Outsourcing Data Annotation Services
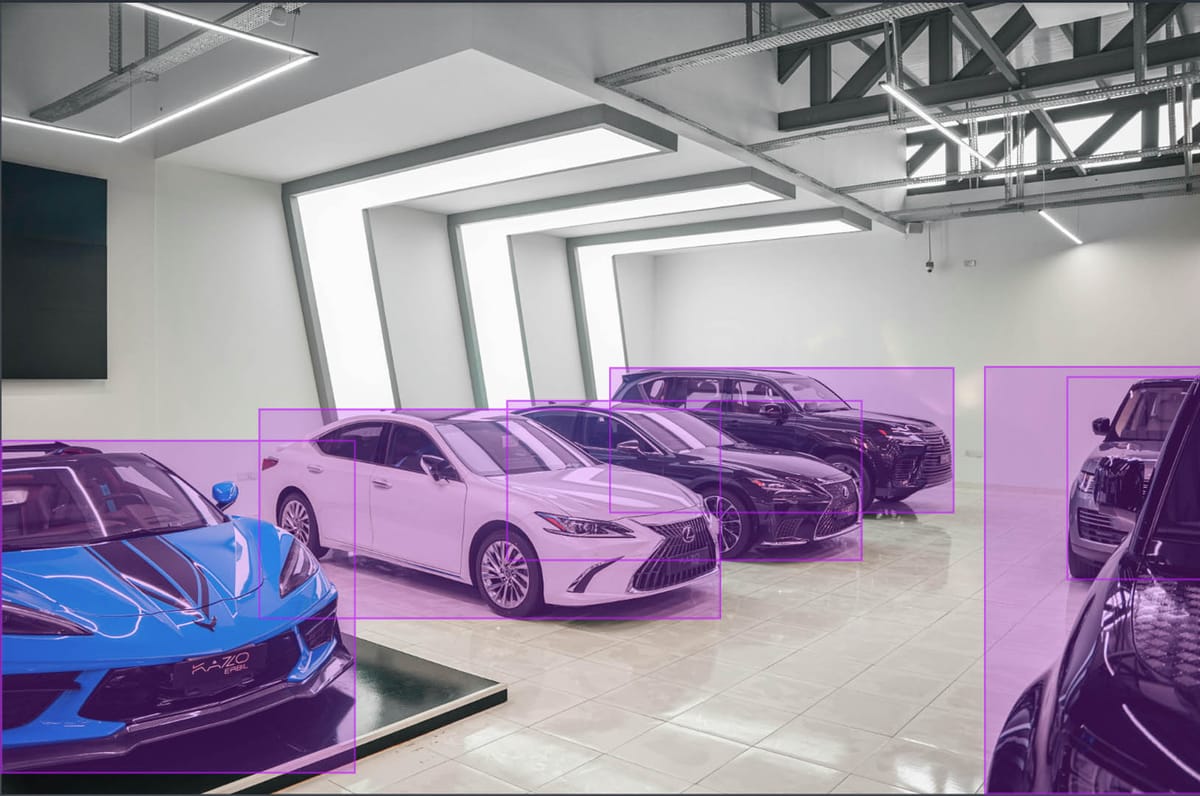
Outsourcing data annotation services is a strategic choice for companies looking to enhance their AI models with precision and quality. By partnering with a reliable data annotation provider, businesses can access a skilled workforce that specializes in image annotation, data labeling, and natural language processing (NLP). This section will explore the benefits and key considerations of outsourcing data annotation services.
Key Takeaways:
- Data annotation services contribute to enhancing AI models with precision and quality.
- Partnering with a reliable data annotation provider gives access to skilled specialists.
- Outsourcing data annotation services include image annotation, data labeling, and natural language processing (NLP).
- Exploring the benefits and key considerations of outsourcing data annotation services is crucial.
- Proper data annotation leads to the successful implementation of AI solutions.
The Importance of Data Annotation in Machine Learning
Data annotation is an essential step in training machine learning models, particularly those that rely on image-based data. With the growing popularity of applications such as image recognition and computer vision, accurately labeled images play a critical role in ensuring the success and effectiveness of these AI systems. Through the process of image annotation, relevant tags and annotations are added to each image, providing a foundation for the model to learn and make accurate predictions.
Image annotation involves various techniques such as bounding boxes, semantic segmentation, keypoint annotation, and polygon annotation. These techniques help in identifying and labeling objects, regions of interest, and other important details within an image. For example, in an image recognition system, data annotators would label different objects present in the image, enabling the model to recognize and distinguish between them accurately.
The accuracy and quality of the annotated data are crucial for training machine learning models. High-quality training data ensures that models learn from representative, diverse, and unbiased examples, which leads to better performance in real-world scenarios. Incorrect or incomplete annotations can result in models that are inaccurate, biased, or unable to understand the complexities of real-world data.
Outsourcing data annotation services can help businesses ensure the high-quality training data needed for successful AI applications. By partnering with experienced data annotation providers, companies can leverage the expertise of skilled data annotators who specialize in image annotation and understand the nuances of different annotation techniques. These professionals ensure that each image is annotated accurately and consistently, resulting in robust and reliable training datasets.
Furthermore, outsourcing data annotation offers scalability and cost-efficiency. Instead of investing in setting up an internal annotation team, which can be time-consuming and expensive, businesses can tap into the resources of a dedicated data annotation provider. These providers have the infrastructure, tools, and processes in place to handle large volumes of data annotation effectively. This allows companies to scale their annotation efforts based on project requirements without compromising on quality or delivery timelines.
Overall, data annotation is a crucial step in machine learning, particularly in image-based applications. Outsourcing data annotation services provides businesses with access to skilled annotators, high-quality training data, and cost-effective solutions. By prioritizing accurate and comprehensive data annotation, companies can train robust AI models capable of accurate image recognition and understanding.
Benefits of Outsourcing Data Annotation Services
Outsourcing data annotation services provides numerous benefits for businesses, ensuring efficiency, expertise, and high-quality training data. By leveraging image annotation outsourcing, companies can access a pool of skilled data annotators without the need for time-consuming hiring and training processes. This scalability enables projects to be completed efficiently while maintaining the utmost precision and accuracy in the annotated datasets.
When outsourcing data annotation services, companies also gain access to the specialized expertise of data annotators who possess intricate domain knowledge and extensive experience in handling various types of data. This expertise ensures accurate and reliable annotations, leading to the enhanced effectiveness and efficiency of AI training models.
- Scalability: Outsourcing data annotation allows businesses to quickly assemble skilled data annotators, ensuring project success and efficient completion.
- Cost-efficiency: By outsourcing, companies can avoid the costs associated with hiring and training internal staff, optimizing their budget allocation.
- High-quality training data: Skilled data annotators provide accurate and reliable annotations to improve the precision and effectiveness of AI training models.
Outsourcing data annotation services is a strategic choice for businesses looking to streamline their AI model development processes. By partnering with a reliable provider, companies can scale their annotation resources, achieve cost-efficiency, and obtain high-quality training data for their AI projects.
Comparison of In-house Annotation vs. Outsourcing
Factors | In-house Annotation | Outsourcing Annotation |
---|---|---|
Expertise | Limited to internal resources | Access to specialized data annotators with extensive domain knowledge |
Scalability | Dependent on internal hiring and training processes | Quickly assemble skilled annotators based on project requirements |
Cost-efficiency | Higher costs associated with hiring and training | Budget optimization by avoiding internal staffing expenses |
Training Data Quality | Potential inconsistencies and biases | Consistent and reliable annotations for improved model performance |
Project Timelines | Dependent on internal resource availability | Efficient completion within defined timelines |
How to Outsource Data Annotation
When outsourcing data annotation services, selecting the right vendor is crucial for the success of your AI projects. Businesses should consider several factors to ensure the highest quality and most effective annotation process.
Expertise in Data Annotation
One of the key factors to consider is the vendor's expertise in data annotation. Look for a vendor with a proven track record in handling diverse datasets and annotations. Their experience in image annotation, data labeling, and natural language processing (NLP) will determine the accuracy and reliability of the annotations.
Quality Control and Monitoring
Quality control is essential to ensure that the annotated data meets your desired standards. A reputable data annotation vendor will have robust quality control processes in place. They should provide detailed guidelines to annotators, implement rigorous checks, and constantly monitor the quality of the annotations to maintain consistency and accuracy.
Security Certifications
Protecting sensitive data is paramount. Look for a data annotation vendor with appropriate security certifications to handle confidential information. Certifications such as ISO 27001 demonstrate their commitment to maintaining data security and confidentiality throughout the annotation process.
Unbiased Representation
Ensuring unbiased representation in annotations is critical for enhancing the fairness and inclusivity of your AI models. Select a vendor that prioritizes diversity in their workforce and has policies in place to mitigate bias during the annotation process. This will help avoid potential biases that may arise due to cultural, gender, or racial factors in the annotated data.
Scaling Annotation Resources
Consider the vendor's ability to scale annotation resources based on your project needs. They should have sufficient manpower to handle large volumes of data and the flexibility to accommodate tight deadlines. A reliable vendor will have well-defined processes and the capability to adapt to changing requirements seamlessly.
Structured Approach to Outsourcing
By following a structured approach to outsourcing data annotation, businesses can streamline the development process and achieve their AI goals effectively. Establish clear communication channels, define project requirements in detail, and maintain regular feedback loops with the vendor to ensure alignment and address any concerns promptly.
By carefully selecting a data annotation vendor that possesses the necessary expertise, maintains quality control, upholds security certifications, promotes unbiased representation, and can scale annotation resources, businesses can effectively outsource their data annotation needs and enhance the accuracy of their AI models.
Conclusion
Outsourcing data annotation services provides a practical and cost-effective solution for companies and organizations in need of large volumes of annotated images. By partnering with a reliable and experienced data annotation provider, businesses gain access to high-quality training data, enabling them to enhance the accuracy of their AI models and streamline the development process.
Data annotation outsourcing offers numerous benefits, including the ability to assemble a skilled team of data annotators without the time and effort required for internal hiring and training. This scalability ensures projects are completed efficiently while maintaining the highest standards of quality. Additionally, partnering with specialized data annotators who possess domain knowledge and expertise in dealing with various data types guarantees accurate and reliable annotations, ultimately improving the speed and efficiency of AI training.
With the right approach to data annotation outsourcing, companies can unlock the full potential of their AI projects. By harnessing the capabilities of a reliable data annotation provider, businesses are able to access high-quality training data, enhance their AI model development, and achieve their goals in a streamlined and cost-effective manner.
FAQ
What is data annotation?
Data annotation is the process of labeling or tagging data to provide a framework for machine learning models to learn and make accurate predictions. In the context of image annotation, it involves labeling images with relevant tags and annotations.
Why is data annotation important in machine learning?
Data annotation is crucial in machine learning as it helps train AI models that rely on image-based data. By annotating images with relevant tags and annotations, it enables the model to learn patterns and make accurate predictions.
What are the benefits of outsourcing data annotation services?
Outsourcing data annotation services offers several benefits, including quick access to a team of skilled data annotators, scalability to efficiently complete projects, access to specialized domain knowledge, and enhanced accuracy and efficiency in AI training.
What should businesses consider when selecting a data annotation vendor?
When selecting a data annotation vendor, businesses should consider factors such as the vendor's expertise in data annotation, security certifications for handling sensitive data, commitment to diversity and unbiased representation, and the ability to scale annotation resources based on project needs. Quality control and monitoring are also essential considerations.
Is outsourcing data annotation a cost-effective solution?
Yes, outsourcing data annotation services can be a cost-effective solution, especially for companies and organizations requiring large volumes of annotated images. By partnering with a reliable and experienced data annotation provider, businesses can access high-quality training data and streamline the development process of their AI models.
Comments ()