What You Need to Know: Data Annotation Job Requirements
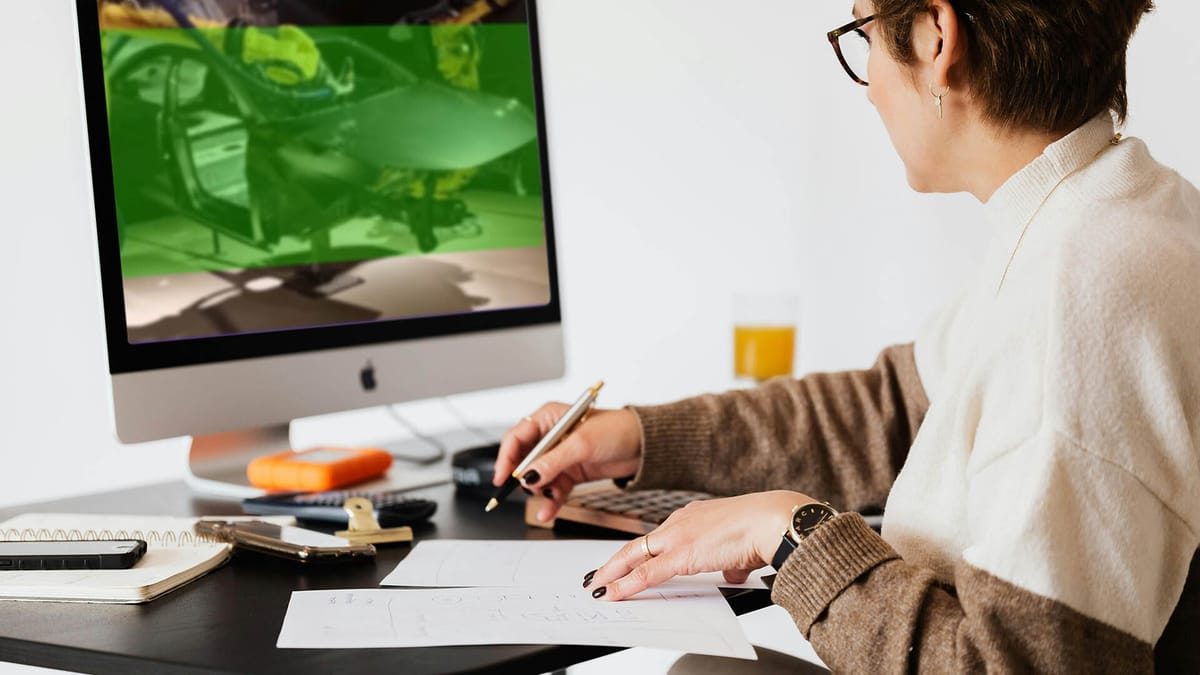
Data annotators can work with thousands of data points. They help train machine learning models which is key to the development of AI. This work includes tasks like image labeling, video marking, and transcription.
This job offers flexibility for those working freelance. It allows them to manage their own time. This can lead to a better balance between work and personal life.
But, being accurate and consistent in your work is very important. This helps you earn more, especially if you work freelance. Knowing the skills needed for data labeling is crucial. It helps you work more efficiently and meet deadlines.
Key Takeaways
- Data annotators label data points for training machine learning models, performing tasks like bounding boxes and transcription.
- Freelance data annotators enjoy flexibility in their schedules, managing their own productivity and deadlines.
- Accuracy and attention to detail are essential for success and higher compensation.
- Both technical skills such as SQL proficiency and soft skills like self-management are crucial in this role.
- The field offers various opportunities for personal and professional growth, with freelance and employed positions available.
Introduction to Data Annotation
Data annotation is vital for *improving machine learning* in recent years. It includes marking boundaries, identifying objects in pictures, and more. With an increase in unstructured data types, like social media posts and photos, this work is key.
Data annotators play a crucial role. They label data to help algorithms learn accurately. Image labeling, for example, is essential for self-driving cars and medical technology.
This data annotation job description explains the tasks required. Annotators must label text clearly and segment images well. Such labels help AI systems understand our language and improve user experiences.
There are different methods to annotate data, each with its own pros and cons:
- In-house Annotation: You get better quality control but might need more staff or training.
- Outsourced Annotation: Offers professional annotators but you sacrifice some control and there can be privacy issues.
- Crowdsourced Annotation: Offers scalability and cost-savings but it can be harder to ensure quality and privacy.
- AI-driven Annotation: Speeds up labeling big data sets but initial setup and ethical concerns might arise.
Annotation platforms such as Keylabs provide easy-to-use tools, expert advice, and strong security. They make managing data annotations easier, especially for big projects.
Key Skills Required for Data Annotation Jobs
Data annotation involves marking data points with great care. You need to have specific skills to do this work well. These include being very detail-oriented, good with computers, and able to manage your time. These skills help ensure accuracy and keep the workflow smooth and efficient.
Attention to Detail
Data annotators must pay very close attention to details. They label data with methods like drawing around objects in pictures, marking video frames, or writing down spoken words. Data annotator precision affects the quality of the labeled data. It also impacts how well the data helps machine learning models learn.
Computer Proficiency
You should be comfortable with computer tools and software. Data annotators use special platforms and software to label data. They need to be good with computers to use these tools well. Knowing SQL, programming like Python, and typing fast is important. This is especially true when working with big sets of data and making sure the data entered is correct.
Self-Management
Being able to manage yourself is key, especially for those who do data annotation as freelancers. Freelancers can choose their own working hours and places, like working from home. But they have to keep themselves on track. Without a boss or colleagues nearby, staying productive can be a challenge. Good self-management in data labeling means planning your work, setting achievable goals, and working somewhere quiet without distractions.
To be great at data annotation, you need to be detail-focused, good on a computer, and able to manage your time well. These skills are essential for delivering high-quality work. And this work is crucial for training machine learning models well.
Skill | Description | Importance |
---|---|---|
Attention to Detail | Ensuring precise and accurate data labeling | High |
Computer Proficiency | Using specialized software and tools effectively | High |
Self-Management | Managing time and tasks for productivity | High |
Understanding Different Types of Data Annotation
In the world of artificial intelligence, knowing about types of data annotation is key. It helps build better machine learning models. Specialists use different ways to mark data. This lets AI systems learn and decide. We will look at important types of data annotation in this field.
Bounding Boxes
Bounding boxes are an important type of data annotation. They draw rectangles around items in pictures. This helps models see and find these things. It's crucial for spotting objects in computer vision. This is useful in areas like self-driving cars and video cameras.
Video Marking
Video marking tasks need workers to note objects, scenes, or movements in each video frame. This process is key for teaching models quick identification. It's used in areas like self-driven systems and facial recognition. Sites like DataAnnotation.tech involve a big group who do this work.
Transcription and Translation
Transcription in data annotation changes spoken words from audio or video into text. It's vital for NLP setups. These include voice helpers and translation services. Such work also helps overcome language differences, making models better in a variety of languages.
Text Copying
Text copying moves text from different places into a machine-readable form. This helps NLP models work better. It ensures texts are the same and accurate. Doing tasks like text copying can make your annotation work more accurate and effective.
Freelance vs. Employed Data Annotators
Thinking of working in data annotation? Knowing the differences between freelance and employed work is key. Each offers its own pros and cons, depending on what you're looking for in a job.
Work Structure
Freelance annotators have a lot of freedom. They can pick what hours and which projects suit them best. This lets them balance work with their personal life more easily.
Employees have a tighter schedule, set by their bosses. This brings regular expectations and a steady routine. This kind of work tends to be more stable, as you don't have to look for projects.
Employee Benefits
Employed annotators get benefits like paid time off and health insurance.
Freelancers miss out on these perks but have more control. They handle their insurance, taxes, and retirement plans. For many, the flexibility is worth it.
Compensation Structure
Freelancers get paid for each piece of data they annotate. This system rewards accuracy and can lead to good daily pay. Depending on your geography, you can expect $10-$25 per hour as a beginner.
Employees, on the other hand, earn a regular salary. This predictability is comforting, with the chance for bonuses. However, freelancers might make more, depending on the work available.
Choosing between freelance or employed work comes down to personal preference. The data annotation field keeps growing, creating opportunities for all. It's all about what fits your goals and lifestyle best in the expanding world of AI data.
Data Annotation Job Requirements
If you're entering the data annotation field, you'll need a mix of tech skills and good people skills. It's super important to be good with computers and very detail-oriented. These skills ensure you label data accurately for training machine learning models well.
Having top-notch data labeling skills is key in this profession. You should be good at a wide range of tasks, like creating bounding boxes, marking videos, transcribing, and copying text. Doing these tasks well needs you to be both careful and consistent.
Success in data annotation also means you're good at managing yourself. You need to be on top of your time and work well to meet deadlines. This part of the job is vital for making good money freelancing or keeping a job's quality high.
Having knowledge in some specific industries can give you a leg up. For example, if you understand what waste management data needs, you can provide more accurate labels. Knowing how to use SQL, typing fast, and programming can help you do your work quicker and better.
If you want to do well in data annotation, you need to have the right data labeling qualifications.
How to Get Started in Data Annotation
If you're starting in data annotation, take some first steps. Your journey begins by looking at platforms that pay for tasks.
At these spots, you'll show how well you can tag data, like in images, text, or videos. Transparent pay in data labeling means you get fair money for your work. Some gigs pay about $20 an hour, but you might get more for unique tasks. Even with different pays and jobs, there's a lot of room to advance.
To do well in data annotation, you need computer smarts, a sharp eye for detail, and the ability to work independently and hit deadlines. This goes for both regular jobs and freelance work. Making these skills strong is key to success.
Job Vacancies and Employment Opportunities
Data annotation is key in AI's growth and creates many jobs. It's needed in many areas, leading to more jobs. People looking for part-time or full-time work can find jobs in freelancing or at companies.
Freelance Platforms
There are sites like Amazon Mechanical Turk that are great for freelancers. Over 100,000 freelancers use these sites for flexible, at-home work.
Corporate Opportunities
Working for a company might appeal to those who want regular hours and office settings. You have positions like ML Data Operations Lead to look forward to if you learn to manage other annotators and handle data for ML training beyond simple annotation.
Skilled annotators can also make $25 to $30 an hour. Plus, you might get health insurance and a retirement plan. This is for people who work full-time for these companies.
These jobs ask for advanced skills and sometimes need special certifications. Learning about things like natural language processing can help you get better pay.
Job Type | Potential Earnings | Benefits |
---|---|---|
Freelance Data Annotator | Up to $20/hr | Flexible Schedule |
Corporate Data Annotator | $25-$30/hr | Health Insurance, Retirement Plans |
ML Data Operations Lead | $117,000+ a year | Health Insurance, Retirement Plans |
Challenges in Data Annotation Work
Data annotation is crucial for creating AI models, but it faces many challenges. A key issue is the lack of skilled AI trainers. This makes it hard for companies to meet their needs. The work's repetitive nature and high mental demand lead to many people leaving these jobs. This turnover makes it tough to keep a skilled team.
There are problems with data quality and consistency. This is sometimes made worse by using crowdsourcing. Crowdsourcing can bring in a lot of data quickly. But, it might not always be of the best quality because the people annotating it might lack expertise.
Labeling large data sets accurately for AI models takes a lot of time. The challenge is to keep data annotation costs in check without sacrificing quality. Manual annotation is accurate but expensive. On the other hand, automated tools, while faster, might not be as precise. Finding a balance between these two approaches or using advanced tools can help.
Subjectivity in data annotation can cause problems, as different people may interpret images differently. This can lead to issues in model training. It shows how important it is to have strict quality control in place.
Let's look at the types of data annotation used in different industries:
- Image Labeling: It is used to categorize images by putting them into classes or groups using labels.
- Bounding Boxes: These are important for models detecting objects. They help mark the exact position of various objects in images.
- Segmentation:
- Semantic Segmentation: It labels each pixel in an image with a class, without separating objects of the same class.
- Instance Segmentation: This shows different objects of the same class as separate entities.
- Landmark Annotation: What this does is mark important points on objects in images. It’s great for tasks that need precise measurements or to recognize features.
- Polygon Annotation: It shows the shape or path of objects in images. This is crucial for models that need to understand shapes or navigate.
The financial side also poses challenges. The pay of data annotators varies widely. Sometimes, companies use pre-annotated data to save time. But this can mean compromising on the quality needed for advanced AI. Despite these issues, data annotation is key to AI advancement. It requires continual improvement and strategic solutions.
Summary
As a data annotator, you will do many tasks, like image annotation or text transcription. Each job needs certain abilities, showing why learning is important. Starting jobs for junior data annotators play a big role in teaching AI well, making it better for handling things like healthcare and self-driving cars.
FAQ
What are the qualifications needed for a data annotation job?
To be a data labeler, you need to be very sharp-eyed, good with computers, and able to handle your work well. It's helpful to know your way around data annotation tools.
What roles do data annotators play in advancing machine learning?
Data annotators are key in making machine learning models better. They carefully tag data like images and text. This makes sure the training data is spot-on.
What key skills are required for data annotation jobs?
For this job, you really need to pay close attention to every detail and love working on computers. Being able to manage your time and work quietly is also a big plus.
What are the different types of data annotation?
Data annotation has lots of types, from drawing boxes around stuff in pictures to transcribing text. You might also copy text into databases from different sources.
What is the difference between freelance and employed data annotators?
Freelance annotators make their own schedules and pick their projects. But they miss out on things like paid days off. Employed annotators work set hours and get benefits, depending on their jobs.
What are some data annotation tools and platforms available?
For data annotation, you can use tools like Amazon Mechanical Turk. They have tasks like labeling photos that can sometimes pay more for special skills.
Comments ()