The Future of Roboflow: Exploring New Tools and Advancements
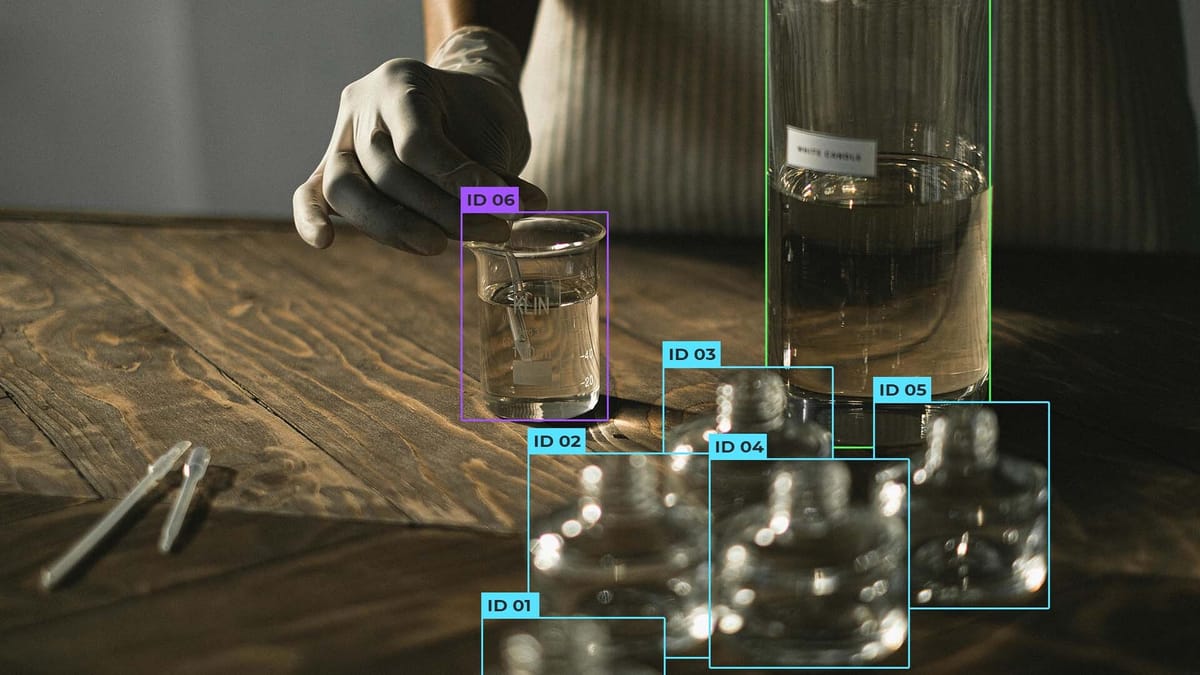
As we move forward into 2024, Roboflow has introduced 12 new enhancements, showcasing its dedication to transforming AI models and AI technology. These enhancements span from model-assisted labeling to better annotation tools and expanded REST API and Python SDK capabilities. Roboflow is setting a new benchmark for training and deploying AI models. It's making it easier for developers and organizations to unlock the full potential of AI.
Roboflow's latest updates reflect its commitment to expanding the horizons of computer vision. Their focus is on practical applications, not just theoretical discussions. This approach is driving real-world impact across various sectors.
Key Takeaways
- Significant improvements include model-assisted labeling and enhanced annotation tools.
- Expansion of REST API and Python SDK for enhanced capabilities.
- Roboflow's updates aim to make programmed human experiences more accessible and comprehensive.
- Computer vision technology facilitates valuable insights through visual media analysis such as photos and videos.
- Integration of computer vision data enhances machine learning model performance and decision-making processes across industries.
Introduction to Roboflow and Its Impact on AI
Roboflow is at the cutting edge of transforming computer vision, cutting the cost of developing computer vision solutions by over tenfold compared to traditional methods. It offers hosted GPU training, enabling developers to create custom models swiftly, thus optimizing time and resources. The platform boasts a vast library of 50,000 pre-trained models on Roboflow Universe, designed to boost project efficiency and facilitate the rapid deployment of custom models within a week.
Recent Developments and Achievements
Roboflow has made significant strides in refining data and image annotation processes. It supports a variety of image formats, including JPG, PNG, BMP, and TIF, ensuring flexible data management. The platform integrates with popular annotation tools like CVAT, VoTT, and LabelImg, enhancing annotation capabilities. Roboflow Organize efficiently manages large datasets, allowing for the creation of up to 50 new versions of each image for dataset variety.
Roboflow Annotate has attracted over 250,000 engineers, offering features like label assist and collaboration. Additionally, its tools for data augmentation, including flips, rotations, shear, and blur, aim to improve model performance. The future of Roboflow looks promising, with its development tools streamlining computer vision projects, saving time, and reducing costs.
Roboflow's version control system tracks changes in datasets and allows for rolling back as needed, supporting over 15 export formats and more than 40 for organizing datasets. This robust infrastructure supports over 10,000 projects globally, with more than 100,000 developers using their services. Image annotation, bolstered by these innovative features, continues to drive the advancement of AI powered by Roboflow.
New Tools for Model Training and Annotation
In the evolving world of AI, Roboflow has introduced significant updates that boost the efficiency of creating and refining AI models. These updates include new tools like model-assisted labeling and enhanced annotation features. These tools streamline the model training process, leading to higher accuracy through easier data handling.
Model-Assisted Labeling
Roboflow's toolkit features model-assisted labeling, a technology that simplifies the complex task of data labeling. This is crucial for training AI models. For example, using a dataset of screws, you can train a quality assurance model for manufacturing facilities efficiently.
To start, upload images and annotations to Roboflow, ensuring a balanced mix of classes. Begin with 5-10 images for an effective model training session. Tools like Smart Polygon enable detailed annotations using polygons, significantly boosting model performance. Roboflow supports over 40 computer vision formats, making the process flexible and comprehensive.
Enhanced Annotation Tools
Roboflow also offers a suite of enhanced annotation tools to speed up the annotation phase. These tools enhance the traditional process with features like bounding box drawing for objects of interest. They also provide various augmentation options, including resizing, flipping, rotations, and contrast adjustments. Start with one or two augmentations to improve model generalization and initial training outcomes.
For projects, aim to annotate about 90 images per class and strive for around 300 images per class for superior performance. Roboflow allows dataset splits into Train, Test, and Valid sets, aiding thorough training and validation. This structured approach ensures your AI models are well-trained and deliver accurate results.
Feature | Description | Benefit |
---|---|---|
Model-Assisted Labeling | Simplifies data labeling by leveraging AI | Reduces time and effort in manual labeling |
Smart Polygon | Enables polygon-based labeling | Improves annotation accuracy and model performance |
Data Augmentation | Includes resizing, flipping, rotations, and more | Enhances model generalization capability |
Dataset Splits | Allows creating Train, Test, and Valid sets | Facilitates effective training and validation |
Advancements in Computer Vision and Machine Learning
Roboflow leads in generative AI within computer vision, revolutionizing how software understands and processes visual data. These AI advancements are crucial across various fields, from identifying objects to generating digital assets for virtual worlds. They expand traditional AI's limits.
Role of Generative AI in Computer Vision
Generative AI in computer vision is growing swiftly. It produces artificial data for training more powerful and adaptable machine learning models. For example, Rivian Automotive Inc. uses computer vision and AWS for quality checks in manufacturing, showing these technologies' practical use.
Generative AI's impact is clear through its contributions to datasets like PASCAL VOC, ImageNet, and COCO. These datasets have greatly advanced computer vision research. They provide high-quality data for various vision-related problems, boosting machine learning abilities.
Roboflow's commitment to computer vision is seen in Roboflow Universe. This platform hosts fifty open source projects from launch partners, offering accessible, high-quality datasets for researchers. It also includes Roboflow Train, a simple solution for training models without deep neural network knowledge.
Roboflow's work in environmental monitoring through computer vision highlights its practical uses. By analyzing aerial images, computer vision models can track crop health, sort waste, and support sustainable urban growth. This technology is vital for sustainability efforts.
Future of Roboflow
Roboflow is ushering in a new era of innovation by continually improving its platform. These improvements aim to boost the tech landscape, empower developers, and nurture a thriving Roboflow community. The platform's future is deeply rooted in its dedication to developer empowerment and community engagement, ensuring a comprehensive and enriching AI development journey.
Innovative Features and Functionalities
The platform has been enhanced with a UI creator for better workflow integration, offering features like filtering and conditional branching. The addition of Universal Query Language (UQL) allows users to construct transformation chains and evaluate boolean expressions, simplifying AI projects.
Enhancements also include the default representation of predictions under sv.Detections and advancements in video processing. Users can now process multiple videos simultaneously using InferencePipeline and workflows. These updates highlight Roboflow's commitment to addressing the evolving needs of its users.
Developer Empowerment and Community Engagement
The Roboflow community benefits from initiatives like the Roboflow Universe, providing extensive datasets and images via open APIs. Models can also be deployed to popular cloud services like AWS Sagemaker and Google’s Vertex through APIs, showcasing Roboflow's effort to simplify integration and empower developers.
Roboflow addresses AI bias concerns by offering tools that enhance explainability, accessibility, and discoverability. CEO Joseph Nelson stresses the importance of creating such an ecosystem for the ongoing advancement of AI technologies.
Integration with Large Language Models (LLMs)
Roboflow's integration with Large Language Models (LLMs) marks a significant leap in AI capabilities, enhancing its precision in complex tasks. This integration leverages the transformer architecture, first introduced in 2017, to mimic human language processing. This innovation is set to transform various industries, unlocking new efficiencies and possibilities.
LLMs and Multimodality
Large Language Models excel in analyzing vast amounts of text through self-supervised learning. They can train on enormous amounts of unlabeled text, capturing semantic relationships without human annotation. This capability allows them to produce coherent, contextually relevant text.
Furthermore, these models extend their reach beyond text analysis. They can process and generate text, images, and audio, replicating human information processing across different modalities. The MM1 model, with 30 billion parameters, stands out in visual question answering, in-context predictions, and few-shot learning with instruction tuning.
Real-World Applications and Use Cases
The integration of LLMs has far-reaching implications for real-world applications. Tools like ChatGPT by OpenAI and BERT by Google have transformed natural language processing across sectors. These applications span content creation, legal assistance, coding, scientific research, and screenplay writing with tools like Dramatron, which organizes scripts for enhanced context.
Large Language Models support complex tasks such as literature reviews and domain-specific knowledge exploration. MM1's architecture benefits from pre-training data mixes, optimizing performance with a blend of image-caption pairs and interleaved image-text data. This versatility makes it a key tool in AI-driven innovations.
Autonomous Systems and Intelligent Automation
Roboflow's innovations are at the forefront of the autonomous systems and intelligent automation growth. They're making significant strides in autonomous vehicles and robotics. These advancements address the specific needs of these industries, boosting performance and safety.
Applications in Autonomous Vehicles and Robotics
Intelligent automation in autonomous vehicles is set to transform transportation. It aims to cut down human error, which causes nearly 94% of road accidents, as stated by the American National Highway Traffic Safety Administration (NHTSA). This could greatly improve safety. Intel projects that autonomous vehicles will save over half a million lives and reduce commuting time by 250 million hours annually between 2035 and 2045.
In robotics, intelligent automation boosts the capabilities and adaptability of robotic systems across various sectors. Autonomous robots are now key in manufacturing, healthcare, and logistics for their ability to perform complex tasks with high precision.
Visual Perception and Mirroring Physical Experiences
Roboflow is revolutionizing the connection between visual perception and physical experiences with its computer vision advancements. These innovations are key to enhancing metaverse applications. By allowing users to interact with digital versions of real-world objects, Roboflow's technology is creating more immersive virtual spaces.
The potential of metaverse applications is vast, especially in simulating physical experiences. Models like YOLO-World, with an Average Precision of 35.4 on the LVIS dataset, showcase the field's progress. Integrating vision and language models, such as CLIP, boosts object detection in real-time, highlighting the model's capabilities.
Applications in the Metaverse
In the metaverse, the applications are numerous. YOLO-World's efficiency, operating at 52.0 frames per second on the V100 platform, underscores its real-time detection prowess. It also introduces open-vocabulary instance segmentation, demonstrating exceptional performance across datasets. Grounding DINO combines deep learning with grounded pre-training for superior open-set object detection, enhancing visual perception.
The F-VLM method has improved mask AP by +6.5 points over the previous benchmark on LVIS, highlighting advancements in model robustness and accuracy. These improvements are crucial for metaverse applications. Techniques like "prompt-then-detect" in YOLO-World eliminate real-time text encoding, making object detection more intuitive.
For insights into how gaze estimation systems are improving human-computer interactions, explore the provided research.
Roboflow's computer vision innovations are not just about recognizing virtual objects. They're about creating a harmonious blend of digital and physical worlds. These advancements are central to developing metaverse applications that offer realistic and engaging experiences, merging physical experiences with enhanced visual perception.
Summary
Roboflow's journey highlights an exciting future with new tools and advancements set to transform AI. With over 250,000 users worldwide, it's clear that Roboflow's developer tools are making a significant impact. The Roboflow Universe alone boasts more than 200,000 labeled datasets and 150 million images, featuring over 500,000 unique classes of objects. This vast collection, along with 50,000 fine-tuned models and 800+ pre-trained YOLO models, underscores the platform's strength.
Roboflow is continually pushing boundaries with its comprehensive training solution, Roboflow Train, which has gained widespread adoption. The integration of active learning methods ensures continuous improvement of computer vision models. Developers can refine their data collection strategies, leading to enhanced model accuracy. HITL (Human-in-the-Loop) labeling elevates dataset quality, combining manual input with professional expertise for superior annotations.
The Roboflow community, with its contributions to over 660,000 tutorial views on the Roboflow YouTube channel and support for more than 100,000 visitors on the User Forum, stands as a cornerstone of shared knowledge and innovation.
FAQ
What is the future of Roboflow in the realm of computer vision and AI models?
Roboflow is at the forefront of AI and computer vision innovation. They aim to make human experiences programmable. Recent updates include model-assisted labeling, enhanced training, and expanded REST API and Python SDK.
What recent developments has Roboflow introduced?
Roboflow introduced 12 new enhancements in early 2023. These include better model-assisted labeling, training, annotation tools, and expanded REST API and Python SDK. These updates highlight their commitment to AI technology advancement.
How do Roboflow's model-assisted labeling and annotation tools benefit AI models?
Model-assisted labeling and enhanced annotation tools make data labeling more efficient. This is crucial for training accurate AI models. These tools cut down manual labor, speeding up the development of machine learning models.
What role does generative AI play in Roboflow's computer vision advancements?
Generative AI is essential for interpreting and processing visual data like humans do. It's crucial for tasks like object identification and creating digital assets for virtual worlds.
What is the significance of Roboflow's integration with Large Language Models (LLMs)?
Combining LLMs with Roboflow's AI enhances decision-making by adding reasoning and critical thinking. This integration boosts AI's capabilities in real-world applications.
What advancements has Roboflow made in data annotation and object detection?
Roboflow has made significant strides in data annotation and object detection. These advancements reduce manual effort, improve quality, and enhance model precision. This is crucial in areas like autonomous automotive manufacturing.
How are Roboflow's AI advancements applicable to autonomous systems and intelligent automation?
Roboflow's technologies are key to the growth of autonomous systems and intelligent automation. Their AI solutions meet the needs of these industries, improving visual quality assurance and other critical processes.
How is Roboflow contributing to visual perception and the metaverse?
Roboflow is connecting virtual and physical worlds by enabling the recognition and interaction with digital objects. This is crucial for creating immersive and realistic virtual environments in the metaverse.
Comments ()