annotation ensures accuracy, while automated methods speed up processing. Quality control is critical for reliable annotations.
What are the best practices for maritime video annotation?
Best practices include standardizing protocols for consistency and training annotators to high standards. Ensuring data consistency is also key for accurate AI models.
What are the main challenges in maritime video annotation?
Challenges include environmental variability, equipment limitations, and data privacy and security concerns. These factors affect data quality and accuracy.
How is video annotation integrated with AI models in the maritime sector?
Integration involves preparing and managing datasets, training AI algorithms, and implementing continuous learning. This ensures AI adapts to new data and conditions.
How is annotation quality evaluated in maritime video annotation?
Quality evaluation uses robust metrics and feedback mechanisms. It involves iterative improvement to meet standards and enhance over time.
What future trends are expected in maritime video annotation?
Future trends include AI advancements, enhanced sensor technologies, and increased collaboration. These will drive innovation and standardization in the industry.
Can you provide examples of successful maritime video annotation implementations?
Case studies show successful implementations that enhance efficiency and security. Learning from successes and failures refines strategies and improves AI systems in the maritime industry.
Comments ()