Robust Data Annotation for Autonomous Vehicles
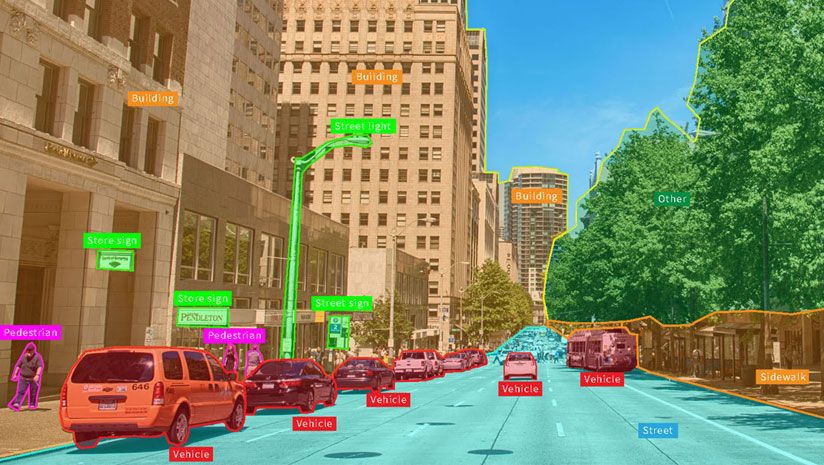
Creating models to operate a self-driving vehicle safely is the job of well-designed training data. The data must be robust and reflective of the world. For example, cars operate in different weather, road conditions, and environments.
The actions of other manned vehicles are often unpredictable. Cars must predict and incorporate these circumstances into their operational guidelines.
How do self-driving cars use annotated data?
Data annotation is the key to making this happen. Self-driving cars are complex pieces of technology. There isn't a single "algorithm" that controls its actions. Everything from the steering to speed to accident avoidance must use these training datasets.
Although self-driving cars use many types of sensors, all try to make sense of surrounding objects. Being able to make that sensory data machine-readable is central to the operation of the car.
Because these cars will have to receive and interpret this data in real time, this training data is vital. Moreover, the vehicle must reach some level of understanding and then make important decisions. A road is a high-stakes environment. Therefore, the algorithms that make those decisions must be trustworthy and precise.
How do self-driving cars make sense of incoming data?
There are several ways that self-driving cars can make sense of incoming sensory data. It depends on the type of sensor being used, the intention of the model, and the kinds of incoming data. Some of the most common types of sensors are:
- Radar
- LiDAR
- Infrared/thermal-imaging cameras
- Sonar
- GPS tracking
- Vehicle data
The types of data each of these devices produce can be very different. Modern self-driving vehicles often use many different kinds of sensors at once. The operational framework of the cars will often integrate these data sources into a single model. There are interesting videos online that show what the world looks like to the sensors in a self-driving car.
For example, radar bounces radio waves off surrounding objects to determine their position and size. LiDAR does virtually the same, using lasers instead of radio waves. Knowing the expected movement patterns and size can help these cars predict future actions. For example, radar/LiDAR systems can identify other cars and encourage certain maneuvers. This is especially important when it comes to pedestrian safety.
The same is true of thermal cameras mounted on these vehicles. Thermal data adds a new dimension to the kinds of annotations needed. The best action for a vehicle to take depends on feedback from this data. A car is not the same as a bike. A bike is not the same as a motorcycle. Being able to identify the unique thermal profile of different objects means that more precise actions can be made when the time comes.
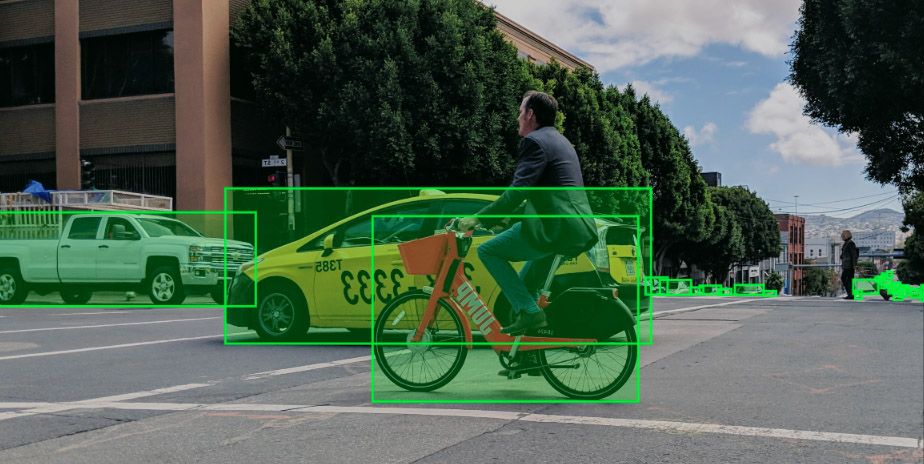
GPS data, as well as data about the speed and direction of the car, is another framing of data annotation needed for self-driving cars. Unlike humans, self-driving cars cannot make directional or operational errors. Knowing exactly what a trip should look like requires a layering of vehicle speed and location data. With a proper training dataset, mistakes can be identified sooner. This also applies to things like road closures and other possible disruptions.
What will the self-driving future mean?
The idea of self-driving cars has been around almost as long as the car itself. However, recent data analytics and manufacturing breakthroughs have allowed it to become a reality. As more self-driving cars get on the road, the amount of available data will grow. The ability to improve the "software" of a vehicle is one of the major shifts in this new world.
The challenge with self-driving cars is also the low bar for error. Because human life is often at stake with these cars, any accidents become amplified and threaten the entire industry. This means that the data used to train the initial self-driving cars matters a lot. Having a variety of sensory data helps construct a comprehensive world model. However, knowing exactly what that data demonstrates is much more critical.
Conclusion
Many experts and AI adepts talk about a future when some roads and cities only allow self-driving cars. In that situation, This is why getting the data right now might have drastic effects on the future. One day, those cars might need to learn how to best operate with other self-driving cars. If we create well-designed algorithms now, technology can advance to new levels in the future.
Comments ()