The Future of Image Editing? Exploring the Potential of Segment Anything
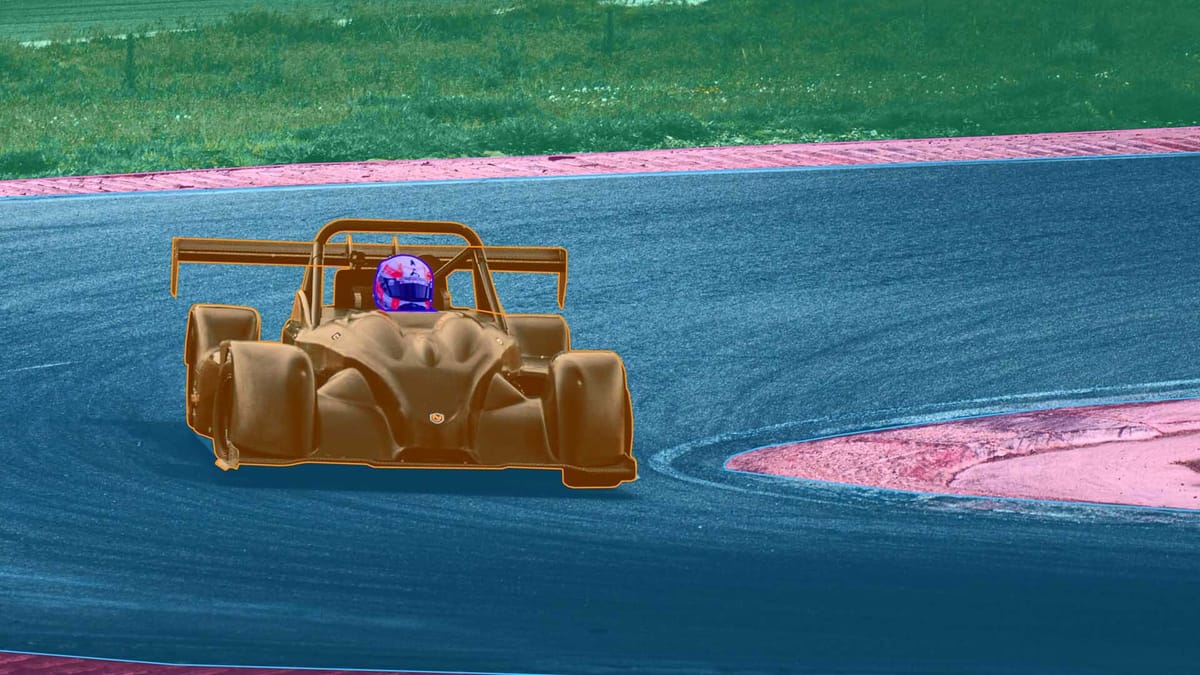
The Segment Anything Dataset (SA-1B) boasts an impressive 11 million images and 1.1 billion masks. This massive dataset marks a significant shift in computer vision, offering unparalleled precision and versatility in complex image segmentation tasks. It raises questions about the future of image editing and visual data analysis.
The Segment Anything Model (SAM), developed by Meta AI, marks a groundbreaking advancement in AI image segmentation. Unlike traditional models, SAM excels with zero-shot inference, segmenting images without extensive training. This innovation is akin to the impact of language models like GPT and BERT on natural language processing, poised to transform image editing.
Understanding SAM's innovation in visual data analysis requires exploring its core components: the image encoder, prompt encoder, and mask decoder. These elements are essential for SAM's real-time performance, making it crucial in diverse fields from augmented reality (AR) and virtual reality (VR) to autonomous vehicles and medical imaging.
Key Takeaways
- The Segment Anything Dataset (SA-1B) contains over 11 million images and 1.1 billion masks, highlighting its vast scale.
- SAM offers zero-shot inference, allowing accurate segmentation without prior specific training.
- The image encoder, prompt encoder, and mask decoder are fundamental components driving SAM's performance.
- SAM is pivotal in applications ranging from augmented reality (AR) to medical imaging and autonomous vehicles.
- Significant innovation in AI image segmentation is driven by SAM's transformative capabilities and vast data resources.
Introduction to Segment Anything
The Segment Anything Model (SAM), led by Meta AI, is revolutionizing image and video segmentation. It employs computer vision, machine learning, and deep learning innovatively. SAM responds to user prompts with unmatched efficiency, redefining how we interpret visual information.
One of SAM's key strengths is its zero-shot and few-shot learning capabilities. This makes it incredibly versatile, requiring minimal retraining. The model draws from the Segment Anything 1-Billion mask dataset (SA-1B), which includes over 1 billion masks from around 11 million images. This vast dataset enables the model to generalize across various tasks.
SAM operates at lightning speed, generating segmentation masks in just 50 milliseconds. This makes it a groundbreaking tool in computer vision. It uses advanced image and prompt encoders to process high-resolution inputs efficiently. The encoder processes an image and, combined with the decoder, predicts accurate segmentation masks.
SAM democratizes segmentation tasks, suitable for AR/VR, content creation, and scientific research. It employs interactive methods like point selection and bounding boxes for precise mask generation per object.
The Segment Anything Model (SAM) stands out for its real-time performance and reduced need for specialized modeling knowledge. Through machine learning and deep learning, SAM produces highly accurate segmentation masks. It surpasses traditional methods in speed and efficiency.
Its precision and robustness position SAM as a crucial tool for diverse applications, from urban planning to environmental monitoring. The SA-1B dataset, the largest segmentation dataset to date, enhances SAM’s performance across various tasks and domains.
Core Components of the Segment Anything Model
The Segment Anything Model (SAM) marks a leap forward in image editing and processing. It leverages advanced techniques, drawing inspiration from NLP models like GPT and BERT. This enables SAM to excel in instance segmentation, semantic segmentation, and object detection. Let's delve into the essential components that define this groundbreaking model:
Image Encoder
The Image Encoder is the cornerstone of SAM's architecture. It compresses input images into a format that facilitates analysis. This capability is vital for tasks such as instance and semantic segmentation. SAM's real-time image segmentation, accomplished in just 50 milliseconds, highlights its efficiency and the Image Encoder's strength.
Prompt Encoder
The Prompt Encoder is crucial for SAM's interactive features. It converts user inputs into embeddings the system can understand and respond to. This component ensures SAM can handle a variety of prompts, making it versatile for different object detection scenarios.
Mask Decoder
The Mask Decoder combines data from previous components to produce precise segmentation masks. Employing Generative Adversarial Networks (GANs), it generates masks crucial for in-depth image analysis. This component is key to SAM's high segmentation accuracy, vital in fields like medical imaging and autonomous driving. It also supports zero-shot inference, allowing efficient performance across various tasks without extensive retraining.
These components collectively enhance SAM’s position as a leading tool in image editing and analysis. By reducing computational costs and needing minimal supervision, SAM offers a versatile and potent solution for professionals in the industry.
How Segment Anything Works
The Segment Anything Model (SAM) is a pioneering tool in image segmentation, expanding the limits of computer vision. This section explores SAM's core features and its groundbreaking performance in real-time annotation.
Promptable Segmentation
Prompt-based segmentation is central to SAM. Users guide the model with various prompts like clicks, bounding boxes, and text. This method offers flexible image editing for a wide range of tasks. Users can specify regions in an image for precise and adaptable results.
This flexibility helps SAM tackle diverse challenges, from detailed object boundaries to selecting entire regions.
Real-time Interactive Performance
SAM stands out with its real-time annotation. It works smoothly in web browsers, offering immediate results that boost user experience and efficiency. The model balances performance and quality, ensuring reliable segmentation across various objects and contexts.
This instant feedback is vital for applications needing quick adjustments and validations, like medical imaging and autonomous vehicles. SAM also produces multiple valid masks when uncertain, ensuring robust performance in different scenarios.
In conclusion, SAM's prompt-based segmentation and real-time capabilities mark a significant advance in computer vision. Its versatility in adapting to various tasks and continuous learning makes it a valuable tool for both simple and complex image segmentation needs.
Segment Anything Dataset (SA-1B)
The Segment Anything Dataset, or SA-1B, is a cornerstone in the Segment Anything Model (SAM). It has transformed large-scale image analysis. With over 11 million images and more than 1 billion masks, SA-1B is the largest segmentation dataset available. This dataset opens up a broad spectrum of applications and research opportunities, paving the way for future advancements in computer vision.
Dataset Overview
SA-1B is essential for SAM's data-driven model performance, offering a vast, diverse image collection. It trains SAM to perform promptable segmentation with zero-shot performance across various tasks. This dataset's comprehensive nature allows SAM to tackle segmentation challenges with higher accuracy than traditional methods.
Moreover, SA-1B's scale and diversity are unmatched in supervised machine learning, providing deep insights and applications. This diversity enables SAM to manage a wide range of real-world scenarios, from everyday settings to complex environments.
Stages of Data Annotation
The creation of SA-1B involves a multi-stage annotation process to enhance SAM's image analysis capabilities. These stages include:
- Assisted-Manual Annotation: Annotators interact with SAM, using its real-time mask generation to provide initial annotations.
- Semi-Automatic Annotation: SAM refines these annotations with minimal human input, reducing annotator workload while increasing speed and accuracy.
- Fully Automatic Annotation: In the final stage, SAM annotates images autonomously, achieving high precision and consistency.
This process integrates human expertise with supervised machine learning, enhancing SAM's efficiency and performance. This continuous improvement not only boosts SAM's current capabilities but also sets the stage for future advancements in computer vision technology.
The SAM model and its vast SA-1B dataset are released under an Apache 2.0 license, fostering open research and development. This openness allows academics, researchers, and developers to build upon SAM's foundation, driving innovation in image segmentation and beyond.
Stages of Data Annotation | Description | Benefits |
---|---|---|
Assisted-Manual Annotation | Interactive use of SAM by human annotators | Initial precise annotations with real-time feedback |
Semi-Automatic Annotation | Human-guided refinement with minimal intervention | Increased speed and improved accuracy |
Fully Automatic Annotation | Autonomous image annotation by SAM | Consistency and high precision across datasets |
Revolutionizing Image Editing: Practical Applications
The Segment Anything Model (SAM) is a game-changer, offering transformative applications across various fields. It uses cutting-edge techniques like pixel-level classification, residual blocks, and attention gates. This makes SAM a key player in revolutionizing how we interact with images in different areas.
Augmented Reality (AR) and Virtual Reality (VR)
SAM is a game-changer for AR/VR, enabling precise interaction with objects and understanding of the environment. These advancements lead to more immersive experiences, making virtual spaces feel real and intuitive. SAM's ability to accurately identify and segment objects within a class enhances these experiences.
Medical Imaging
In medical diagnostics, SAM improves the accuracy of analyzing microscopic images. This leads to earlier detection and better diagnosis, which is crucial for patient care. The model's focus on important image areas, thanks to residual blocks and attention gates, boosts segmentation accuracy for doctors.
Autonomous Vehicles
SAM's precision in recognizing and categorizing objects is crucial for self-driving technology. It enhances navigation and safety, allowing vehicles to make informed decisions. The model's detailed segmentation and point prompts help it adapt to real driving scenarios, ensuring reliable performance.
SAM's capabilities in these areas show its significant impact across industries. It's pushing forward AR/VR innovation, medical diagnostics, and self-driving technology. SAM is a key force in evolving image editing and analysis.
Innovations in Computer Vision: SAM’s Impact
The Segment Anything Model (SAM) has revolutionized computer vision. It overcomes traditional hurdles like over-segmentation and under-segmentation with its advanced technology. This results in higher accuracy and efficiency. SAM's zero-shot learning feature allows models to adapt instantly, marking a significant shift in AI advancements.
SAM enhances real-time applications with its prompt processing and control. Its vision transformer models excel with complex datasets, driving innovation in AR/VR, medical imaging, and autonomous vehicles. This sets a new benchmark for image segmentation.
SAM opens up numerous business opportunities, from content creation to medical applications. It also boosts robotics and product segmentation in retail. The potential for growth is immense.
Developers can use the OpenVINO toolkit to deploy SAM efficiently on consumer-grade hardware. This democratizes advanced AI, fostering innovation across various sectors.
Challenges | SAM Solutions |
---|---|
Over-segmentation | Precise object recognition |
Under-segmentation | Enhanced accuracy |
Noise and blurriness | Improved data processing |
Changing environments | Zero-shot learning adaptability |
Scalability issues | Efficient large-scale application |
Meta Platforms Inc.'s release of SAM and the SA-1B dataset showcases cutting-edge technology. This model can create masks for any object in images or videos without prior training. Its adaptability promises to transform the AI landscape, setting the stage for future breakthroughs.
The Segment Anything Future
Looking ahead, the future of Segment Anything Model (SAM) is bright, poised to revolutionize computer vision. Its open-source nature and the vast SA-1B dataset open up new AI research avenues. This initiative not only enhances image segmentation accessibility but also spurs innovation across applications like augmented reality, virtual reality, and medical imaging.
SAM's flexibility and adaptability are key for evolving computer vision tasks. Its real-time performance fosters a more inclusive and dynamic approach. With ongoing research to improve segmentation efficiency, SAM is at the forefront of computer vision advancements.
Proposed improvements aim to enhance scoring and instance mask generation. These advancements could boost SAM's processing speed and performance, making it more viable for real-world use. FastSAM, a notable variant, significantly increases efficiency, running 50 times faster than SAM. This could be a game-changer for industries needing swift image analysis.
In the realm of future predictions, FastSAM's rapid image segmentation capabilities are groundbreaking. It's particularly beneficial for applications requiring quick results, such as autonomous driving and real-time video analysis. FastSAM's advancements highlight the evolving landscape of AI research, suggesting a future where sophisticated models operate more efficiently.
The SA-1B dataset, the largest segmentation dataset ever, is a crucial resource for the computer vision industry. Its vastness enables the development of more robust and reliable models capable of complex tasks. This dataset is a stepping stone for significant computer vision advancements.
In summary, the Segment Anything project and its variants like FastSAM represent a forward-thinking approach in computer vision. The ongoing enhancements and AI research opportunities signal a shift towards a more democratized and innovative future for image segmentation and analysis.
Comparing SAM with Traditional Segmentation Approaches
The evolution of image segmentation highlights the gap between the Segment Anything Model (SAM) and traditional methods. This comparison focuses on segmentation efficiency, the contrast between manual and automatic annotation, and the impact of deep learning models.
Interactive Segmentation
Traditional methods for interactive segmentation require iterative refinement and significant manual effort. Annotators must painstakingly outline objects, leading to lengthy and labor-intensive processes. SAM, however, leverages manual prompts to enhance segmentation efficiency. Studies indicate SAM excels with manual hints, such as box prompts, outperforming current interactive methods at a minimal number of points. Yet, its effectiveness may wane with an increase in points.
In interactive settings, deep learning models like SAM show sensitivity to randomness in center points and precise box prompts. Nevertheless, SAM's performance improves significantly when tailored for specific tasks. For instance, in medical image segmentation, fine-tuning led to a notable improvement in average DICE performance by 4.39% for ViT-B and 6.68% for ViT-H. This underscores its adaptability and precision in interactive scenarios.
Automatic Segmentation
Traditional automatic segmentation relies heavily on extensive training datasets. This requirement often limits their flexibility and increases computational demands. SAM transforms this by excelling in scenarios where both manual and automatic annotation are involved. Zero-shot evaluations reveal that SAM and FastSAM can achieve competitive performances with fewer parameters, even with limited training data.
For instance, FastSAM offers 50 times faster runtime than SAM while maintaining similar performance metrics with just 1/50th of the SA-1B dataset. This efficiency not only enhances productivity but also reduces computational load, making it suitable for real-time applications across various fields. Moreover, SAM's efficiency is further demonstrated in anomaly detection tasks on the MVTec AD dataset, segmenting nearly all regions with certain limitations in precision compared to manual methods.
Comparative metrics and practical applications highlight that SAM and its variants, such as FastSAM, offer a substantial improvement over traditional techniques. This is particularly evident in building extraction from remote sensing imagery, where SAM performs robustly against noise interference. However, it may fall short in specific scenarios like shadow-related regions, indicating areas for future enhancements.
Model | Task | Performance | Efficiency |
---|---|---|---|
Traditional Models | Interactive Segmentation | High Detail, Time-Consuming | Lowest |
Traditional Models | Automatic Segmentation | Depends on Extensive Datasets | Varies |
SAM | Interactive Segmentation | Improved with Prompts | High |
SAM | Automatic Segmentation | Zero-shot Efficiency | High |
FastSAM | Multiple Tasks | Comparable to SAM | Up to 50 Times Faster |
Summary
Our journey through the Segment Anything Model (SAM) concludes with a clear understanding of its transformative impact on image segmentation. SAM merges NLP foundation models with cutting-edge visual data processing. This fusion creates a powerful solution that outpaces traditional methods. The Segment Anything 1-Billion mask dataset (SA-1B) highlights SAM's scalability and adaptability, marking it as the largest dataset in image segmentation. These advancements signal a new era of vision processing innovation.
SAM breaks through traditional model limitations by creating masks for a wide variety of objects in images and videos. It does so without needing specific training data. This versatility makes it invaluable in fields like augmented reality (AR), virtual reality (VR), medical imaging, and autonomous driving. The integration of SAM with stable diffusion inpainting through the Ikomia API broadens its application scope, showcasing its potential in image processing.
Users of SAM have access to a wealth of resources. Meta AI Research publications and the SAM GitHub repository provide deep technical insights and practical examples. The model's performance metrics, including Intersection over Union (IoU), Precision, Recall, and F1 score, demonstrate its excellence in object detection and segmentation. As ongoing research refines and expands SAM, it marks a significant leap in computer vision applications. SAM is redefining how we engage with digital images, making computer vision more intelligent, accessible, and powerful.
FAQ
What is the Segment Anything Model (SAM) developed by Meta AI?
The Segment Anything Model (SAM) is a cutting-edge tool by Meta AI for enhancing image editing and computer vision. It excels in identifying and segmenting objects within various settings using user prompts. This is achieved without the need for additional training.
What are the core components of the Segment Anything Model?
At its core, SAM comprises an image encoder that condenses input images into a matrix for detailed analysis. It also includes a prompt encoder that translates user prompts into embeddings. Lastly, a mask decoder synthesizes this information to produce segmentation masks.
How does SAM perform in real-time?
SAM delivers real-time interactive performance. This enables users to engage with the model directly within web browsers, receiving immediate segmentation results.
What makes the Segment Anything Dataset (SA-1B) unique?
The Segment Anything Dataset (SA-1B) stands out for its immense scale and diversity. It boasts over 1.1 billion masks on 11 million images. This dataset serves as a comprehensive training ground for SAM, driving extensive research and applications in computer vision.
How does promptable segmentation work in SAM?
SAM's promptable segmentation empowers users to steer the model using diverse prompts like clicks, boxes, or text. This adaptability ensures high effectiveness across a spectrum of segmentation tasks.
What are some practical applications of SAM?
SAM finds its applications in various fields. These include enhancing object interaction in AR/VR, segmenting microscopic images in medical imaging, and improving object identification and classification in autonomous vehicles.
How does SAM compare to traditional segmentation methods?
SAM diverges from traditional segmentation methods, which often necessitate substantial manual effort or extensive training data. It integrates manual and automated inputs through its promptable interface. This approach delivers accurate segmentation masks efficiently, without prior tailored training.
What does the future hold for SAM and its impact on computer vision?
SAM heralds a future where AI in computer vision is more inclusive and versatile. Its open-source nature and the vast SA-1B dataset foster ongoing research, industry collaboration, and the development of advanced machine learning models.
What role does SAM play in transforming computer vision and AI technology?
SAM marks a pivotal innovation in computer vision, enabling zero-shot learning. This allows technology to adapt swiftly to new challenges without the need for model retraining. Consequently, it significantly shapes the future of AI.
Comments ()