Success Stories: Projects Using Label Studio
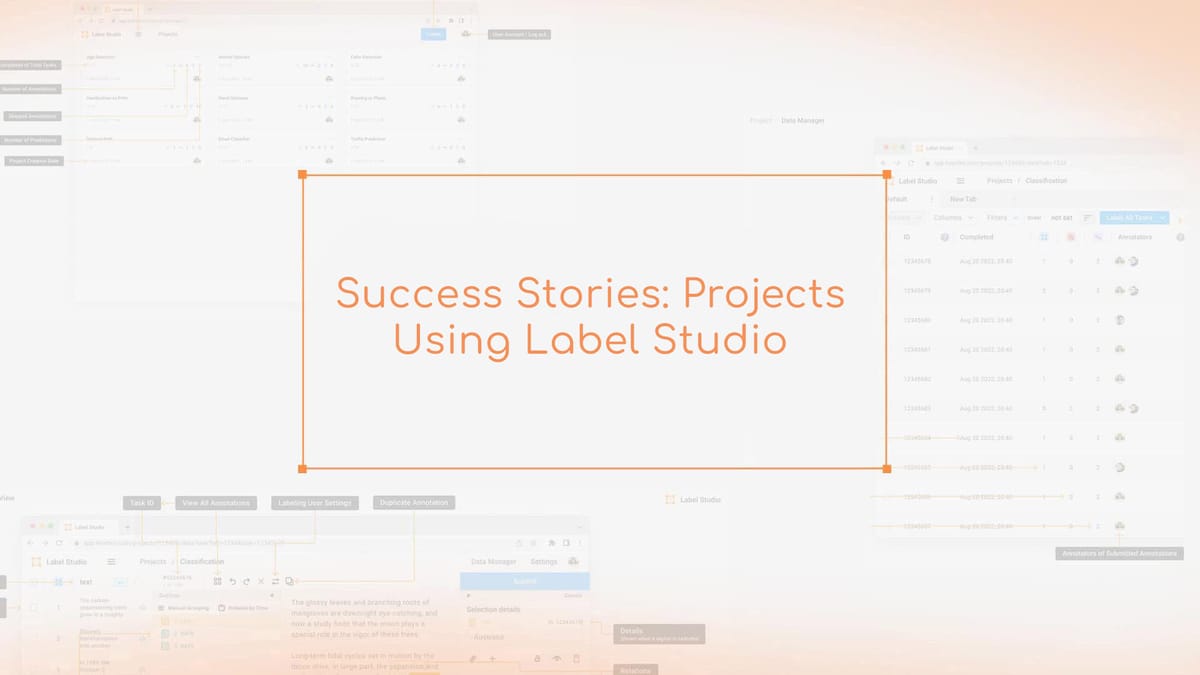
Curious about how Label Studio can revolutionize your AI projects? Dive into the possibilities and boost your team's labeling efficiency. Here, innovation meets precision in the fast-paced world of machine learning.
Introduction to Label Studio and Data-Centric AI
The evolution of the data labeling ecosystem has seen a significant leap forward with the advent of tools that enhance data annotation efficiency and accuracy. These tools are critical for training machine learning models. Label Studio, an open-source platform, is at the forefront. It supports data-centric ML initiatives and streamlines artificial intelligence workflows.
As the demand for high-quality training data grows, Label Studio plays a key role. It transforms artificial intelligence workflows from model-centric to data-centric. This shift places a greater emphasis on data quality, which directly impacts AI performance, over algorithmic complexity.
Label Studio makes this transformation seamless by integrating into existing workflows. It enriches these workflows with more structured and precise data labeling capabilities. The platform supports various machine learning models and automates many data labeling tasks. This allows teams to concentrate on the qualitative aspects of their work.
Feature | Description | Version |
---|---|---|
Generative AI Template Library | Enables fine-tuning of models like Llama 2 and GPT-4 | 1.8 |
Machine Learning Backend | Automates labeling tasks using AI like GPT-4 | Ongoing |
Audio Labeling Features | Enhanced tools for setting up audio data projects | 1.7 |
Integration with Amazon SageMaker | Tutorial led by notable industry expert Sarah Moir | Not specified |
OCR Optimization | Improves OCR accuracy for diverse documents using Tesseract and Python | Recent Updates |
The table above highlights Label Studio's capabilities beyond traditional data labeling tasks. It extends to complex AI-driven projects. This robust support system fosters a dynamic data labeling ecosystem that drives data-centric ML initiatives.
By focusing on these tools and integrations, your organization can stay at the forefront of the machine learning and artificial intelligence landscape. This ensures that your data works effectively for you.
Label Studio’s Role in the Evolution of Data Labeling
In today's fast-paced technological world, AI industry applications heavily rely on quality data to enhance ML model performance. Label Studio emerges as a key tool, revolutionizing the annotation process. It elevates the data prep responsibility to unprecedented levels of precision and efficiency.
Label Studio has transformed data labeling, introducing features for annotators to mark multiple regions at once. It also links results with bidirectional labels, ensuring data accuracy across AI industry applications. This precision boosts ML model performance. Team collaboration features further solidify a robust dataset, essential for effective model training.
For those handling large datasets, Label Studio's integration with TensorFlow and PyTorch is groundbreaking. This integration boosts flexibility and workflow efficiency, fulfilling the data prep responsibilities of ML teams.
To grasp Label Studio's importance in AI training frameworks, explore how Label Studio is transforming computer vision. It ensures a solid foundation for AI deployments through meticulous data preparation.
Label Studio supports various data types, including text, images, audio, and video. This versatility extends AI industry applications and improves ML model performance. It offers a unified platform for data annotation.
Label Studio's inclusion of keyboard shortcuts and ML-assisted preannotations showcases its dedication to speeding up and refining annotation. Real-time collaboration tools and advanced data management features streamline every step of data annotation.
In summary, Label Studio's extensive features and integrative capabilities underscore its vital role in the evolution of data labeling. It enhances annotation quality and efficiency, significantly boosting ML model performance and expanding AI industry applications.
Industry-Wide Adoption and Diverse Use Cases
Label Studio's impact across various industries showcases its versatility and wide applicability in tackling complex data annotation challenges. As more companies embrace AI-driven operations, tools like Label Studio have become essential. They help harness the power of NLP, vision, audio, and video data annotation.
Natural Language Processing (NLP) and Vision Applications
In NLP, Label Studio plays a key role, handling tasks from sentiment analysis to chatbot training. Recent NLP industry statistical data highlights how automated solutions and ML-assisted labeling are transforming text and vision data processing. NLP's global industry applications are significantly enhanced by features like synchronized scrolling and predictive labeling, focusing on efficiency and high data quality.
Vision applications of Label Studio also benefit from integrations with platforms like Azure AutoML and Google Vertex AI. These integrations provide robust support for image and video annotations, essential in sectors such as automotive and healthcare.
Audio and Video Data Annotation
The growth in AI applications has led to an increase in audio and video data usage. Label Studio addresses these needs with flexible annotation tools for time-synced transcriptions and bounding box labeling. This is critical in industries like entertainment and security, where precision and scalability are essential.
Global Reach: From the US to India and Europe
Label Studio's adaptability is evident in its widespread adoption from North America to Europe and Asia. Organizations worldwide value the platform's ability to manage diverse datasets and multifunctional teams. This facilitates seamless project collaborations, aligning with the increasing need for integrated machine learning solutions that transcend geographic and industrial boundaries.
The diverse applications of Label Studio are further highlighted by its consistent updates and improvements. This ensures it remains at the forefront of data annotation technologies.
Feature | Application | Industry Impact |
---|---|---|
Predictive Labeling | NLP, Vision | Increases labeling speed and accuracy |
Bounding Box Labeling | Video Annotations | Enhances detail in object detection for security and surveillance |
Automated Transcriptions | Audio Data | Supports accessibility and enhances media content analysis |
ML-assisted Labeling | NLP, Vision | Improves project efficiency and data accuracy |
The Strategic Value of Label Studio in Machine Learning Investment
As businesses integrate machine learning and AI into their operations, the importance of tools like Label Studio grows. Label Studio enhances your machine learning investment strategy. It also offers diverse project examples, demonstrating effective ML initiatives across industries.
The strategic value of Label Studio is evident in its impact on data preparation and management. This is key for any machine learning system. Its efficient labeling solutions ensure data accuracy, boosting the performance of machine learning models.
This positive effect on machine learning investments is backed by real-world improvements in cost-efficiency and scalability. These are vital for long-term tech investment strategies. Label Studio's automation in data labeling overcomes challenges like maintaining consistency and managing large data sets, outperforming manual processes.
Feature | Benefits |
---|---|
Automated Data Labeling | Increases efficiency, consistency, and scalability |
Custom Labeling Templates | Enhances flexibility and adaptation to specific project needs |
Real-time Quality Tracking | Ensures high standards of data accuracy for better ML model training |
Multi-annotator Collaboration | Reduces bias and accelerates project timelines |
Adopting tools like Label Studio, which integrate seamlessly into machine learning strategies, refines data handling and analysis in ML projects. This strategic choice streamlines project operations and boosts investment returns by improving machine learning model quality and applicability. Label Studio is a critical asset for businesses aiming to fully exploit machine learning's benefits.
Projects Using Label Studio: A Collection of Case Studies
This section explores various applications and methodologies. It shows how different companies use Label Studio to achieve project success. The Sense Street case study is a prime example, highlighting strategic innovations in banking. It demonstrates how team efficiency and precise metric tracking create a strong data labeling strategy for financial contexts.
Banking Sector Innovations with Sense Street
In the financial sector, data accuracy is critical. Sense Street maximizes Label Studio's capabilities. This case study shows a significant improvement in handling complex financial data labeling. It's essential for training large language models.
This precise application boosts regulatory compliance, customer service, and fraud detection. It's a testament to the importance of accurate data in finance.
Improved Team Efficiency and Data Labeling Scope
Label Studio's deployment at Sense Street has greatly enhanced team efficiency. The tools streamline collaboration and workflows. This allows the team to handle larger datasets and broader labeling scopes efficiently.
This showcases Label Studio's scalability. It also highlights the tool's ability to maintain high team productivity. Label Studio offers project management features and collaborative workflows.
Key Metrics Used by Sense Street to Measure Success
Sense Street uses several key success metrics to ensure high-quality data labels. These include precision, speed, and the number of labels per session. Their focus on precision ensures models perform well in real-world scenarios.
This approach avoids costly misclassifications in sensitive financial environments. By using advanced technologies like Label Studio, financial institutions like Sense Street redefine their capabilities. They set new standards in financial data labeling precision and efficacy.
Challenges Overcome by Label Studio Users
Adopting Label Studio has been more than just using a tool for scalable data labeling. It has been a journey of overcoming significant technical and operational hurdles. These efforts have greatly optimized data annotation processes.
Scaling Data Labeling Alongside Team Growth
As organizations expand, the challenge of scalable data labeling becomes more apparent. With growing teams, the volume of data also increases. This poses a critical challenge in maintaining an efficient labeling process. Label Studio has been instrumental in providing scalable solutions. These solutions adapt to increased data flows without compromising speed or accuracy.
Maintaining Consistency and Quality Control in Data Annotation
Ensuring consistent quality control in data annotation is a core challenge. As data types and volumes grow, maintaining this consistency becomes more daunting. Label Studio's robust framework supports advanced configuration options and workflows. These help uphold high standards of quality control in data annotation, ensuring reliable data output for training machine-learning models.
Adapating to Complex, Multilingual Data Demands
Multilingual annotation challenges are significant when businesses scale globally. The need to handle and accurately label data across multiple languages complicates the annotation process. Label Studio adeptly manages these complexities. It provides versatile tools that cater to a broad range of linguistic data, simplifying multilingual annotation tasks.
Below is a succinct representation of the various challenges users faced and how Label Studio provided solutions:
Challenge | Label Studio Solution |
---|---|
Scaling with Team Growth | Scalable data labeling frameworks |
Quality Control | Consistent review workflows and QA features |
Multilingual Data | Support for diverse linguistic datasets |
The Influence of Label Studio on Data Annotation Efficiency
Label Studio's impact on annotation efficiency is profound. It revolutionizes ML operations and machine learning models with advanced features. These include semi-automated labeling and active learning. Label Studio refines the process, boosting both speed and quality of data annotation.
In machine learning, high-quality training data is essential. Label Studio meets this need with tools that streamline annotation tasks. It improves ML operations' scalability. Customizable annotation templates and integration with ML models like SPaCy for NER or Segment Anything for image segmentation showcase Label Studio's optimization of workflows.
Label Studio's bootstrapping labels using GPT-4 reduces initial manual effort in dataset labeling. It supports active learning, which strategically selects data samples for annotation. These features accelerate and enhance annotation efficiency. They allow data scientists to build robust machine learning models quickly.
Feature | Description | Impact |
---|---|---|
Bootstrapping Labels | Utilizing GPT-4 to generate initial labels | Reduces manual labeling effort significantly |
Semi-Automated Labeling | Combines automated pre-labeling with human verification | Speeds up annotation, ideal for large datasets |
Active Learning | Focuses on the most informative samples | Improves efficiency and label quality |
Integration with ML Models | Supports tools like SPaCy, Segment Anything | Enhances the labeling process efficiency |
Keyboard Shortcuts | Facilitate quicker navigation and action | Increases labeling speed by up to 30% |
The integration of these technologies marks significant progress in optimizing task management and human-in-the-loop methodologies. Label Studio enhances data annotation efficiency and streamlines machine learning model development and refinement.
Label Studio's Community Impact and User Feedback
Label Studio, a leading platform for data annotation, thrives on community engagement and feedback. It continuously evolves, thanks to insights from its users. This evolution supports the growth of machine learning tools.
Community Survey Insights and Actionable Feedback
Every year, community surveys highlight the platform's strengths. Users praise its flexibility and user-friendly interface. This feedback drives improvements, keeping Label Studio at the forefront of AI solutions.
Collaborative Knowledge Sharing and Open Source Contributions
Label Studio's open-source nature attracts a wide range of contributors. From researchers to tech giants, everyone can contribute. This collaborative spirit enriches the ecosystem and speeds up the development of data annotation tools.
Adoption of Feature Requests in Label Studio Development
Label Studio incorporates community suggestions into its roadmap. This has led to significant enhancements. For example, the Enterprise edition now offers better access control and cloud storage options, improving efficiency and security.
Here's a comparison of community features in Label Studio's editions:
Feature | Community Edition | Enterprise Edition |
---|---|---|
Access to Functionalities | All users access same functionalities | Role-based access and permissions |
Account Creation | Through sign-up on website | Invite link and restricted sign-ups |
Collaborative Features | Limited collaboration tools | Advanced collaboration tools and security measures |
Data Storage and Sync | Local server and manual sync | Cloud deployment and automated sync with external buckets |
These enhancements, driven by community insights and contributions, highlight Label Studio's dedication to inclusivity. This collective effort boosts feature adoption in ML tools and fosters a collaborative ecosystem for advancing machine learning.
Technological Advancements Facilitated by Label Studio
Label Studio has transformed the fields of machine learning and data science. It has done so through strategic cloud platform integration and advancements in open-source AI technology. By integrating with major cloud services like AWS, Google Cloud Platform, and Azure, Label Studio has become a key tool. It is now essential for deploying and scaling machine learning models across various industries.
More organizations are now using these integrations, leading to improved efficiency in developing and deploying machine learning models. This has resulted in more agile and responsive technological environments. Users can now engage with large-scale model repositories more easily, expanding the scope and reach of machine learning applications.
Integration with Cloud Platforms and Proprietary Systems
The seamless integration of Label Studio with extensive cloud platforms showcases its ability to adapt and enhance proprietary systems. This integration simplifies scaling machine learning solutions and optimizes data flow and accessibility. It makes Label Studio a cornerstone for successful cloud platform integration in technology-driven business models.
Expansion in Large Scale Model Repositories
As demand for sophisticated machine learning models grows, Label Studio's integration capabilities have allowed developers to effectively use large-scale model repositories. This integration supports the continuous expansion of machine learning models. These models are essential for developing advanced solutions in fields like healthcare, finance, and public safety.
Revolutionizing Open Source AI through Collaborations
Open-source AI technology, fostered by collaborations, is another area where Label Studio makes significant contributions. By promoting an open, collaborative approach, it has enabled a fertile ground for innovation. This has helped businesses and developers to leverage collective knowledge and technology. It has pushed the boundaries of what's possible with AI.
The integration and practical application of these technologies highlight Label Studio’s role as a driver of change in the modern landscape of data science and machine learning. These advancements demonstrate the platform's commitment to improving data labeling accuracy and efficiency. This is critical for training reliable machine learning models.
Conclusion
In the world of machine learning, the value of high-quality, manually labeled data is immense. Label Studio stands out as a key player in this field, making a significant impact on data labeling success. It offers a solid foundation for annotating data, vital for developing advanced AI applications. By combining Label Studio with DagsHub, the annotation process becomes more efficient and collaborative, catering to various project needs and complex workflows.
The tutorial discussed earlier highlights the essential role of machine learning in data labeling. It covers the technical aspects, from understanding Git and Python to using modest hardware for ML examples. Engaging with Label Studio’s Predictions API and Python SDK marks a significant step forward in project management. These features allow users to create custom integrations and enhance their machine learning models' performance.
By using Label Studio for your data annotation, you tap into a community-driven tool that adapts to AI's evolving needs. It simplifies project creation and facilitates bulk data exports, leading to increased efficiency in AI projects. As more industries adopt these tools, it's evident that the combination of well-labeled data and machine learning models is fundamental to AI's growth.
FAQ
What are some of the success stories involving projects using Label Studio?
Label Studio has been instrumental in numerous success stories across various sectors. In finance, Sense Street used it for large generative language models. This led to better label accuracy and increased team productivity. Other sectors have similar success stories, highlighting Label Studio's role in scaling ML projects efficiently.
How is Label Studio contributing to the advancement of data-centric AI?
Label Studio bridges the gap between high-quality training data and machine learning capabilities. It offers an open source platform for continuous data labeling. This is key to improving AI workflows and ML model performance, promoting a data-centric AI approach.
Can you detail Label Studio's role in evolving AI industry applications?
Label Studio is vital in expanding AI applications by ensuring accurate data annotation. Its versatility supports a wide range of tasks, from NLP and computer vision to audio and video annotation. This versatility meets the diverse needs of the AI industry.
What industries have adopted Label Studio, and what are some use cases?
Label Studio is widely adopted in finance, healthcare, retail, and technology. It's used for tasks like natural language processing, computer vision, and audio processing. These applications include chatbots, sentiment analysis, object detection, and voice recognition systems.
How does Label Studio add strategic value to machine learning investments?
Label Studio streamlines data annotation, allowing organizations to focus on building robust ML models. This strategic investment in quality data labeling leads to higher performing models. It gives businesses a competitive edge in their ML initiatives.
Are there any case studies available that demonstrate the effectiveness of Label Studio in real-world projects?
Yes, several case studies highlight Label Studio's effectiveness. The Sense Street case in finance is a notable example. It shows how Label Studio helped the company scale their data labeling operations and maintain quality as their team grew.
What are some of the scaling challenges Label Studio help users to overcome?
Label Studio helps manage large datasets and complex team coordination. It adapts to diverse and multilingual data requirements. This enables organizations to efficiently scale their data labeling efforts.
How does Label Studio influence annotation efficiency and ML model development?
Label Studio enhances annotation efficiency with its intuitive interface and automation features. This leads to more accurate datasets. These datasets improve the performance of AI applications when used to train ML models.
What impact has Label Studio's community had on its development?
Label Studio's community has significantly shaped its development. User feedback, surveys, and open-source contributions have driven continuous improvement. This ensures the platform meets real-world user needs, fostering ongoing development.
How has Label Studio facilitated technological advancements through its integration capabilities?
Label Studio's architecture supports easy integration with cloud services like AWS, GCP, and Azure. It also integrates with other open-source tools and model repositories. This interoperability keeps Label Studio at the forefront of AI technology advancements and ML project deployment.
Comments ()