Industry Adoption of Synthetic Data: Case Studies and Trends
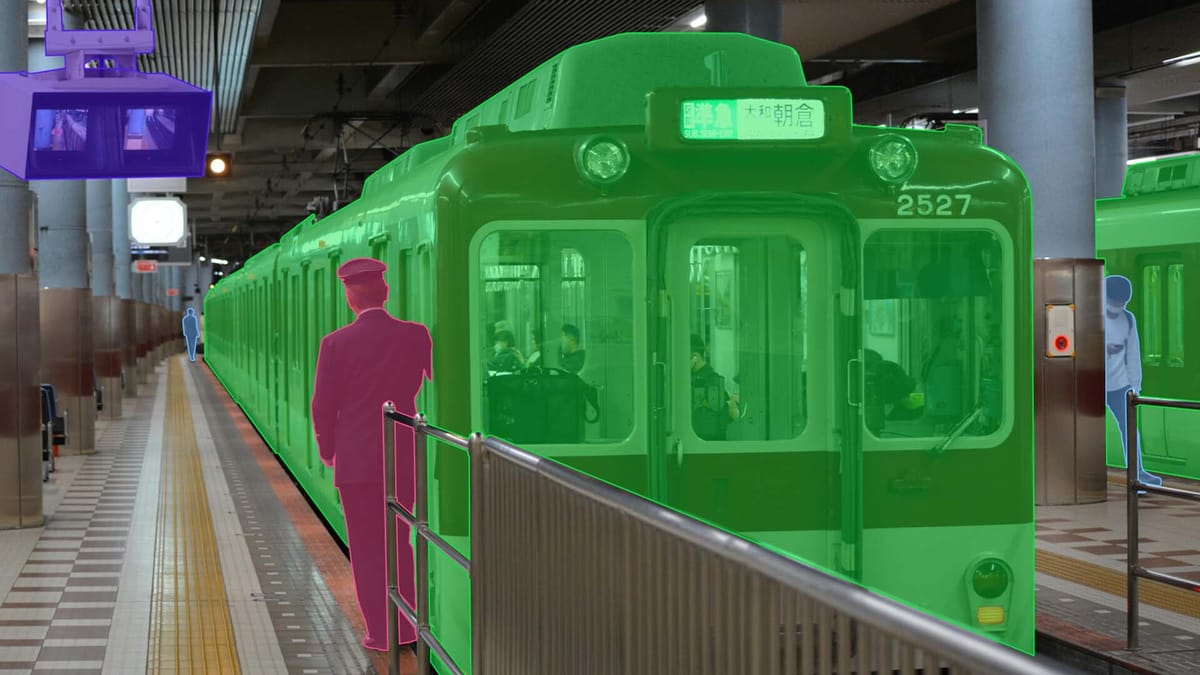
In a world overflowing with data, a significant shift is underway. By 2024, 60% of AI development data will be synthetic. The synthetic data market is rapidly expanding, expected to hit USD 2,339.8 million by 2030. This growth, with a CAGR of 35.3%, highlights synthetic data's critical role in various sectors. Industries like healthcare, finance, and automotive are eager to leverage its benefits, including data protection.
Agent-based modeling dominated the market in 2023, showing the sector's rapid progress. Companies like Applied Intuition and Synthesis AI have achieved significant valuations, illustrating synthetic data's financial appeal. Success stories, such as Waymo's simulated driving data, underscore its role in advancing self-driving technologies. Through detailed case studies, it's evident that synthetic data is more than a supplement; it's a cornerstone of digital transformation in the 21st century.
Key Takeaways
- The synthetic data market size is expanding rapidly, expected to surpass USD 2 billion by the end of the decade.
- With a Compound Annual Growth Rate (CAGR) of 35.3%, synthetic data's prevalence across industries is accelerating significantly.
- Agent-based modeling and predictive analytics are becoming focal points in the synthetic data space.
- Healthcare and automotive sectors showcase remarkable success stories in the application of synthetic data, fostering innovation and efficiency.
- From startups to tech giants, the industry's embrace of synthetic data showcases its transformative impact on AI and machine learning.
Exploring the Growth of Synthetic Data Across Sectors
The field of data science is constantly evolving, with synthetic data adoption at its forefront. Healthcare, automotive, and finance sectors are embracing synthetic data. It helps maintain privacy while delivering valuable insights.
Synthetic data accurately replicates real data without breaching privacy. This is critical under strict regulations like GDPR and HIPAA. Technologies like Generative Adversarial Networks (GANs) and data masking have elevated industry use cases. For example, industries using synthetic data are finding effective solutions where data privacy is essential.
sector trends show a strong move towards synthetic data for innovation. It's used to improve machine learning and real-time decision-making. Companies like Waymo and Tesla are leading this shift, using synthetic data for advanced autonomous driving.
Industry | Synthetic Data Adoption Growth | Operational Impact |
---|---|---|
Healthcare | 50% increase by 2025 | Enhanced disease prediction models |
Automotive | 70% increase over 5 years | Accelerated ADAS development |
Finance | 65% adoption rate | Better fraud detection systems |
Retail | 30% growth in use | Improved personalization |
Manufacturing | 58% increase in decade | Efficient quality control |
Despite ongoing challenges in dataset realism and complexity, synthetic data's future looks bright. It has vast possibilities to change how businesses handle data analytics, privacy, and compliance. The rapid adoption of synthetic data across various sectors highlights its role as a key technological asset and a cornerstone in modern data strategy.
Understanding Synthetic Data: Definition, Development, and Deployment
In today’s digitized era, synthetic data definition is key to grasping its role and importance across various sectors. Synthetic data, created artificially, mirrors real-world data's statistical aspects without revealing sensitive information. This ensures privacy while keeping data useful. It has significant implications for sectors like healthcare, finance, and automotive, where data privacy is critical.
Defining Synthetic Data
Synthetic data includes various forms like tabular data, text, images, and videos. It is generated through advanced software models to mimic the statistical properties of original data sets. This allows machine learning models to train on large, diverse datasets without risking private information.
Historical Perspective and Advancements
The evolution of synthetic data generation techniques has seen significant growth. From simple randomized numbers to complex Generative Adversarial Networks (GANs) and Variational Autoencoders (VAEs), the progress is notable. This evolution highlights the growing importance of privacy-enhancing technologies in protecting user data while ensuring data quality for analysis.
Methods for Generating Synthetic Data
Today, various techniques are used to create high-quality synthetic data. Key methods include:
- GANs (Generative Adversarial Networks)
- VAEs (Variational Autoencoders)
- Transformer Models for sequential data prediction
- LSTM (Long Short-Term Memory networks)
- Markov Chains for simpler probability-based models
The table below compares several synthetic data generation techniques. It outlines their applications and benefits:
Technique | Common Use Cases | Advantages |
---|---|---|
GANs | Image and video synthesis | High-quality outputs, good at capturing complex distributions |
VAEs | Data anonymization, image generation | Efficient in handling missing data, provides smooth latent space for interpolation |
Transformer Models | Text and sequential data generation | Handles dependencies in data effectively, scalable to large datasets |
LSTM Networks | Time-series prediction, sequential text generation | Ability to remember long sequences, useful for time-dependant data |
Markov Chains | Stochastic modeling, simple probabilistic scenarios | Easy to implement, good for modeling discrete systems |
The use of these diverse methodologies expands the scope of applications. It also strengthens security frameworks by creating detailed yet anonymous data. This ensures data is both useful for analysis and compliant with privacy regulations.
Industry Adoption of Synthetic Data
The field of synthetic data is rapidly changing how industries operate and innovate. It's seen as a key privacy-enhancing technology. It's most impactful in sectors like healthcare, finance, and automotive. Here, it enables secure data sharing and robust AI model training without risking personal or sensitive information.
For example, Fortune 100 banks are using AI-generated synthetic data to unlock sensitive data assets. This allows for privacy-preserving machine learning at scale. It's revolutionizing risk assessments and fraud detection, improving financial model performance and security.
In healthcare and life sciences, synthetic data is vital for speeding up medical research and approvals. It meets strict regulatory demands for data privacy. Companies like Cytel Inc. and NVIDIA Corporation are leading in data-driven innovations. They're advancing clinical advancements and patient care through synthetic data.
The automotive and manufacturing sectors are also benefiting from synthetic data. It helps overcome data scarcity by generating high-quality data for training machine learning models. This is key for developing smarter, AI-driven systems. These systems can predict maintenance issues and optimize manufacturing processes without extensive real-world testing.
Dr. Nimita Limaye, Research Vice President at IDC, stated, "The transformative power of synthetic data in accelerating data availability and compliance in life sciences cannot be overstated. The adoption rate will largely depend on the industry's willingness to embrace this technology."
The market statistics show optimism for synthetic data applications. The Synthetic Data Generation Market was valued at USD 0.29 billion in 2023. It's expected to reach approximately USD 3.79 billion by 2032, growing at a CAGR of 33.05%. This indicates strong growth and widespread industry support.
Sector | 2023 Market Share | Key Contribution |
---|---|---|
Healthcare and Life Sciences | 24% | Accelerates research and compliance |
Banking and Finance | Data not specified | Enhances privacy and fraud detection |
Automotive | Data not specified | Improves ML model training |
Manufacturing | Data not specified | Optimizes production processes |
These insights clarify the industry use cases and pave the way for innovative synthetic data applications. They promise to redefine industry standards and operational efficiencies across the board.
Success Stories: Impact of Synthetic Data in Various Industries
Synthetic data has revolutionized many sectors, with standout success stories in healthcare, finance, and manufacturing. These tales of triumph not only spark innovation but also showcase the tangible advantages of using synthetic data. They demonstrate how it can be a game-changer for real-world applications.
Healthcare Innovations with Synthetic Data
In healthcare, synthetic data has led to groundbreaking advancements. It has improved algorithm training and clinical trials, all while protecting patient privacy. For example, it enables the development of AI models for early disease detection and tailored treatments. This is a major leap forward in patient care and treatment precision.
By making data creation more affordable and efficient, synthetic data is opening doors to predictive and preventive healthcare. This is a significant step towards better health outcomes.
Synthetic Data Transforming Finance
The finance sector has also seen a major shift with synthetic data. It's helping to solve big problems like fraud detection, credit scoring, and risk management. Financial institutions can now build stronger predictive models, ensuring they meet regulatory standards and protect customer data.
This strategic use of synthetic data is key to improving decision-making and operational efficiency. It highlights the vital role synthetic data plays in the finance world.
Manufacturing Efficiency through Predictive Analysis
Synthetic data is making a big difference in manufacturing, driving improvements in predictive maintenance and production optimization. It allows companies to simulate production lines and test scenarios, predicting and preventing failures. This leads to less downtime and higher quality products.
The integration of synthetic data into manufacturing showcases its power to transform industry practices. It leads to more dynamic and cost-effective production environments.
Driving Forces Behind the Rise in Synthetic Data Usage
The digital world is rapidly changing, with synthetic data adoption driven by technological progress and regulatory changes. This shift is seen across various sectors, showing a strong compound annual growth rate (CAGR) of 33.1% for synthetic data generation from 2022 to 2028.
Technological Advancements Spurring Synthetic Data Growth
The integration of artificial intelligence (AI), machine learning (ML), and the Internet of Things (IoT) has transformed industries. These advancements make synthetic data essential for training advanced models. Companies like Mostly AI are leading this trend, retaining 99% of the original data's value while protecting sensitive information. This growth is expected to expand the market size from $381.3 million in 2022 to $2.1 billion by 2028.
Data Privacy and Regulatory Policies as Catalysts
Cyber security threats are on the rise, with 17% of the online population experiencing digital theft and 80% of cybercrimes unreported. Regulatory policies, such as GDPR in Europe and HIPAA in the US, are critical in synthetic data's growth. These laws enforce strict data privacy, pushing companies to use synthetic data as a secure, compliant alternative.
Advancements in privacy-focused AI models, like Large Language Models (LLMs) and Generative Adversarial Networks (GANs), highlight the importance of data protection. This shift is reflected in significant market adoptions, with North America holding a 35% market share in 2022.
Region | 2022 Market Size ($ million) | 2028 Projected Market Size ($ billion) | 2022-2028 CAGR |
---|---|---|---|
North America | 133.255 | 0.735 | 33.1% |
Europe | Rapid growth with strong data protection focus | 37.3% | |
Asia-Pacific | 67.05 | 0.92 | 35% |
Rest of World (RoW) | 47.995 | 0.41 | 60.02% |
The synthetic data market is expanding rapidly, playing a vital role in today's digital ecosystems. Technological advancements and regulatory drivers ensure synthetic data remains at the forefront of innovation. This drives its adoption across diverse industries worldwide.
Sector Trends: Who’s Adopting Synthetic Data and Why?
Synthetic data is transforming various industries, becoming a key driver of innovation. Its adoption is growing due to its unique benefits and applications. This article focuses on sectors embracing synthetic data, highlighting synthetic data applications and sector trends. These trends are reshaping competitive landscapes.
Automotive Industry’s Shift Towards Synthetic Data
The automotive sector is leveraging synthetic data to enhance autonomous vehicle development and safety. It simulates diverse driving scenarios, training AI systems more effectively. This approach avoids the high costs and risks of real-world data gathering.
Media and Entertainment Embracing Synthetic Data for Personalization
In media and entertainment, synthetic data personalizes content while protecting user privacy. It enables the creation of tailored viewing experiences, boosting engagement. This innovation ensures data security without compromising on personalization.
AI and Machine Learning's Reliance on Synthetic Data
AI and Machine Learning (ML) heavily rely on training data to enhance accuracy and functionality. Synthetic data offers a scalable, ethical solution. It generates data that mimics real-world scenarios, avoiding ethical and compliance risks.
The table below illustrates the Synthetic Data Software Market's growth from 2024 to 2031:
Year | Market Size (USD Million) | CAGR | Key Growth Driver |
---|---|---|---|
2024 | 319.4 | 35.28% | Data Privacy Solutions |
2028 | Approx 2,423.27 | AI & ML Innovations | |
2031 | 4,846.54 | Reduced Data Acquisition Costs |
The synthetic data sector is booming, essential for future advancements in various industries. These sector trends highlight the critical role of synthetic data applications in driving progress and innovation globally.
The Role of Synthetic Data in Navigating Data Privacy Concerns
The strategic deployment of synthetic data applications is transforming how companies tackle data privacy hurdles. As data governance demands grow, synthetic data emerges as a key solution. It meets strict privacy laws while allowing for extensive data use.
Industries like healthcare, finance, and marketing are leveraging synthetic data to tackle regulatory complexities. This method safeguards sensitive data and supports advanced analytics and machine learning. It does so without compromising individual privacy.
In healthcare, synthetic data boosts patient privacy, facilitating medical research and predictive model development. It ensures compliance with privacy laws. Financial institutions use it to refine risk models, protecting client data and maintaining confidentiality.
The adoption of synthetic data is reshaping data strategies, as highlighted by MindTech Global. It replicates real data properties while anonymizing sensitive info. This allows for innovation and data sharing within and outside organizations.
Looking ahead, synthetic data will be critical in upholding data privacy standards across sectors. It supports organizational flexibility and ensures data-driven innovations adhere to privacy laws.
Forecasting the Future: Market Projections and Synthetic Data Trends
The future of synthetic data generation is set for significant growth. Market analyses predict a substantial increase from USD 300 million in 2024 to USD 13.0 billion by 2034. This growth is expected at a Compound Annual Growth Rate (CAGR) of 45.9%. Such projections highlight the robust market projections tied to synthetic data trends.
Applications and Expected Innovations
The future of synthetic data is bright with emerging applications. Innovations in healthcare, finance, and public safety rely heavily on synthetic data trends. These trends improve dataset quality and ensure compliance with data privacy laws. Large Language Models (LLM), like GPT-3, are already showing promise in text generation and translation.
Real-time data synthesis is another advancing area. It enables organizations to create datasets that simulate current conditions. This is critical in areas like autonomous driving and real-time advertising bidding.
Cloud-based services will also benefit from synthetic data. As companies move to cloud environments, synthetic data enhances data management and scalability. This is essential for industries needing vast amounts of secure data for daily operations.
The future of synthetic data generation is not just promising; it's revolutionary. It will transform how industries operate, making data privacy, efficiency, and quality central to this field.
Challenges to Adoption: Addressing Skepticism and Technical Roadblocks
The adoption of synthetic data by industries holds great promise for innovation and privacy. Yet, significant adoption challenges persist. These include skepticism about data quality and realism, technical compatibility, and the need for substantial skill development for effective use.
Overcoming Quality and Realism Issues in Synthetic Data
Concerns about synthetic data's authenticity and applicability hinder its adoption. It must accurately reflect real-world scenarios to be truly valuable. This gap between ambition and capability is a major hurdle. For example, simulating user interactions in digital environments is challenging due to the complexity of human behavior.
Ensuring data diversity and unpredictability is a significant challenge. This leads to doubts about data reliability and the analytical insights it supports. To overcome these hurdles, refining data generation techniques is essential. Advanced machine learning models can enhance data realism. Rigorous testing against real-world scenarios also boosts trust among skeptics.
Documented case studies, like those in a recent report on AI in transportation, provide valuable examples of successful integrations. They demonstrate how to overcome associated challenges.
Skilling the Workforce for a Synthetic Data Future
The full realization of synthetic data's promise depends on the skill development of those who use it. The rapid pace of technological change demands continuous learning and adaptation. Professionals in data science and AI analytics must continually update their skills and understanding of ethical and regulatory issues.
Companies must invest in training programs and partnerships with educational institutions. This is necessary to build a skilled workforce. Upgrading current employees' skills is also critical, as they must navigate the complexities of modern data environments. This dual strategy can help address the pressing issue of a lack of qualified professionals.
Encouraging a culture of continuous learning and curiosity empowers teams to innovate with synthetic data. This fosters acceptance across industries and drives further progress.
In conclusion, while the journey to widespread synthetic data adoption is challenging, it is achievable. Improving data quality, realism, and workforce skills are key. Each step forward enhances synthetic data's functionality and broadens its acceptance. This paves the way for more innovative and privacy-respecting data applications in the future.
Summary
The surge in synthetic data adoption is reshaping industries. It's enabling more sophisticated AI training, deeper analytics, and more accurate risk simulations. Gartner predicts a significant leap, with 60% of AI training data being synthetic by the end of the year. This is a sharp increase from the 1% seen in earlier years.
Synthetic data addresses the issues of data scarcity, privacy, and unbalanced datasets. These are common problems with traditional data sets. It's a game-changer for industries facing these challenges.
Industry trends highlight synthetic data's strategic role in areas like anomaly detection and fraud prevention. In sectors where real-time data is critical, synthetic data is key. For example, in financial services, it's essential for quick decision-making.
FAQ
What is synthetic data?
Synthetic data is created using algorithms to mimic real-world data. It's used for training AI models, testing, and analysis. This approach ensures privacy and security.
How is synthetic data being adopted across different sectors?
Synthetic data is transforming various sectors. Healthcare uses it for research without compromising patient privacy. Finance leverages it for fraud detection and risk assessment. In manufacturing, it aids in predictive maintenance and quality improvement. Automotive and media also benefit from its use in training autonomous systems and personalizing experiences.
Can you provide examples of how synthetic data is transforming industries?
In healthcare, synthetic data enables research without risking patient privacy. Finance uses it for secure risk assessment models. Manufacturing benefits from predictive maintenance and quality enhancement through synthetic data.
What are the driving forces behind the increased use of synthetic data?
The rise of AI, ML, and IoT in business operations, along with strict data privacy laws, drives synthetic data adoption. Companies innovate while adhering to GDPR and HIPAA.
How do regulatory policies like GDPR impact the use of synthetic data?
GDPR and similar policies focus on data privacy, boosting synthetic data use. It allows companies to simulate and analyze data without privacy breaches.
What are the methods used to generate synthetic data?
Various methods generate synthetic data. These include Generative Adversarial Networks (GANs), Variational Autoencoders (VAEs), and Markov Chains. Recurrent Neural Networks (RNNs), Transformer Models, and ARIMA models are also used. Long Short-Term Memory (LSTM) Networks and Sequential GANs (SeqGANs) are among the tools employed.
What are the challenges in adopting synthetic data?
Adopting synthetic data faces challenges. Ensuring its quality and realism is key. Addressing skepticism and developing a skilled workforce are also hurdles.
What are the market size and growth projections for synthetic data?
The synthetic data generation market is valued at USD 218.4 million in 2023. It's expected to reach USD 2,339.8 million by 2030, growing at a CAGR of 31.1%.
What role does synthetic data play in AI and machine learning?
Synthetic data is essential for AI and machine learning. It's used when real-world data is scarce, impractical, or must remain private due to data protection laws.
Are there any case studies highlighting the successful application of synthetic data?
Yes, several case studies exist. Banking uses synthetic data for risk management. Retail personalizes shopping experiences without real customer data. Telecommunications benefit from network optimization and procedural efficiencies through synthetic data.
Comments ()