Why Document Annotation is a Game-Changer in Text Processing
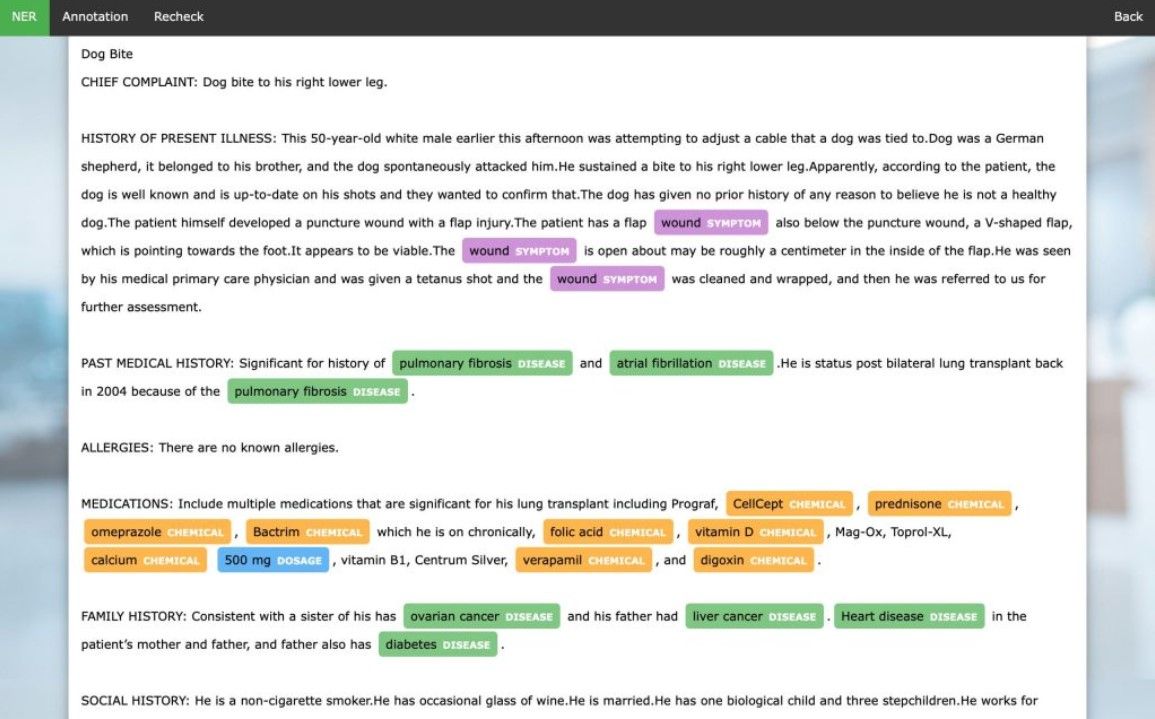
What is Document Annotation?
Document annotation means using a machine learning (ML) tool or AI technology to find and pull out specific details from a document based on certain rules. This way, instead of looking through a whole document, you can easily find the information you need. It also helps to arrange the information in a way that's easy to understand and share with others.
In the past, businesses hired people specifically for this job. But now, we see advancements in things like OCR (which helps computers read text from images or documents) and RPA (which automates routine tasks). Through this advancement, workers can focus on more important duties while machines take care of this task.
Document annotation forms the backbone of many AI-enabled document processing systems to help them in understanding, processing, and interacting with human language.
The scope of document annotation extends beyond text annotation. It includes various forms like value identification and document classification which are vital in the fields of natural language processing (NLP) and data annotation.
What are the types of document annotation and use cases?
1. Named Entity Annotation
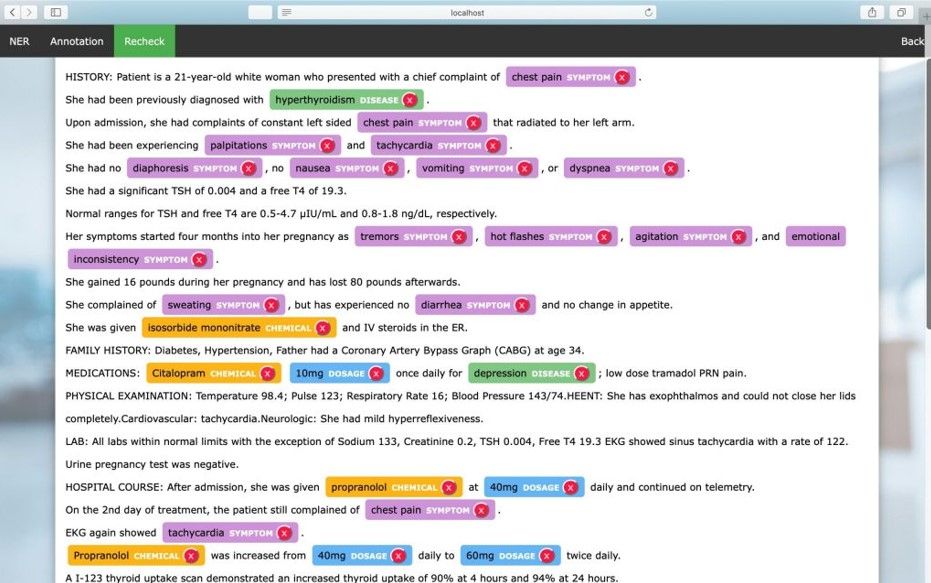
Named Entity Annotation focuses on identifying specific types of information in a text, such as names of people, organizations, locations, dates, and numerical values.
In the context of a document processing system, named entity annotation is essential for tasks like automatic data extraction, information retrieval, or question-answering systems.
For instance, in the healthcare sector, named entity annotation can help an AI-enabled document processing system identify and categorize specific terms within patient medical records, such as drug names, medical conditions, or procedures. This information is crucial for health monitoring, disease prediction, or designing personalized treatment plans.
2. Sentiment Annotation
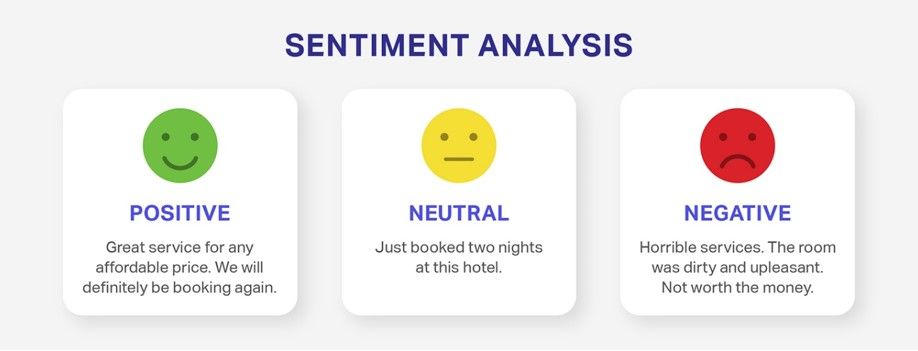
Sentiment annotation, an aspect of document annotation, classifies text based on the sentiment or emotion it conveys. This technique is vital in fields where subjective interpretation of text documents is crucial.
Consider a legal document processing system with legal training data powered by AI. The system can identify and understand the relationships between various legal terms and clauses in contracts using semantic document annotation. This helps ensure compliance by flagging potential issues and automating the review process. It saves time and reduces the margin for human error.
3. Semantic Document Annotation
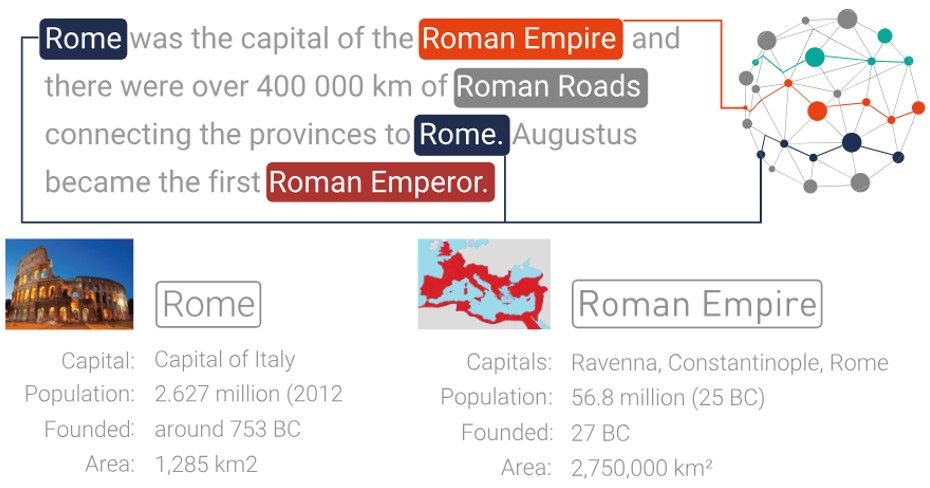
Semantic annotation, or tagging, is when you attach extra information to a text document or other unstructured content. This extra information, known as metadata, is about key concepts that are related to the document, like people, places, things, or topics.
The best thing about semantic annotations is that not only people, but machines can use them too. This makes documents with semantic tags easier to find, understand, mix together, and reuse.
The outcome of semantic annotation is metadata that describes the document by referring to concepts and things mentioned in the text or that are related to it. These references connect the content to formal descriptions of these concepts in a kind of knowledge network known as a knowledge graph.
Usually, this metadata is shown as a set of tags or annotations that add more information to the document, or specific parts of it, by identifying these concepts.
Organizations can leverage AI and machine learning to perform complex tasks with improved accuracy and efficiency using these types of document annotations. They assist in data extraction and value identification and enable a deeper understanding of the context, sentiment, and semantics hidden within the sea of unstructured data.
Importance of document annotation
Document annotation is instrumental in shaping the future of AI, machine learning, and natural language processing (NLP). It lays the groundwork for efficient data processing, understanding, and decision-making by converting unstructured data into structured formats. Here are some other reasons why document annotation is important.
1. Improve AI and Machine Learning Models
Document annotation helps create high-quality datasets essential for training robust AI and machine learning models. It enriches raw data by adding human insight and context, enhancing the learning process of these models. This leads to improved performance, including higher accuracy and efficiency.
2. Enhance Natural Language Understanding
In NLP, document annotation allows models to move beyond just understanding vocabulary and syntax. It captures the nuances of human language, such as sentiment, tone, and context. For instance, through sentiment annotation, AI systems can gauge the overall tone of a document. Similarly, semantic annotation allows systems to grasp complex relationships and abstract concepts within the text.
3. Facilitate Information Extraction
Document annotation streamlines the process of extracting relevant information from vast datasets. AI systems can tag and classify parts of a text to easily identify and retrieve specific pieces of information. This can range from extracting key details from legal documents to identifying essential patient data in healthcare records.
4. Enable Automation in Various Domains
Document annotation is not confined to a single industry or application. Its potential is being realized across sectors, from healthcare to finance, from HR to legal, and beyond. An AI-enabled document processing system with properly annotated data can automate tasks such as customer support, contract analysis, report generation, and more. It saves time, reduces costs, and improves overall productivity.
5. Drive Innovation
The foundational role of document annotation in AI and machine learning means it is a key driver of innovation. As annotation techniques become more sophisticated, so do the capabilities of AI systems. This paves the way for advancements like self-driving cars, advanced virtual assistants, and more.
Conclusion
Document annotation is an essential building block in AI, machine learning, and natural language processing. It enhances AI systems' understanding and processing capabilities, powering efficient information extraction and fostering automation across various domains.
As we continue to innovate and develop more advanced AI and ML applications, the importance and relevance of document annotation will undoubtedly keep growing.
Author: Vatsal Ghiya is a serial entrepreneur with more than 20 years of experience in healthcare AI software and services. He is the CEO and co-founder of Shaip, which enables the on-demand scaling of our platform, processes, and people for companies with the most demanding machine learning and artificial intelligence initiatives.
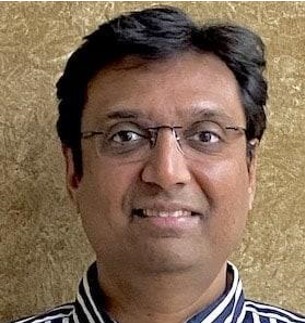
Comments ()