Predictive Maintenance Revolutionized by AI Models
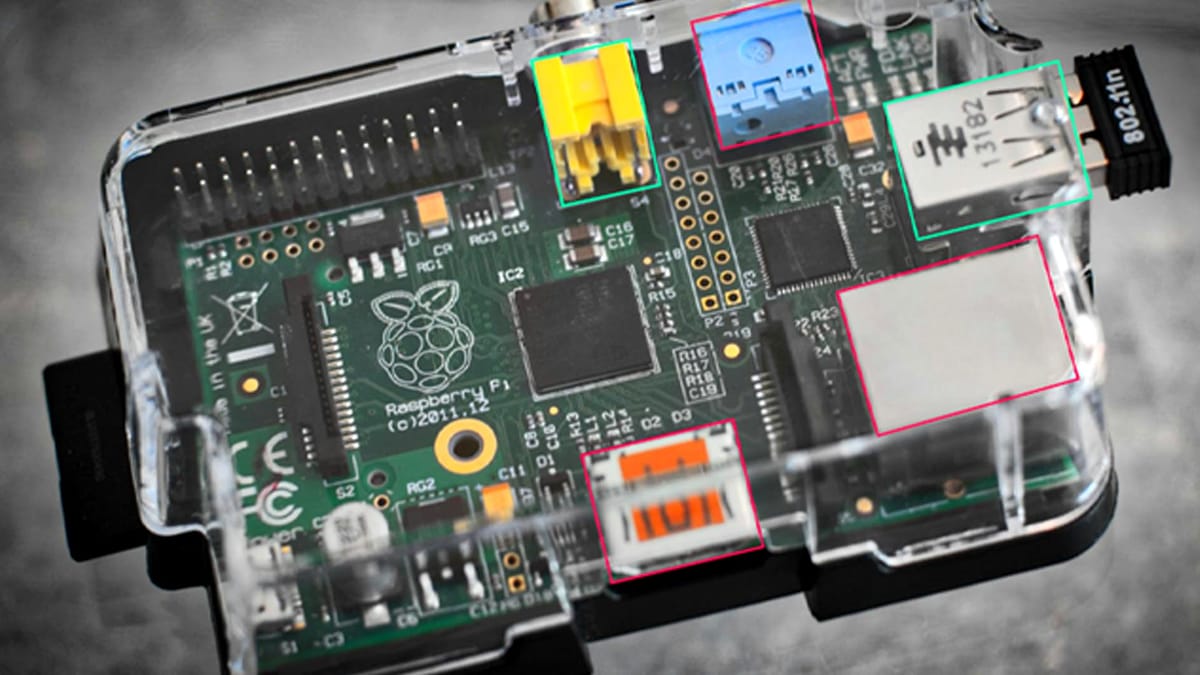
Imagine reducing breakdowns by a huge 70% and cutting maintenance costs by 25%. At the same time, productivity jumps by 25%. These figures are real benefits from using AI model predictive maintenance. Now, machine learning and AI are changing how we manage and care for equipment.
Advanced analytics forecasting lets companies find possible breakdowns before they happen. This big leap in predictive analytics means businesses can prevent problems, not just fix them. Now, maintenance is all about stopping issues before they start, which boosts both productivity and profits.
Key Takeaways
- AI model predictive maintenance ushers in a new paradigm in enterprise asset management.
- Machine learning for asset management enables businesses to forecast and prevent equipment issues proactively.
- AI-powered maintenance forecasting is a significant contributor to minimizing operational downtime.
- Advanced analytics enables a more efficient and cost-effective approach to asset maintenance and longevity.
- The adoption of predictive analytics leads to improved operational efficiency and elevated ROI.
Unveiling AI Model Predictive Maintenance
The leap in predictive analytics for equipment is changing how we manage assets. It turns unexpected failures into alerts we can act on. With AI-enabled condition monitoring, companies gain from real-time data. This boosts how reliable operations are and lowers the risk of equipment shutting down.
AI predictive maintenance uses smart algorithms. It looks at both sensor data and past maintenance work. This helps understand how equipment is doing over time. It leads to data-driven maintenance optimization.
Let's look at how manufacturing uses predictive maintenance. We'll compare it to the old way of doing things:
Aspect | Traditional Maintenance | AI-Powered Predictive Maintenance |
---|---|---|
Data Utilization | Periodic reviews, often outdated | Continuous, real-time data analysis |
Cost Implications | High due to unplanned downtimes | Reduced, with maintenance preemptively managed |
Equipment Lifespan | Decreases with reactive maintenance | Increases due to proactive care |
Operational Safety | Compromised by unexpected failures | Enhanced by predictive insights |
Moving to proactive maintenance saves money and improves how industries like manufacturing and aerospace operate. It also makes these places safer to work. The predictive analytics for equipment in AI-enabled condition monitoring systems are making maintenance smarter and more reliable.
Imagine all companies following data-driven maintenance optimization as a norm. That's the future AI predictive maintenance is creating for industries everywhere.
The Proficiency of Machine Learning Algorithms in Maintenance
Industries like manufacturing and healthcare have greatly improved with machine learning for predictive maintenance. This approach uses large datasets from operation logs and sensor data. It helps predict failures before they happen.
Analyzing Patterns: The Heart of Prediction
Machine learning is key in spotting patterns and issues that could lead to equipment failure. Deep learning for fault prediction looks into complex data, finding links not seen with old methods.
Training Models with Historical Data
Using old maintenance records and data, machine learning models become better at predicting breakdowns. This makes predictive maintenance better. It cuts downtime significantly.
Enhancing Early Fault Detection Capabilities
Improved predictive models boost early fault detection. This allows for quick fixes, avoiding big repair costs and shutdowns. This switch to proactive maintenance saves resources and boosts productivity.
Predictive maintenance cuts equipment failures by 70%. It also increases productivity and efficiency by 25%. This reduces maintenance costs, giving companies an advantage in the market.
Feature | Impact | Technique Used |
---|---|---|
Early Fault Detection | Reduces downtime by preempting equipment failures | Neural Networks, Support Vector Machines |
Operational Efficiency | Enhances performance and lifespan of machinery | Deep Learning, Reinforcement Learning |
Cost Management | Lowers maintenance expenses by up to 25% | Genetic Algorithms, Particle Swarm Optimization |
With more industries using machine learning and deep learning, maintenance is transforming. This brings better control over operations, leading to great savings and efficiency.
Farewell to One-Size-Fits-All Maintenance Schedules
Old maintenance schedules, common in the past, are now replaced by smarter, customized plans. This change is due to AI and machine learning. With condition-based maintenance, tools now signal when they need care, avoiding outdated, costly ways.
AI-enabled maintenance optimization uses real-time sensor data. It predicts and meets maintenance needs just in time. This approach cuts downtime and saves money, letting companies focus on improving their operations.
- Proactively addresses maintenance needs
- Reduces wasteful expenditures
- Enhances equipment lifespan
- Decreases unplanned downtime
Switching to flexible, need-based maintenance lets businesses stay ahead in today's fast market. Real-time data and AI make operations efficient and sustainable, marking a significant shift.
"Leveraging AI-enabled maintenance optimization is not just about technology adoption, it's about transforming business processes for operational resilience and sustainability."
Traditional Maintenance | AI-Enabled Condition-Based Maintenance |
---|---|
Scheduled based on time or usage | Driven by real-time data and AI analysis |
Often leads to unnecessary maintenance | Optimizes maintenance tasks, reducing unnecessary actions |
May not address sudden failures | Predictive maintenance can forecast and mitigate sudden issues |
Resource-intensive and often inefficient | Improves resource allocation and utilization |
With AI, the way we maintain operations is transforming. Your move to condition-based maintenance will make your processes smarter. It's a crucial change for a more planned and cost-saving operation.
Data-Driven Maintenance: Cost Reduction and Agility
In our fast-moving world, using data-driven maintenance practices is a big change. We've moved from checking on things regularly to proactively watching over asset health with real-time data monitoring. This shift doesn't just keep things running smoothly; it completely changes the game.
From Scheduled Checks to Proactive Monitoring
Before, managing assets was about reacting after things went wrong, guided by a schedule that could miss problems leading to downtime. Now, picture a system where IoT sensors gather data all the time. This data helps advanced AI models spot problems before they happen. This way of monitoring is a huge improvement, making maintenance something we can plan for instead of just react to.
Optimizing Resources for Maximum Efficiency
Predictive maintenance uses data to make smart decisions about where and when to use resources. This approach boosts how well operations run and cuts down on maintenance costs. It makes sure we act based on what the data and alerts tell us, not just routine checks. This is much more efficient.
Benefit | Statistical Impact |
---|---|
Reduction in unplanned downtime | 30-50% |
Extension of asset life | 20-40% |
Cost savings over traditional maintenance | Significant |
Early identification of failures | Up to months in advance |
By mixing real-time data monitoring with AI and ML algorithms, we cut down on unexpected repair costs. We also improve how reliable and available our assets are. With proactive asset health monitoring, businesses can do more than keep up; they can lead in today's market.
Improving Reliability with Predictive Analytics
Predictive analytics is reshaping how businesses handle maintenance, making operations more reliable and cost-effective. By using AI, companies can now spot problems before they worsen. This improves availability and allows for smarter maintenance planning.
With AI-driven predictive maintenance, industries adopt a proactive approach to upkeep. This is crucial in sectors like manufacturing and vehicle rentals, where downtime means big losses. Teams analyze real-time and historical data to prevent equipment failures accurately.
- Predictive analytics schedule repairs without disrupting work, cutting unwanted downtime.
- Teams make faster, better decisions with AI-generated insights.
- Statistical insights help focus maintenance, avoiding unnecessary work.
Predictive analytics do more than stop downtime. They also boost safety by spotting hazards early, protecting people and machines. This strategy extends equipment life and reduces costs. Companies can arrange maintenance during downtimes, keeping operations smooth.
Deloitte Analytics Institute shares a compelling fact: predictive maintenance boosts productivity by 25%, cuts breakdowns by 70%, and lowers maintenance expenses by 25%. These numbers show how powerful AI-enhanced maintenance is across industries.
Optimizing Asset Performance with AI Insights
Industries today aim to optimize asset performance by integrating AI insights. By analyzing sensor data, businesses can adjust their operations proactively. This improves energy efficiency and cuts costs, offering a sustainable way to boost performance.
Boosting Efficiency Through Data Intelligence
Using data intelligence, companies can make their operations smoother and more energy-efficient. Smart AI analytics help in making better decisions for machinery operations. This enhances asset use and extends equipment life, optimizing cost and performance.
Realizing the Full Potential of Assets
Proactive asset performance management takes a full-scale approach. It uses AI for predictive maintenance and real-time adjustments. This boosts reliability, reduces upkeep costs, and prepares for growth.
The manufacturing world is changing fast. Adapting and innovating are key. AI not only meets current needs but also helps in future asset management. Using tech smartly gives industries a competitive edge.
Precision in Resource Allocation Through AI
In today's fast-paced industrial landscape, precision in maintenance resource allocation is key. It uses AI-models for resource prioritization. AI helps businesses become more efficient and manage their expenses better.
AI and machine learning are changing how we do maintenance. They turn old methods into smart, data-driven strategies. By looking at data from sensors and logs, AI predicts when equipment might fail. This helps keep things running smoothly and saves money by preventing unnecessary spending.
- Predictive analytics move from general schedules to monitoring based on actual conditions.
- Resources are strategically used where they're needed most.
- This boosts reliability and cuts down on sudden downtime.
Using AI-models for resource prioritization makes machinery last longer. It also makes sure maintenance fits with production needs. This way, industries run without hitches. It shows how powerful AI can be in making maintenance proactive.
Feature | Impact |
---|---|
AI-Driven Predictive Maintenance | Up to 25% reduction in maintenance costs and 35% decrease in downtime |
Condition-Based Monitoring | Immediate attention to issues saves time and resources |
Resource Prioritization | Makes sure important resources are used wisely |
The smart use of AI-models for resource prioritization cuts costs and sets up businesses for future success. By using AI, industries are not just keeping up; they're getting better. They put intelligence at the heart of what they do.
The Power of Data-Driven Decision-Making
In today's fast-changing business world, data-driven decisions are key. Advanced AI helps manage assets smartly. It lets organizations make wise choices about their resources. This new approach improves maintenance plans through top-notch data analytics, boosting work effectiveness.
Leveraging Predictive Intelligence for Strategic Planning
AI tools fit perfectly into asset management, improving investment decisions. They fine-tune maintenance and operations based on real performance data. AI predicts when equipment might fail. So, organizations can fix issues before they happen, saving resources and extending asset life.
Mitigating Risks with Informed Decisions
AI-driven risk strategies significantly benefit a business's financial and operational aspects. Predictive intelligence helps companies spot risks early. They then take steps to shield crucial assets and maintain smooth operations. This method cuts downtime and the costs of fixing problems after they arise.
Using these advanced tools transforms business operations. It makes asset management more reliable and sets new industry standards. With data guiding decisions, AI and machine learning pave the way for ongoing growth and success.
AI Technologies: The Backbone of Predictive Maintenance
Using AI technologies in predictive maintenance transforms how industries operate. These tools improve the timing and accuracy of maintenance work. They analyze data from sensors and usage stats. This helps predict failures before they happen.
AI cuts down on costs and helps equipment last longer. For example, some industries see up to a 12% cost reduction. They also report a 20% boost in how long equipment lasts. Plus, using resources well gets a 15% improvement.
AI also makes workplaces safer. It leads to a 25% drop in accidents from equipment failures. This proactive step saves money and keeps workers safe.
Key Metrics | Impact |
---|---|
Average Cost Reduction | 12% decrease |
Equipment Lifespan Extension | 20% increase |
Resource Efficiency Improvement | 15% better allocation |
Safety Enhancements | 25% reduction in accidents |
Success Rate in Failure Prediction | Over 85% |
Companies invest an average of 20% of their maintenance budget initially. Another 15% goes into training staff. This investment enables the use of AI technologies in predictive maintenance. It leads to machine learning models that get better over time. They have an over 85% success rate in catching equipment failures early. Remember, high-quality data is key for accurate models.
For more info on AI's role in future maintenance, check out this in-depth article.
The benefits of data-driven analytical tools in maintenance are huge. They give businesses a leg up in being reliable and excellent. With AI, predictive maintenance is changing the game.
The Transformational Impact of Deep Learning on Fault Prediction
Deep learning is changing how we predict faults in machines. It's making it easier to keep an eye on machine health. By using advanced deep learning, we can better understand complex data from machines. This includes identifying different fault types like Heat dissipation failure, Overstrain Failure, and Power Failure.
Deciphering Complex Data for Predictive Insights
Our dataset doesn't have missing values and shows normal distribution in some areas, making it perfect for neural networks. These networks are key in monitoring the health of assets. They sort through data, even when it's unbalanced or has missing pieces.
Techniques like One-Hot encoding and recommended data splits help. They ensure deep learning models work as they should.
Transforming Anomaly Detection with Neural Networks
Deep learning can find the best way to structure a network by exploring and adjusting parameters. It's great for detecting "Random Failure" events, despite their rarity. Dropout and Batch Normalization help the model avoid overfitting.
Using 'softmax' activation and tackling high class imbalance sharpens fault classification. It changes how we plan maintenance and keep machines running smoothly.
FAQ
What is AI model predictive maintenance?
AI model predictive maintenance uses AI and machine learning to foretell when to maintain equipment. It's a proactive method. It uses real-time sensor data and past maintenance records. The goal is to predict failures, reduce downtime, and make assets last longer.
How does machine learning enhance asset management?
Machine learning improves asset management by analyzing data to find patterns indicating possible equipment failures. It learns from past data. This helps maintenance teams fix issues before they cause downtime.
What advantages does AI-powered maintenance forecasting offer?
AI-powered maintenance forecasting has many advantages. It uses advanced analytics to boost operational efficiency. Organizations can avoid unplanned downtime, cut maintenance costs, enhance equipment reliability, and boost performance.
Why is predictive analytics important for equipment maintenance?
Predictive analytics is key because it spots potential equipment failures early. It examines historical and real-time data. These tools tell maintenance teams the best times for maintenance, improving asset reliability and availability.
How are AI models trained for predictive maintenance?
AI models for predictive maintenance are trained with historical data. This includes past failures, maintenance records, sensor data, and operational data. This training helps the models identify patterns and anomalies indicating maintenance needs, better predicting faults.
Can predictive maintenance strategies be optimized?
Yes, predictive maintenance strategies can get better over time. This happens as machine learning algorithms process more data and results. The ongoing learning anticipates failures more accurately and schedules maintenance to avoid unnecessary work.
What is condition-based maintenance and how does AI play a role?
Condition-based maintenance happens based on equipment's actual condition, not a set schedule. AI analyzes sensor data in real-time to assess condition. It recommends maintenance only when needed, optimizing operations and avoiding unnecessary tasks.
How does real-time data monitoring contribute to proactive asset health monitoring?
Real-time data monitoring aids in proactive asset health by assessing equipment condition through sensors. It instantly detects issues, allowing for swift correction before they worsen. This helps keep operations smooth.
What are the benefits of predictive analytics for equipment reliability?
Predictive analytics for equipment reliability helps foresee and prevent breakdowns, tailor maintenance schedules, and prolong asset life. It means fewer surprises and steadier operations.
How do AI insights optimize asset performance?
AI insights optimize performance by analyzing data to find optimal operational patterns. Adjustments based on these insights improve efficiency, lessen energy use, and maximize asset throughput and life.
How does AI assist in precision maintenance resource allocation?
AI uses predictive analytics for precise maintenance planning. It identifies critical tasks, allowing for strategic scheduling of maintenance staff and resources. This approach prevents waste and maximizes impact.
In what ways do AI technologies serve as the backbone of predictive maintenance?
AI technologies are vital for predictive maintenance because they analyze large data sets, forecast maintenance needs, and learn from feedback to enhance future predictions and schedules.
What is the impact of deep learning on fault prediction in predictive maintenance?
Deep learning greatly impacts fault prediction in predictive maintenance. It uses complex networks to analyze patterns undetectable by simpler methods. This improves fault prediction, making maintenance more reliable and efficient.
Comments ()